グラフ探索の構造
グラフ探索の構造では、各探索ステップの結果が調べられます。
グラフ探索の構造
単純な探索が複雑になる場合もありますが、通常は、再帰や分岐などの特殊なテクニックは採用されません。
グラフ探索チェーンを探索ステップに分割します。
g.V().hasLabel('recipe').count()
グラフ内のレシピ数を見つけるこのグラフ探索は、4つの部分で構成されます。- グラフ探索
g
g
は単独で実行される場合にエラーを返す- すべての頂点が
V()
で収集されます - すべての頂点が返されます。以下は結果の例です。
gremlin> g.V()==>v[{~label=recipe, recipeId=2003}] ==>v[{~label=recipe, recipeId=2005}] ==>v[{~label=ingredient, ingredId=3017}] ==>v[{~label=ingredient, ingredId=3028}] ==>v[{~label=person, personId=1}] ==>v[{~label=person, personId=3}] ==>v[{~label=book, bookId=1004}] ==>v[{~label=book, bookId=1001}] ==>v[{~label=meal, type="lunch", mealId=4003}]
- レシピとしてラベル付けされた頂点を
hasLabel('recipe')
で除外する - レシピである頂点のみが返されます。
gremlin> g.V().hasLabel('recipe') ==>v[{~label=recipe, recipeId=2003}] ==>v[{~label=recipe, recipeId=2005}] ==>v[{~label=recipe, recipeId=2006}] ==>v[{~label=recipe, recipeId=2007}] ==>v[{~label=recipe, recipeId=2001}] ==>v[{~label=recipe, recipeId=2002}] ==>v[{~label=recipe, recipeId=2004}] ==>v[{~label=recipe, recipeId=2008}]
- 頂点の数を
count()
でカウントする - 前回の探索ステップから返された頂点の数が合計されます。
gremlin> g.V().hasLabel('recipe').count() ==>8
エッジを使用したグラフ探索
以下に示す探索を試行する前に、Studio(コピー&ペースト)またはGremlin Console(:load /tmp/generateReviews.groovy)で次のスクリプトを実行します。
// reviewer vertices
johnDoe = graph.addVertex(label, 'person', 'personId', 11, 'name','John Doe')
johnSmith = graph.addVertex(label, 'person', 'personId', 12, 'name', 'John Smith')
janeDoe = graph.addVertex(label, 'person', 'personId', 13, 'name','Jane Doe')
sharonSmith = graph.addVertex(label, 'person', 'personId', 14, 'name','Sharon Smith')
betsyJones = graph.addVertex(label, 'person', 'personId', 15, 'name','Betsy Jones')
beefBourguignon = g.V().has('recipe', 'recipeId', 2001, 'name','Beef Bourguignon').tryNext().orElseGet {graph.addVertex(label, 'recipe', 'recipeId', 2001, 'name', 'Beef Bourguignon')}
spicyMeatLoaf = g.V().has('recipe', 'recipeId', 2005, 'name','Spicy Meatloaf').tryNext().orElseGet {graph.addVertex(label, 'recipe', 'recipeId', 2005, 'name', 'Spicy Meatloaf')}
carrotSoup = g.V().has('recipe', 'recipeId', 2007, 'name','Carrot Soup').tryNext().orElseGet {graph.addVertex(label, 'recipe', 'recipedId', 2007, 'name', 'Carrot Soup')}
// reviewer - recipe edges
johnDoe.addEdge('reviewed', beefBourguignon, 'timestamp', Instant.parse('2014-01-01T00:00:00.00Z'), 'stars', 5, 'comment', 'Pretty tasty!')
johnSmith.addEdge('reviewed', beefBourguignon, 'timestamp', Instant.parse('2014-01-23T00:00:00.00Z'), 'stars', 4)
janeDoe.addEdge('reviewed', beefBourguignon, 'timestamp', Instant.parse('2014-02-01T00:00:00.00Z'), 'stars', 5, 'comment', 'Yummy!')
sharonSmith.addEdge('reviewed', beefBourguignon, 'timestamp', Instant.parse('2015-01-01T00:00:00.00Z'), 'stars', 3, 'comment', 'It was okay.')
johnDoe.addEdge('reviewed', spicyMeatLoaf, 'timestamp', Instant.parse('2015-12-31T00:00:00.00Z'), 'stars', 4, 'comment', 'Really spicy - be careful!')
sharonSmith.addEdge('reviewed', spicyMeatLoaf, 'timestamp',Instant.parse('2014-07-23T00:00:00.00Z'), 'stars', 3, 'comment', 'Too spicy for me. Use less garlic.')
janeDoe.addEdge('reviewed', carrotSoup, 'timestamp', Instant.parse('2015-12-30T00:00:00.00Z'), 'stars', 5, 'comment', 'Loved this soup! Yummy vegetarian!')
グラフ・データのフィルター処理と変換を必要に応じて行って、任意の数の探索ステップをつなげて1つの探索にまとめることができます。場合によっては、予想外にエッジが結果として生成されることがあります。次の探索があるとします。
g.V().hasLabel('recipe').has('name', 'Beef Bourguignon').inE().values('comment')
このグラフ探索は、前回の探索と同様にg.V().hasLabel('recipe')
で始まります。その後に以下が続きます。- 指定されたレシピ・タイトルを持つ頂点のみを選択するための探索ステップ
- レシピ・タイトルが一意の場合は、このフィルターによって単一レシピが捕捉されます。
gremlin> g.V().hasLabel('recipe').has('name', 'Beef Bourguignon') ==>v[{~label=recipe, recipeId=2001}]
- 内向きエッジを取得する探索ステップ
- 完全な結果から標本抽出された2つのエッジからわかるとおり、ラベルを持つエッジはこのステップでフィルターされます。ターゲットの結果がレーティングのみの場合は、
inE('reviewed')
を使用すると精度が向上します。gremlin> g.V().hasLabel('recipe').has('name', 'Beef Bourguignon').inE() ==>e[{~label=includedIn, ~out_vertex={~label=ingredient, ingredId=3001}, ~in_vertex={~label=recipe, recipeId=2001}, ~local_id=95672dd0-2e3d-11e8-8043-6bfe97ac83b9}] [{~label=ingredient, ingredId=3001}-includedIn->{~label=recipe, recipeId=2001}] ==>e[{~label=includedIn, ~out_vertex={~label=ingredient, ingredId=3002}, ~in_vertex={~label=recipe, recipeId=2001}, ~local_id=9567ca17-2e3d-11e8-8043-6bfe97ac83b9}] [{~label=ingredient, ingredId=3002}-includedIn->{~label=recipe, recipeId=2001}] ==>e[{~label=includedIn, ~out_vertex={~label=ingredient, ingredId=3003}, ~in_vertex={~label=recipe, recipeId=2001}, ~local_id=9567ca16-2e3d-11e8-8043-6bfe97ac83b9}] [{~label=ingredient, ingredId=3003}-includedIn->{~label=recipe, recipeId=2001}] ==>e[{~label=includedIn, ~out_vertex={~label=ingredient, ingredId=3004}, ~in_vertex={~label=recipe, recipeId=2001}, ~local_id=9567ca14-2e3d-11e8-8043-6bfe97ac83b9}] [{~label=ingredient, ingredId=3004}-includedIn->{~label=recipe, recipeId=2001}] ==>e[{~label=includedIn, ~out_vertex={~label=ingredient, ingredId=3005}, ~in_vertex={~label=recipe, recipeId=2001}, ~local_id=956754e0-2e3d-11e8-8043-6bfe97ac83b9}] [{~label=ingredient, ingredId=3005}-includedIn->{~label=recipe, recipeId=2001}] ==>e[{~label=created, ~out_vertex={~label=person, personId=1}, ~in_vertex={~label=recipe, recipeId=2001}, ~local_id=956495c4-2e3d-11e8-8043-6bfe97ac83b9}] [{~label=person, personId=1}-created->{~label=recipe, recipeId=2001}] ==>e[{~label=reviewed, ~out_vertex={~label=person, personId=11}, ~in_vertex={~label=recipe, recipeId=2001}, ~local_id=95775a70-2e3d-11e8-8043-6bfe97ac83b9}] [{~label=person, personId=11}-reviewed->{~label=recipe, recipeId=2001}] ==>e[{~label=reviewed, ~out_vertex={~label=person, personId=12}, ~in_vertex={~label=recipe, recipeId=2001}, ~local_id=95773361-2e3d-11e8-8043-6bfe97ac83b9}] [{~label=person, personId=12}-reviewed->{~label=recipe, recipeId=2001}] ==>e[{~label=reviewed, ~out_vertex={~label=person, personId=13}, ~in_vertex={~label=recipe, recipeId=2001}, ~local_id=95773360-2e3d-11e8-8043-6bfe97ac83b9}] [{~label=person, personId=13}-reviewed->{~label=recipe, recipeId=2001}] ==>e[{~label=reviewed, ~out_vertex={~label=person, personId=14}, ~in_vertex={~label=recipe, recipeId=2001}, ~local_id=95778180-2e3d-11e8-8043-6bfe97ac83b9}] [{~label=person, personId=14}-reviewed->{~label=recipe, recipeId=2001}]
- ratedエッジからのcommentプロパティの解析
- ここでは、
inE()
がエッジ・ラベルreviewed
を使用して指定されています。プロパティ値はプロパティ・キーcomment
について取得されます。gremlin> g.V().hasLabel('recipe').has('name', 'Beef Bourguignon').inE('reviewed').values('comment') ==>Yummy! ==>Pretty tasty! ==>It was okay.
グラフ探索を一度に1ステップずつ構築すると、興味深い結果が生成され、探索の作成方法に関するインサイトが得られる場合があります。
グラフ探索のパス
グラフ探索のパスを示す探索ステップがあります。まず、料理本とレシピのタイトルがわかっている場合に、「牛肉とニンジンを材料とするどのようなレシピが料理本に含まれているか」という質問に答える探索の結果を見つけます。
gremlin> g.V().hasLabel('ingredient').has('name',within('beef','carrots')).in().as('Recipe').
out().hasLabel('book').as('Book').
select('Book','Recipe').by('name').
by('name')
==>{Book=The Art of French Cooking, Vol. 1, Recipe=Beef Bourguignon}
==>{Book=The Art of Simple Food: Notes, Lessons, and Recipes from a Delicious Revolution, Recipe=Carrot Soup}
ingredient
からrecipe
、book
に至るまでの探索パスが考えられます。この想定が正しいかどうかを確認するには、探索の最後にpath()
を追加します。gremlin> g.V().hasLabel('ingredient').has('name',within('beef','carrots')).
in().as('Recipe').
out().hasLabel('book').as('Book').
select('Book','Recipe').
by('name').by('name').path()
==>[v[{~label=ingredient, ingredId=3001}], v[{~label=recipe, recipeId=2001}], v[{~label=book, bookId=1001}],
{Book=The Art of French Cooking, Vol. 1, Recipe=Beef Bourguignon}]
==>[v[{~label=ingredient, ingredId=3028}], v[{~label=recipe, recipeId=2007}], v[{~label=book, bookId=1004}],
{Book=The Art of Simple Food: Notes, Lessons, and Recipes from a Delicious Revolution, Recipe=Carrot Soup}]
想定されたパスを探索がたどっていることがわかります。探索メトリック
メトリクスは、各グラフ探索ステップの出力をトレースするだけでなく、興味深いインサイトも生成できます。直前に示した探索にメトリクスを追加するには、連続した追加のステップを使用します。
gremlin> g.V().has('recipe', 'name', 'Beef Bourguignon').inE().values('comment').profile()
==>Traversal Metrics
Step Count Traversers Time (ms) % Dur
=============================================================================================================
DsegGraphStep(vertex,[],(label = recipe & name ... 1 1 9.710 77.19
query-optimizer 0.946
\_condition=((label = recipe & name = Beef Bourguignon) & (true))
query-setup 0.051
\_isFitted=true
\_isSorted=false
\_isScan=false
index-query 7.131
\_indexType=Search
\_usesCache=false
\_statement=SELECT "recipeId" FROM "newComp"."recipe_p" WHERE "solr_query" = '{"q":"*:*", "fq":["name:Bee
f\\ Bourguignon"]}' LIMIT ?; with params (java.lang.Integer) 50000
\_options=Options{consistency=Optional[ONE], serialConsistency=Optional.empty, fallbackConsistency=Option
al.empty, pagingState=null, pageSize=-1, user=Optional.empty, waitForSchemaAgreement=true, asyn
c=true}
DsegVertexStep(IN,edge,direction = IN,Unordered) 10 10 2.275 18.09
query-optimizer 0.421
\_condition=((true) & direction = IN)
query-setup 0.016
\_isFitted=true
\_isSorted=false
\_isScan=false
vertex-query 0.475
\_usesCache=false
\_statement=SELECT * FROM "newComp"."recipe_e" WHERE "recipeId" = ? LIMIT ? ALLOW FILTERING; with params
(java.lang.Integer) 2001, (java.lang.Integer) 50000
\_options=Options{consistency=Optional[ONE], serialConsistency=Optional.empty, fallbackConsistency=Option
al.empty, pagingState=null, pageSize=-1, user=Optional.empty, waitForSchemaAgreement=true, asyn
c=true}
\_usesIndex=false
DsegPropertiesStep([comment],value,label = comm... 3 3 0.593 4.71
>TOTAL - - 12.579 -
探索ステップのタイプが、探索の数と探索ステップの所要時間とともに表示されます。探索ステップを並列処理できる場合は、データの取得に複数の探索ツールが使用されます。探索ステップの中には、グラフ全体からの取得を必要とするグラフ・グローバルなものがあります。たとえば、DsegGraphStepは、特定の条件と合致する頂点を見つけるグラフ・グローバルな取得ステップです。一方、グラフ・ローカルな移動である探索ステップは並列処理できます。たとえば、DsegVertexStepは、制約パスに沿ってグラフ内を移動するグラフ・ローカル移動です。DSE Graphは、自動クエリー最適化を使用して、既存のインデックス構造を効率的に使用するための探索ストラテジを決定します。メトリクスを見ていると、パフォーマンスに関する質問が頭に浮かびます。たとえば、前述の探索を最適化する方法はあるでしょうか?実際、次のような単純な修正を加えることで、時間の節約に結び付きます。
gremlin> g.V().has('recipe', 'name', 'Beef Bourguignon').inE('reviewed').values('comment').profile()
==>Traversal Metrics
Step Count Traversers Time (ms) % Dur
=============================================================================================================
DsegGraphStep(vertex,[],(label = recipe & name ... 1 1 7.109 83.34
query-optimizer 0.342
\_condition=((label = recipe & name = Beef Bourguignon) & (true))
query-setup 0.013
\_isFitted=true
\_isSorted=false
\_isScan=false
index-query 6.110
\_indexType=Search
\_usesCache=false
\_statement=SELECT "recipeId" FROM "DSE_GRAPH_QUICKSTART"."recipe_p" WHERE "solr_query" = '{"q":"*:*", "fq":["name:Bee
f\\ Bourguignon"]}' LIMIT ?; with params (java.lang.Integer) 50000
\_options=Options{consistency=Optional[ONE], serialConsistency=Optional.empty, fallbackConsistency=Option
al.empty, pagingState=null, pageSize=-1, user=Optional.empty, waitForSchemaAgreement=true, asyn
c=true}
DsegVertexStep(IN,[reviewed],edge,(direction = ... 4 4 1.234 14.47
query-optimizer 0.187
\_condition=((direction = IN & label = reviewed) & (true))
query-setup 0.009
\_isFitted=true
\_isSorted=false
\_isScan=false
vertex-query 0.474
\_usesCache=false
\_statement=SELECT * FROM "DSE_GRAPH_QUICKSTART"."recipe_e" WHERE "recipeId" = ? AND "~~edge_label_id" = ? LIMIT ? ALL
OW FILTERING; with params (java.lang.Integer) 2001, (java.lang.Integer) 65633, (java.lang.Int
eger) 50000
\_options=Options{consistency=Optional[ONE], serialConsistency=Optional.empty, fallbackConsistency=Option
al.empty, pagingState=null, pageSize=-1, user=Optional.empty, waitForSchemaAgreement=true, asyn
c=true}
\_usesIndex=false
DsegPropertiesStep([comment],value,label = comm... 3 3 0.186 2.19
>TOTAL - - 8.530 -
変更はごくわずかです。レビューであるエッジのみを検索するために、走査ステップinE()
はinE('reviewed')
に置き換えられました。測定結果はそれぞれ異なる場合がありますが、通常は、2番目の探索のパフォーマンスが最初の探索を上回ります。DataStax Studioのメトリクスは、探索の任意のフェーズをスクロールまたは選択できて、使いやすくなっています。
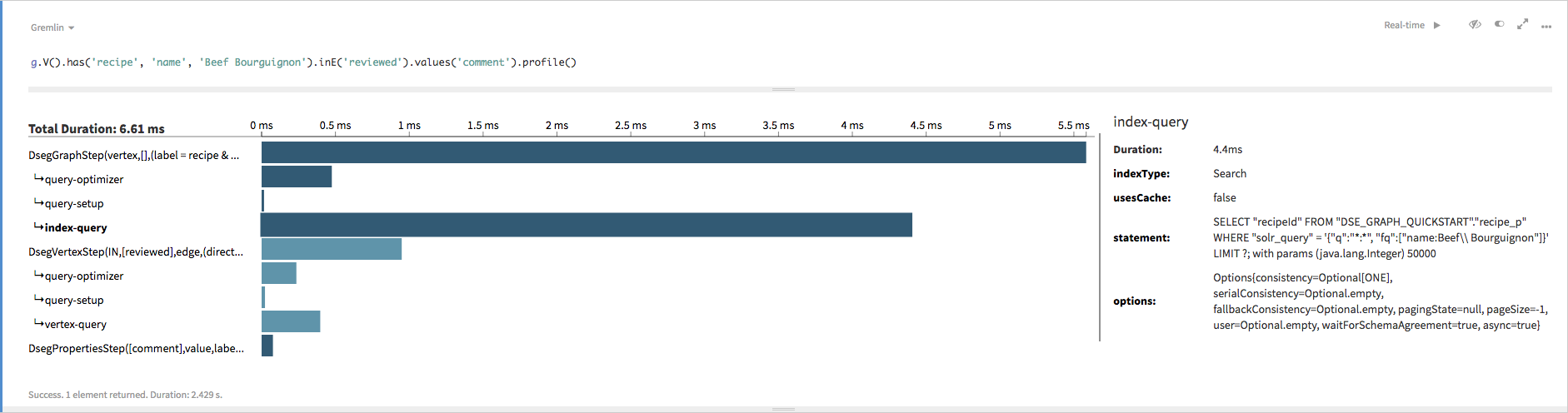
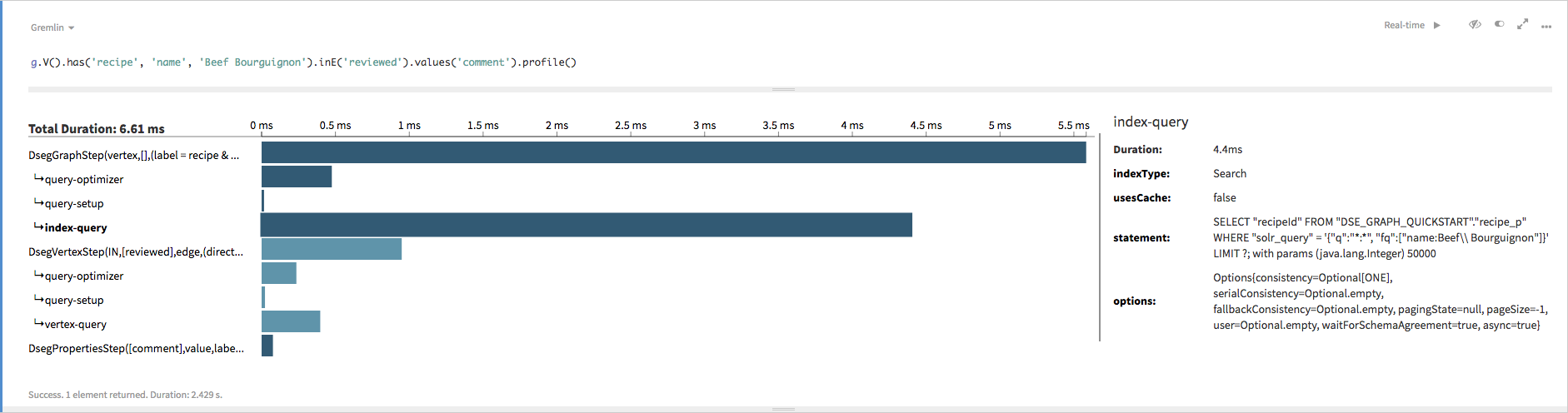