Batching inserts, updates, and deletes
Batch operations for both single partition and multiple partitions ensure atomicity. An atomic transaction is an indivisible and irreducible series of operations that are either all executed, or none are executed. Single partition batch operations are atomic automatically, while multiple partition batch operations require the use of a batchlog to ensure atomicity.
Use batching if atomicity is a primary concern for a group of operations. Single partition batch operations are processed on the server side as a single mutation for improved performance, provided the number of operations do not exceed the maximum size of a single operation or cause the query to time out.
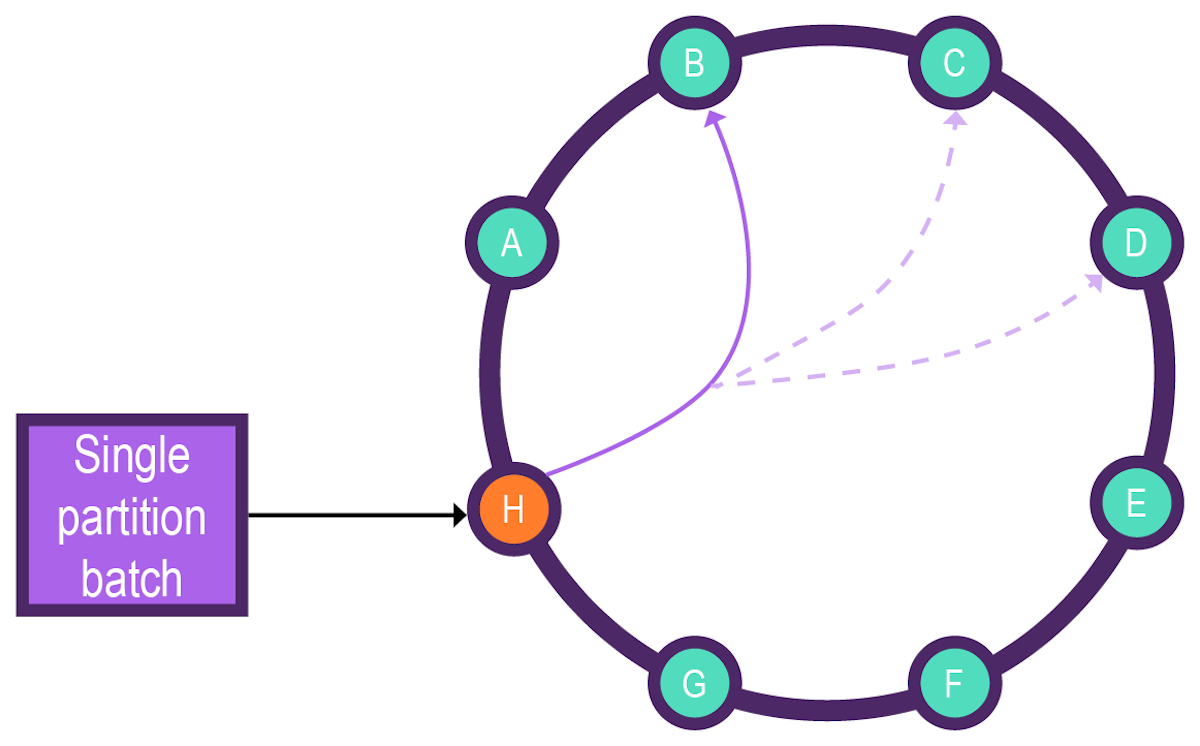
Multiple partition batch operations often have performance issues and should be used only if atomicity must be ensured.
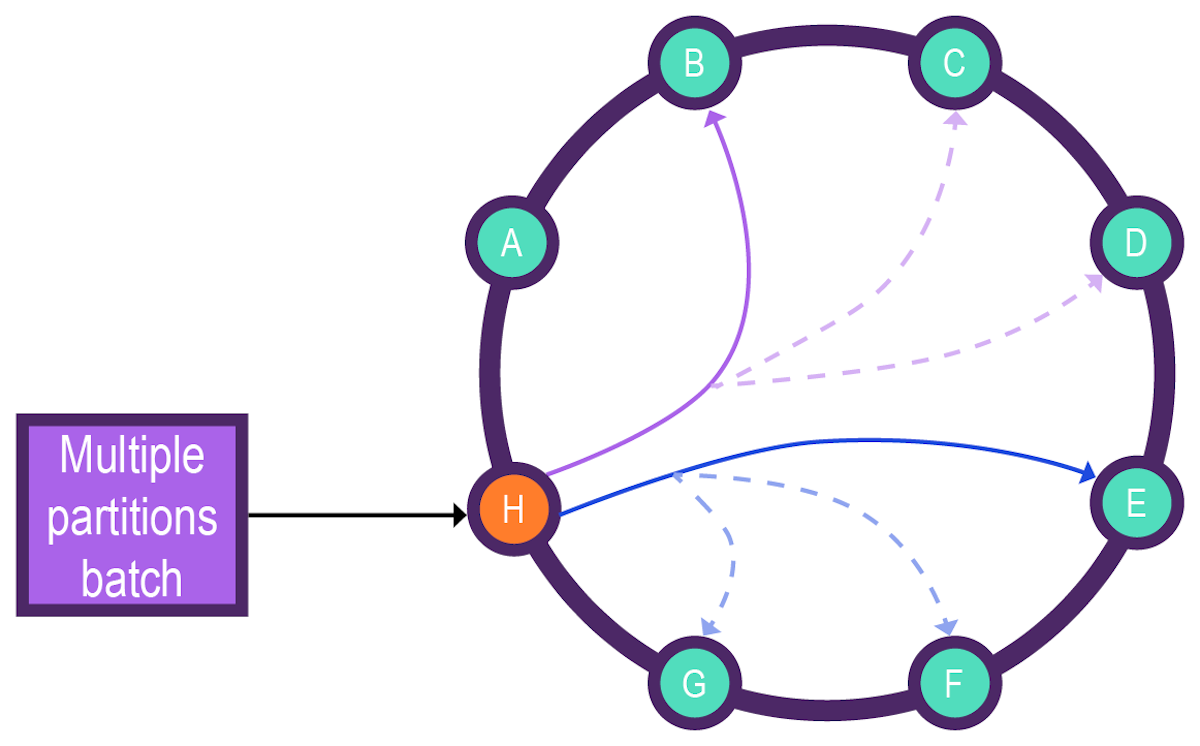
A cassandra.yaml
option lets you choose the batchlog_endpoint_strategy.
Batching can be effective for single partition write operations. But batches are often mistakenly used in an attempt to optimize performance. Depending on the batch operation, the performance may actually worsen. Some batch operations place a greater burden on the coordinator node and lessen the efficiency of the data insertion.
Client sends a batch request to the coordinator node, which sends two copies of the batchlog to the batchlog nodes. The batchlog nodes confirm receipt of the batchlog.
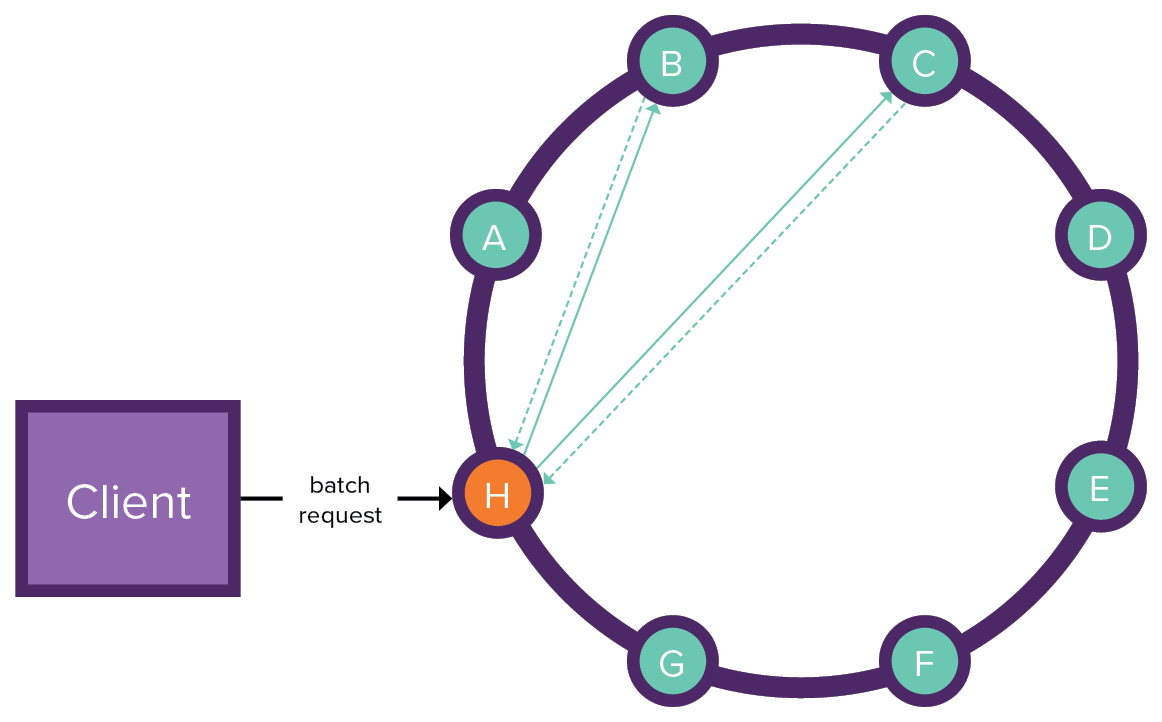
The coordinator node executes the batch statements on the nodes. The receiving nodes return the execution results to the coordinator node. The coordinator node removes the batchlog from the batchlog nodes and sends confirmation to the client.
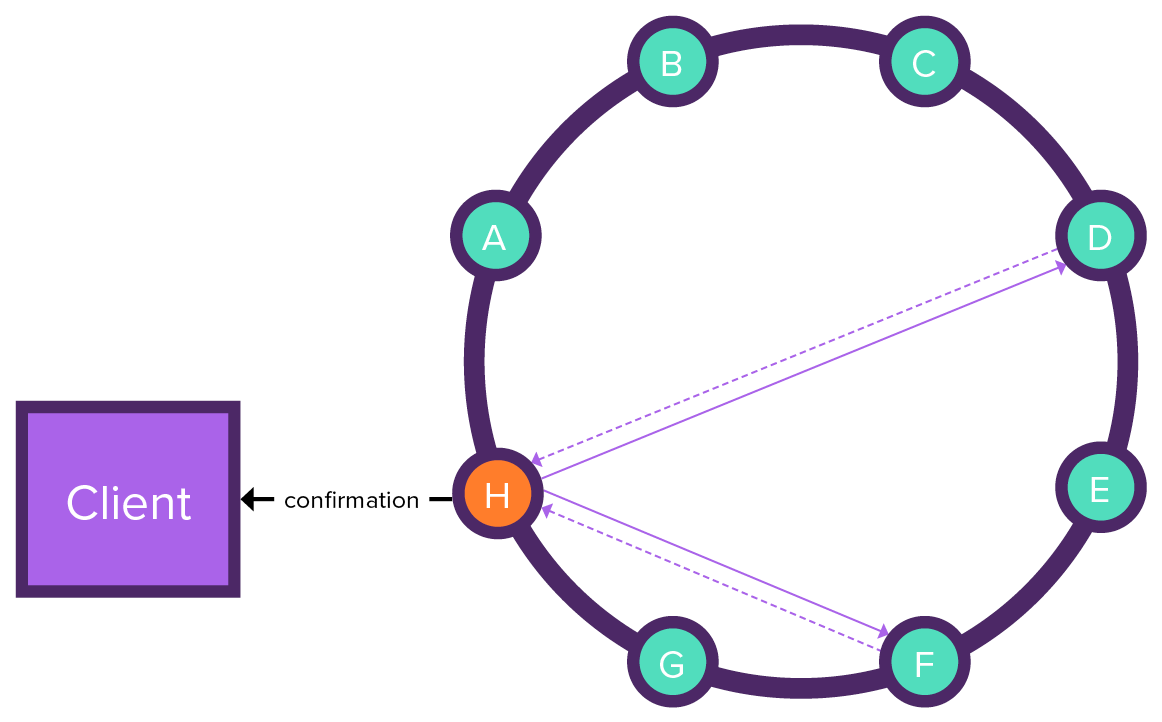
When coordinator node fails during batch execution, the batchlog node tries to execute the batch and the coordinator node sends a batch write timeout to the client. The batchlog nodes continue to try to execute statements until results are received from each receiving node. Once all of the results are received, the batchlog is removed from the batchlog nodes.
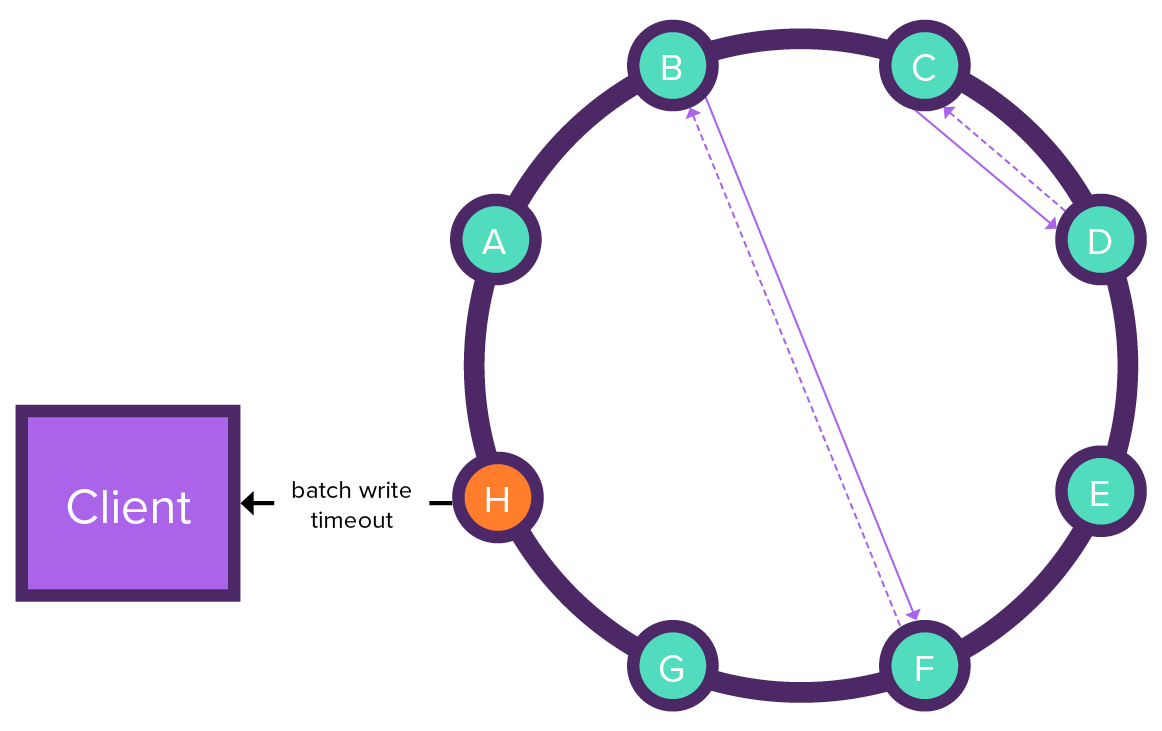
The number of partitions involved in a batch operation, and therefore the potential for multi-node access, can significantly increase latency. In all batching, the coordinator node manages all write operations, so that the coordinator node can be a bottleneck.
Good reasons for batching operations:
-
Inserts, updates, or deletes to a single partition when atomicity and isolation is a requirement. Atomicity ensures that either all or nothing is written. Isolation ensures that partial insertion or updates are not accessed until all operations are complete.
Single partition batching sends one message to the coordinator for all operations. All replicas for the single partition receive the data, and the coordinator waits for acknowledgement. No batchlog mechanism is necessary. The number of nodes involved in the batch is bounded by the number of replicas.
-
Ensuring atomicity for small inserts or updates to multiple partitions when data inconsistency must not occur.
Multiple partition batching sends one message to the coordinator for all operations. The coordinator writes a batchlog that is replicated to other nodes to ensure that inconsistency will not occur if the coordinator fails. Then the coordinator must wait for all nodes with an affected partition to acknowledge the operations before removing the logged batch. The number of nodes involved in the batch is bounded by number of distinct partition keys in the logged batch plus (possibly) the batchlog replica nodes. While a batch operation for a small number of partitions may be critical for consistency, this use case is more the exception than the rule.
Poor reasons for batching operations:
-
Inserting or updating data to multiple partitions, especially when a large number of partitions are involved.
As previously stated, batching to multiple partitions has performance costs. Unlogged batch operations are possible, to avoid the additional time cost of the batchlog, but the coordinator node will be a bottleneck because of synchronization. A better alternative uses asynchronous writes using driver code; the token aware loading balancing will distribute the writes to several coordinator nodes, decreasing the time to complete the insert and update operations.
Batched statements can save network round-trips between the client and the server, and possibly between the coordinator and the replicas. However, consider if batch operations are truly necessary. For information about the fastest way to load data, see "Batch loading without the Batch keyword."