Helpers
This Langflow feature is currently in public preview. Development is ongoing, and the features and functionality are subject to change. Langflow, and the use of such, is subject to the DataStax Preview Terms. |
Helper components provide utility functions to help manage data, tasks, and other components in your flow.
Use a helper component in a flow
Chat memory in Langflow is stored either in local Langflow tables with LCBufferMemory
, or connected to an external database.
The Store Message helper component stores chat memories as Data
objects, and the Message History helper component retrieves chat messages as data objects or strings.
This example flow stores and retrieves chat history from an Astra DB Chat Memory component with Store Message and Chat Memory components.
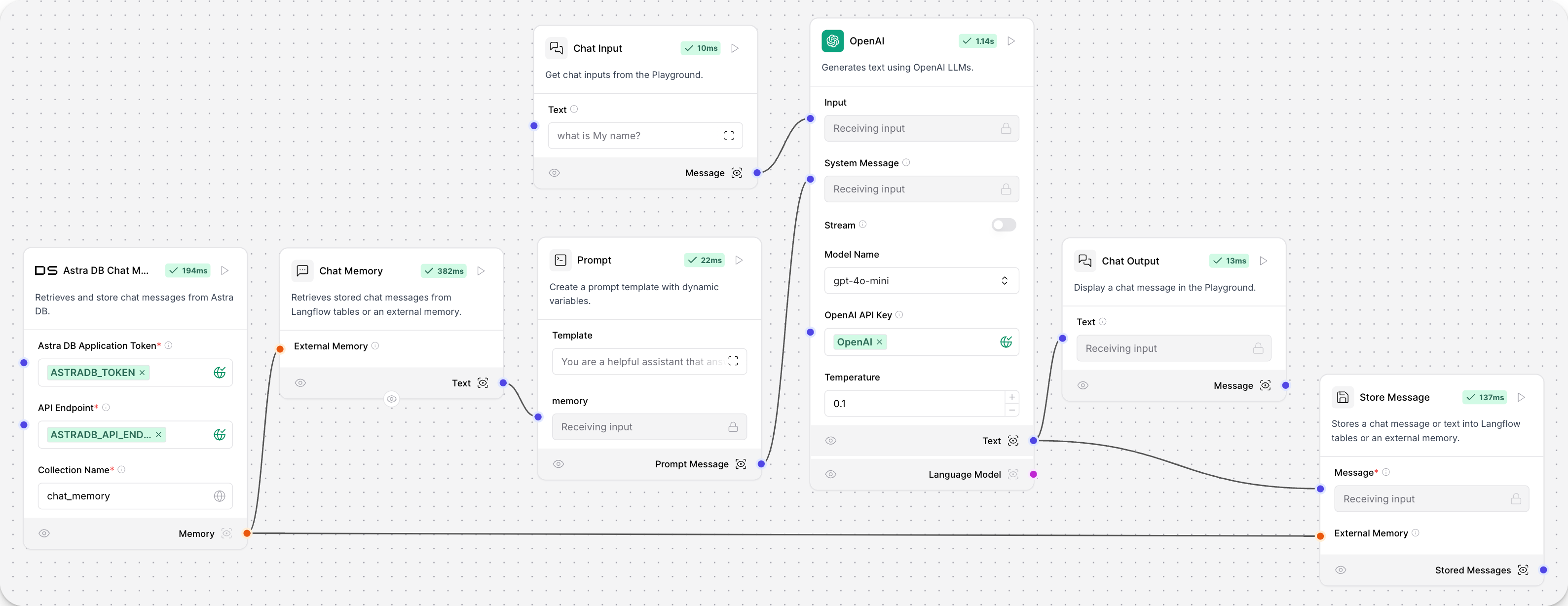
Batch run
This component is in Beta as of Langflow version 1.1.3, and is not yet fully supported. |
The batch run component runs a language model over each row of a DataFrame text column, and returns a new DataFrame with the original text and the model’s response.
Parameters
Name | Display Name | Info |
---|---|---|
model |
Language Model |
Connect the 'Language Model' output from your LLM component here. |
system_message |
System Message |
Multi-line system instruction for all rows in the DataFrame. |
df |
DataFrame |
The DataFrame whose column, specified by 'column_name', will be treated as text messages. |
column_name |
Column Name |
The name of the DataFrame column to treat as text messages. |
Name | Display Name | Info |
---|---|---|
batch_results |
Batch Results |
A DataFrame with two columns: 'text_input' and 'model_response'. |
Component code
batch_run.py
from __future__ import annotations
import operator
from typing import TYPE_CHECKING, Any
from loguru import logger
from langflow.custom import Component
from langflow.io import (
BoolInput,
DataFrameInput,
HandleInput,
MessageTextInput,
MultilineInput,
Output,
)
from langflow.schema import DataFrame
if TYPE_CHECKING:
from langchain_core.runnables import Runnable
class BatchRunComponent(Component):
display_name = "Batch Run"
description = (
"Runs a language model over each row of a DataFrame's text column and returns a new "
"DataFrame with three columns: '**text_input**' (the original text), "
"'**model_response**' (the model's response),and '**batch_index**' (the processing order)."
)
icon = "List"
beta = True
inputs = [
HandleInput(
name="model",
display_name="Language Model",
info="Connect the 'Language Model' output from your LLM component here.",
input_types=["LanguageModel"],
required=True,
),
MultilineInput(
name="system_message",
display_name="System Message",
info="Multi-line system instruction for all rows in the DataFrame.",
required=False,
),
DataFrameInput(
name="df",
display_name="DataFrame",
info="The DataFrame whose column (specified by 'column_name') we'll treat as text messages.",
required=True,
),
MessageTextInput(
name="column_name",
display_name="Column Name",
info="The name of the DataFrame column to treat as text messages. Default='text'.",
value="text",
required=True,
advanced=True,
),
BoolInput(
name="enable_metadata",
display_name="Enable Metadata",
info="If True, add metadata to the output DataFrame.",
value=True,
required=False,
advanced=True,
),
]
outputs = [
Output(
display_name="Batch Results",
name="batch_results",
method="run_batch",
info="A DataFrame with columns: 'text_input', 'model_response', 'batch_index', and 'metadata'.",
),
]
def _create_base_row(self, text_input: str = "", model_response: str = "", batch_index: int = -1) -> dict[str, Any]:
"""Create a base row with optional metadata."""
return {
"text_input": text_input,
"model_response": model_response,
"batch_index": batch_index,
}
def _add_metadata(
self, row: dict[str, Any], *, success: bool = True, system_msg: str = "", error: str | None = None
) -> None:
"""Add metadata to a row if enabled."""
if not self.enable_metadata:
return
if success:
row["metadata"] = {
"has_system_message": bool(system_msg),
"input_length": len(row["text_input"]),
"response_length": len(row["model_response"]),
"processing_status": "success",
}
else:
row["metadata"] = {
"error": error,
"processing_status": "failed",
}
async def run_batch(self) -> DataFrame:
"""Process each row in df[column_name] with the language model asynchronously.
Returns:
DataFrame: A new DataFrame containing:
- text_input: The original input text
- model_response: The model's response
- batch_index: The processing order
- metadata: Additional processing information
Raises:
ValueError: If the specified column is not found in the DataFrame
TypeError: If the model is not compatible or input types are wrong
"""
model: Runnable = self.model
system_msg = self.system_message or ""
df: DataFrame = self.df
col_name = self.column_name or "text"
# Validate inputs first
if not isinstance(df, DataFrame):
msg = f"Expected DataFrame input, got {type(df)}"
raise TypeError(msg)
if col_name not in df.columns:
msg = f"Column '{col_name}' not found in the DataFrame. Available columns: {', '.join(df.columns)}"
raise ValueError(msg)
try:
# Convert the specified column to a list of strings
user_texts = df[col_name].astype(str).tolist()
total_rows = len(user_texts)
logger.info(f"Processing {total_rows} rows with batch run")
# Prepare the batch of conversations
conversations = [
[{"role": "system", "content": system_msg}, {"role": "user", "content": text}]
if system_msg
else [{"role": "user", "content": text}]
for text in user_texts
]
# Configure the model with project info and callbacks
model = model.with_config(
{
"run_name": self.display_name,
"project_name": self.get_project_name(),
"callbacks": self.get_langchain_callbacks(),
}
)
# Process batches and track progress
responses_with_idx = [
(idx, response)
for idx, response in zip(
range(len(conversations)), await model.abatch(list(conversations)), strict=True
)
]
# Sort by index to maintain order
responses_with_idx.sort(key=operator.itemgetter(0))
# Build the final data with enhanced metadata
rows: list[dict[str, Any]] = []
for idx, response in responses_with_idx:
resp_text = response.content if hasattr(response, "content") else str(response)
row = self._create_base_row(
text_input=user_texts[idx],
model_response=resp_text,
batch_index=idx,
)
self._add_metadata(row, success=True, system_msg=system_msg)
rows.append(row)
# Log progress
if (idx + 1) % max(1, total_rows // 10) == 0:
logger.info(f"Processed {idx + 1}/{total_rows} rows")
logger.info("Batch processing completed successfully")
return DataFrame(rows)
except (KeyError, AttributeError) as e:
# Handle data structure and attribute access errors
logger.error(f"Data processing error: {e!s}")
error_row = self._create_base_row()
self._add_metadata(error_row, success=False, error=str(e))
return DataFrame([error_row])
Create list
This component takes a list of text inputs and converts each text into a data object. These data objects are then collected into a list, which is returned as the output.
Parameters
Name | Display Name | Info |
---|---|---|
texts |
Texts |
Enter one or more texts. This input accepts multiple text entries. |
Display Name | Name | Info |
---|---|---|
Data List |
list |
A list of data objects created from the input texts. |
Component code
create_list.py
from langflow.custom import Component
from langflow.inputs import StrInput
from langflow.schema import Data
from langflow.schema.dataframe import DataFrame
from langflow.template import Output
class CreateListComponent(Component):
display_name = "Create List"
description = "Creates a list of texts."
icon = "list"
name = "CreateList"
legacy = True
inputs = [
StrInput(
name="texts",
display_name="Texts",
info="Enter one or more texts.",
is_list=True,
),
]
outputs = [
Output(display_name="Data List", name="list", method="create_list"),
Output(display_name="DataFrame", name="dataframe", method="as_dataframe"),
]
def create_list(self) -> list[Data]:
data = [Data(text=text) for text in self.texts]
self.status = data
return data
def as_dataframe(self) -> DataFrame:
"""Convert the list of Data objects into a DataFrame.
Returns:
DataFrame: A DataFrame containing the list data.
"""
return DataFrame(self.create_list())
Current date
The Current Date component returns the current date and time in a selected timezone. This component provides a flexible way to obtain timezone-specific date and time information within a Langflow pipeline.
Parameters
Name | Display Name | Info |
---|---|---|
timezone |
Timezone |
Select the timezone for the current date and time. |
Name | Display Name | Info |
---|---|---|
current_date |
Current Date |
The resulting current date and time in the selected timezone. |
Component code
current_date.py
from datetime import datetime
from zoneinfo import ZoneInfo, available_timezones
from loguru import logger
from langflow.custom import Component
from langflow.io import DropdownInput, Output
from langflow.schema.message import Message
class CurrentDateComponent(Component):
display_name = "Current Date"
description = "Returns the current date and time in the selected timezone."
icon = "clock"
name = "CurrentDate"
inputs = [
DropdownInput(
name="timezone",
display_name="Timezone",
options=list(available_timezones()),
value="UTC",
info="Select the timezone for the current date and time.",
tool_mode=True,
),
]
outputs = [
Output(display_name="Current Date", name="current_date", method="get_current_date"),
]
def get_current_date(self) -> Message:
try:
tz = ZoneInfo(self.timezone)
current_date = datetime.now(tz).strftime("%Y-%m-%d %H:%M:%S %Z")
result = f"Current date and time in {self.timezone}: {current_date}"
self.status = result
return Message(text=result)
except Exception as e: # noqa: BLE001
logger.opt(exception=True).debug("Error getting current date")
error_message = f"Error: {e}"
self.status = error_message
return Message(text=error_message)
Custom component
Use this component as a template to create your custom component.
Component code
custom_component.py
# from langflow.field_typing import Data
from langflow.custom import Component
from langflow.io import MessageTextInput, Output
from langflow.schema import Data
class CustomComponent(Component):
display_name = "Custom Component"
description = "Use as a template to create your own component."
documentation: str = "https://docs.langflow.org/components-custom-components"
icon = "code"
name = "CustomComponent"
inputs = [
MessageTextInput(
name="input_value",
display_name="Input Value",
info="This is a custom component Input",
value="Hello, World!",
tool_mode=True,
),
]
outputs = [
Output(display_name="Output", name="output", method="build_output"),
]
def build_output(self) -> Data:
data = Data(value=self.input_value)
self.status = data
return data
Hierarchical Task
This component creates and manages hierarchical tasks for CrewAI agents in a Playground environment.
For more information, see the CrewAI documentation.
Parameters
Name | Display Name | Info |
---|---|---|
task_description |
Description |
Descriptive text detailing task’s purpose and execution. |
expected_output |
Expected Output |
Clear definition of expected task outcome. |
tools |
Tools |
List of tools/resources limited for task execution. Uses the Agent tools by default. |
Name | Display Name | Info |
---|---|---|
task_output |
Task |
The built hierarchical task. |
Component code
hierarchical_task.py
from langflow.base.agents.crewai.tasks import HierarchicalTask
from langflow.custom import Component
from langflow.io import HandleInput, MultilineInput, Output
class HierarchicalTaskComponent(Component):
display_name: str = "Hierarchical Task"
description: str = "Each task must have a description, an expected output and an agent responsible for execution."
icon = "CrewAI"
inputs = [
MultilineInput(
name="task_description",
display_name="Description",
info="Descriptive text detailing task's purpose and execution.",
),
MultilineInput(
name="expected_output",
display_name="Expected Output",
info="Clear definition of expected task outcome.",
),
HandleInput(
name="tools",
display_name="Tools",
input_types=["Tool"],
is_list=True,
info="List of tools/resources limited for task execution. Uses the Agent tools by default.",
required=False,
advanced=True,
),
]
outputs = [
Output(display_name="Task", name="task_output", method="build_task"),
]
def build_task(self) -> HierarchicalTask:
task = HierarchicalTask(
description=self.task_description,
expected_output=self.expected_output,
tools=self.tools or [],
)
self.status = task
return task
ID generator
This component generates a unique ID.
Parameters
Name | Display Name | Info |
---|---|---|
value |
Value |
Unique ID generated. |
Component code
id_generator.py
import uuid
from typing import Any
from typing_extensions import override
from langflow.custom import Component
from langflow.io import MessageTextInput, Output
from langflow.schema import dotdict
from langflow.schema.message import Message
class IDGeneratorComponent(Component):
display_name = "ID Generator"
description = "Generates a unique ID."
icon = "fingerprint"
name = "IDGenerator"
inputs = [
MessageTextInput(
name="unique_id",
display_name="Value",
info="The generated unique ID.",
refresh_button=True,
tool_mode=True,
),
]
outputs = [
Output(display_name="ID", name="id", method="generate_id"),
]
@override
def update_build_config(self, build_config: dotdict, field_value: Any, field_name: str | None = None):
if field_name == "unique_id":
build_config[field_name]["value"] = str(uuid.uuid4())
return build_config
def generate_id(self) -> Message:
unique_id = self.unique_id or str(uuid.uuid4())
self.status = f"Generated ID: {unique_id}"
return Message(text=unique_id)
Message history
This component was named Chat Memory prior to Langflow version 1.1.0. |
This component retrieves and manages chat messages from Langflow tables or an external memory.
Parameters
Name | Display Name | Info |
---|---|---|
memory |
External Memory |
Retrieve messages from an external memory. If empty, it uses the Langflow tables. |
sender |
Sender Type |
Filter by sender type. |
sender_name |
Sender Name |
Filter by sender name. |
n_messages |
Number of Messages |
Number of messages to retrieve. |
session_id |
Session ID |
The session ID of the chat. If empty, the current session ID parameter is used. |
order |
Order |
Order of the messages. |
template |
Template |
The template to use for formatting the data. It can contain the keys {text}, {sender} or any other key in the message data. |
Name | Display Name | Info |
---|---|---|
messages |
Messages (data object) |
Retrieved messages as data objects. |
messages_text |
Messages (text) |
Retrieved messages formatted as text. |
lc_memory |
Memory |
The created LangChain-compatible memory object. |
Component code
memory.py
from langflow.custom import Component
from langflow.helpers.data import data_to_text
from langflow.inputs import HandleInput
from langflow.io import DropdownInput, IntInput, MessageTextInput, MultilineInput, Output
from langflow.memory import aget_messages
from langflow.schema import Data
from langflow.schema.dataframe import DataFrame
from langflow.schema.message import Message
from langflow.utils.constants import MESSAGE_SENDER_AI, MESSAGE_SENDER_USER
class MemoryComponent(Component):
display_name = "Message History"
description = "Retrieves stored chat messages from Langflow tables or an external memory."
icon = "message-square-more"
name = "Memory"
inputs = [
HandleInput(
name="memory",
display_name="External Memory",
input_types=["Memory"],
info="Retrieve messages from an external memory. If empty, it will use the Langflow tables.",
),
DropdownInput(
name="sender",
display_name="Sender Type",
options=[MESSAGE_SENDER_AI, MESSAGE_SENDER_USER, "Machine and User"],
value="Machine and User",
info="Filter by sender type.",
advanced=True,
),
MessageTextInput(
name="sender_name",
display_name="Sender Name",
info="Filter by sender name.",
advanced=True,
),
IntInput(
name="n_messages",
display_name="Number of Messages",
value=100,
info="Number of messages to retrieve.",
advanced=True,
),
MessageTextInput(
name="session_id",
display_name="Session ID",
info="The session ID of the chat. If empty, the current session ID parameter will be used.",
advanced=True,
),
DropdownInput(
name="order",
display_name="Order",
options=["Ascending", "Descending"],
value="Ascending",
info="Order of the messages.",
advanced=True,
tool_mode=True,
),
MultilineInput(
name="template",
display_name="Template",
info="The template to use for formatting the data. "
"It can contain the keys {text}, {sender} or any other key in the message data.",
value="{sender_name}: {text}",
advanced=True,
),
]
outputs = [
Output(display_name="Data", name="messages", method="retrieve_messages"),
Output(display_name="Message", name="messages_text", method="retrieve_messages_as_text"),
Output(display_name="DataFrame", name="dataframe", method="as_dataframe"),
]
async def retrieve_messages(self) -> Data:
sender = self.sender
sender_name = self.sender_name
session_id = self.session_id
n_messages = self.n_messages
order = "DESC" if self.order == "Descending" else "ASC"
if sender == "Machine and User":
sender = None
if self.memory and not hasattr(self.memory, "aget_messages"):
memory_name = type(self.memory).__name__
err_msg = f"External Memory object ({memory_name}) must have 'aget_messages' method."
raise AttributeError(err_msg)
if self.memory:
# override session_id
self.memory.session_id = session_id
stored = await self.memory.aget_messages()
# langchain memories are supposed to return messages in ascending order
if order == "DESC":
stored = stored[::-1]
if n_messages:
stored = stored[:n_messages]
stored = [Message.from_lc_message(m) for m in stored]
if sender:
expected_type = MESSAGE_SENDER_AI if sender == MESSAGE_SENDER_AI else MESSAGE_SENDER_USER
stored = [m for m in stored if m.type == expected_type]
else:
stored = await aget_messages(
sender=sender,
sender_name=sender_name,
session_id=session_id,
limit=n_messages,
order=order,
)
self.status = stored
return stored
async def retrieve_messages_as_text(self) -> Message:
stored_text = data_to_text(self.template, await self.retrieve_messages())
self.status = stored_text
return Message(text=stored_text)
async def as_dataframe(self) -> DataFrame:
"""Convert the retrieved messages into a DataFrame.
Returns:
DataFrame: A DataFrame containing the message data.
"""
messages = await self.retrieve_messages()
return DataFrame(messages)
Sequential task
This component creates and manage sequential tasks for CrewAI agents. It builds a SequentialTask object with the provided description, expected output, and agent, allowing for the specification of tools and asynchronous execution.
For more information, see the CrewAI documentation.
Parameters
Name | Display Name | Info |
---|---|---|
task_description |
Description |
Descriptive text detailing task’s purpose and execution. |
expected_output |
Expected Output |
Clear definition of expected task outcome. |
tools |
Tools |
List of tools/resources limited for task execution. Uses the Agent tools by default. |
agent |
Agent |
CrewAI Agent that will perform the task. |
task |
Task |
CrewAI Task that will perform the task. |
async_execution |
Async Execution |
Boolean flag indicating asynchronous task execution. |
Name | Display Name | Info |
---|---|---|
task_output |
Task |
The built sequential task or list of tasks. |
Component code
sequential_task.py
from langflow.base.agents.crewai.tasks import SequentialTask
from langflow.custom import Component
from langflow.io import BoolInput, HandleInput, MultilineInput, Output
class SequentialTaskComponent(Component):
display_name: str = "Sequential Task"
description: str = "Each task must have a description, an expected output and an agent responsible for execution."
icon = "CrewAI"
inputs = [
MultilineInput(
name="task_description",
display_name="Description",
info="Descriptive text detailing task's purpose and execution.",
),
MultilineInput(
name="expected_output",
display_name="Expected Output",
info="Clear definition of expected task outcome.",
),
HandleInput(
name="tools",
display_name="Tools",
input_types=["Tool"],
is_list=True,
info="List of tools/resources limited for task execution. Uses the Agent tools by default.",
required=False,
advanced=True,
),
HandleInput(
name="agent",
display_name="Agent",
input_types=["Agent"],
info="CrewAI Agent that will perform the task",
required=True,
),
HandleInput(
name="task",
display_name="Task",
input_types=["SequentialTask"],
info="CrewAI Task that will perform the task",
),
BoolInput(
name="async_execution",
display_name="Async Execution",
value=True,
advanced=True,
info="Boolean flag indicating asynchronous task execution.",
),
]
outputs = [
Output(display_name="Task", name="task_output", method="build_task"),
]
def build_task(self) -> list[SequentialTask]:
tasks: list[SequentialTask] = []
task = SequentialTask(
description=self.task_description,
expected_output=self.expected_output,
tools=self.agent.tools,
async_execution=False,
agent=self.agent,
)
tasks.append(task)
self.status = task
if self.task:
if isinstance(self.task, list) and all(isinstance(task, SequentialTask) for task in self.task):
tasks = self.task + tasks
elif isinstance(self.task, SequentialTask):
tasks = [self.task, *tasks]
return tasks
Message store
This component stores chat messages or text into Langflow tables or an external memory.
It provides flexibility in managing message storage and retrieval within a chat system.
Parameters
Name | Display Name | Info |
---|---|---|
message |
Message |
The chat message to be stored. (Required) |
memory |
External Memory |
The external memory to store the message. If empty, it will use the Langflow tables. |
sender |
Sender |
The sender of the message. Can be Machine or User. If empty, the current sender parameter will be used. |
sender_name |
Sender Name |
The name of the sender. Can be AI or User. If empty, the current sender parameter will be used. |
session_id |
Session ID |
The session ID of the chat. If empty, the current session ID parameter will be used. |
Name | Display Name | Info |
---|---|---|
stored_messages |
Stored Messages |
The list of stored messages after the current message has been added. |
Component code
store_message.py
from langflow.custom import Component
from langflow.inputs import HandleInput
from langflow.inputs.inputs import MessageTextInput
from langflow.memory import aget_messages, astore_message
from langflow.schema.message import Message
from langflow.template import Output
from langflow.utils.constants import MESSAGE_SENDER_AI, MESSAGE_SENDER_NAME_AI
class MessageStoreComponent(Component):
display_name = "Message Store"
description = "Stores a chat message or text into Langflow tables or an external memory."
icon = "message-square-text"
name = "StoreMessage"
inputs = [
MessageTextInput(
name="message", display_name="Message", info="The chat message to be stored.", required=True, tool_mode=True
),
HandleInput(
name="memory",
display_name="External Memory",
input_types=["Memory"],
info="The external memory to store the message. If empty, it will use the Langflow tables.",
),
MessageTextInput(
name="sender",
display_name="Sender",
info="The sender of the message. Might be Machine or User. "
"If empty, the current sender parameter will be used.",
advanced=True,
),
MessageTextInput(
name="sender_name",
display_name="Sender Name",
info="The name of the sender. Might be AI or User. If empty, the current sender parameter will be used.",
advanced=True,
),
MessageTextInput(
name="session_id",
display_name="Session ID",
info="The session ID of the chat. If empty, the current session ID parameter will be used.",
value="",
advanced=True,
),
]
outputs = [
Output(display_name="Stored Messages", name="stored_messages", method="store_message", hidden=True),
]
async def store_message(self) -> Message:
message = Message(text=self.message) if isinstance(self.message, str) else self.message
message.session_id = self.session_id or message.session_id
message.sender = self.sender or message.sender or MESSAGE_SENDER_AI
message.sender_name = self.sender_name or message.sender_name or MESSAGE_SENDER_NAME_AI
stored_messages: list[Message] = []
if self.memory:
self.memory.session_id = message.session_id
lc_message = message.to_lc_message()
await self.memory.aadd_messages([lc_message])
stored_messages = await self.memory.aget_messages() or []
stored_messages = [Message.from_lc_message(m) for m in stored_messages] if stored_messages else []
if message.sender:
stored_messages = [m for m in stored_messages if m.sender == message.sender]
else:
await astore_message(message, flow_id=self.graph.flow_id)
stored_messages = (
await aget_messages(
session_id=message.session_id, sender_name=message.sender_name, sender=message.sender
)
or []
)
if not stored_messages:
msg = "No messages were stored. Please ensure that the session ID and sender are properly set."
raise ValueError(msg)
stored_message = stored_messages[0]
self.status = stored_message
return stored_message
Structured output
This component transforms LLM responses into structured data formats.
Use the structured output component in a flow
In this example from the Financial Support Parser template, the Structured Output component transforms unstructured financial reports into structured data.
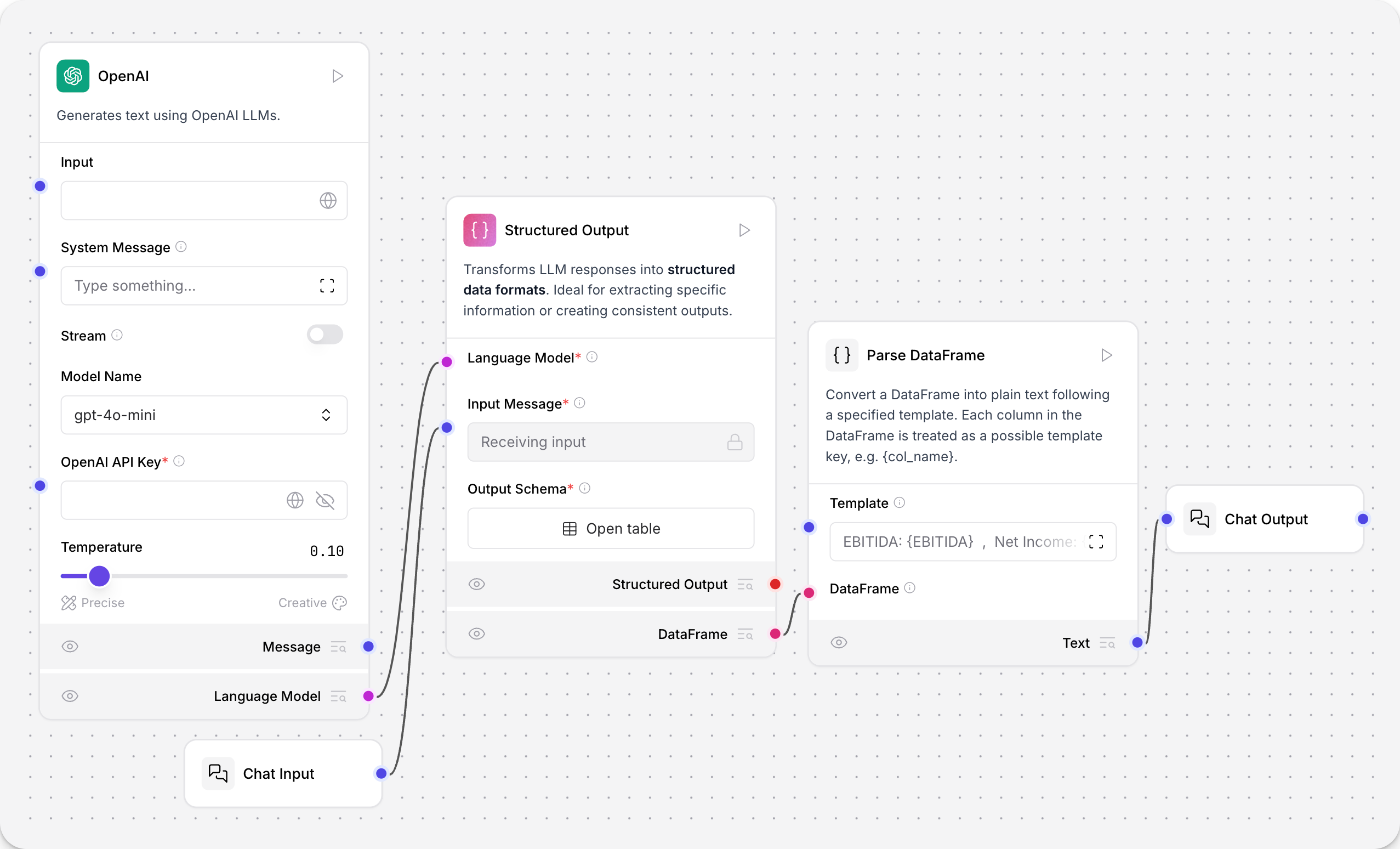
The connected LLM model is prompted by the Structured Output component’s system_prompt
parameter to extract structured output from the unstructured text.
In the Structured Output component, click the Open table button to view the output_schema
table.
The output_schema
parameter defines the structure and data types for the model’s output using a table with the following fields:
-
Name: The name of the output field.
-
Description: The purpose of the output field.
-
Type: The data type of the output field. The available types are
str
,int
,float
,bool
,list
, ordict
. Default:text
. -
Multiple: Set to
True
if you expect multiple values for a single field. For example, a list of features is set totrue
to contain multiple values, such as["waterproof", "durable", "lightweight"]
. Default:True
.
The Parse DataFrame component parses the structured output into a template for orderly presentation in chat output. The template receives the values from the output_schema
table with curly braces.
For example, the template EBITDA: {EBITDA} , Net Income: {NET_INCOME} , GROSS_PROFIT: {GROSS_PROFIT}
presents the extracted values in the Playground as EBITDA: 900 million , Net Income: 500 million , GROSS_PROFIT: 1.2 billion
.
Parameters
Name | Display Name | Info |
---|---|---|
llm |
Language Model |
The language model to use to generate the structured output. |
input_value |
Input Message |
The input message to the language model. |
system_prompt |
Format Instructions |
Instructions to the language model for formatting the output. |
schema_name |
Schema Name |
The name for the output data schema. |
output_schema |
Output Schema |
The structure and data types for the model’s output. |
multiple |
Generate Multiple |
[Deprecated] Always set to True. |
Name | Display Name | Info |
---|---|---|
structured_output |
Structured Output |
The structured output based on the defined schema. |
structured_output_dataframe |
DataFrame |
The structured output converted to a DataFrame format. |
Component code
structured_output.py
from typing import TYPE_CHECKING, cast
from pydantic import BaseModel, Field, create_model
from langflow.base.models.chat_result import get_chat_result
from langflow.custom import Component
from langflow.helpers.base_model import build_model_from_schema
from langflow.io import (
BoolInput,
HandleInput,
MessageTextInput,
MultilineInput,
Output,
TableInput,
)
from langflow.schema.data import Data
from langflow.schema.dataframe import DataFrame
from langflow.schema.table import EditMode
if TYPE_CHECKING:
from langflow.field_typing.constants import LanguageModel
class StructuredOutputComponent(Component):
display_name = "Structured Output"
description = (
"Transforms LLM responses into **structured data formats**. Ideal for extracting specific information "
"or creating consistent outputs."
)
name = "StructuredOutput"
icon = "braces"
inputs = [
HandleInput(
name="llm",
display_name="Language Model",
info="The language model to use to generate the structured output.",
input_types=["LanguageModel"],
required=True,
),
MessageTextInput(
name="input_value",
display_name="Input Message",
info="The input message to the language model.",
tool_mode=True,
required=True,
),
MultilineInput(
name="system_prompt",
display_name="Format Instructions",
info="The instructions to the language model for formatting the output.",
value=(
"You are an AI system designed to extract structured information from unstructured text."
"Given the input_text, return a JSON object with predefined keys based on the expected structure."
"Extract values accurately and format them according to the specified type "
"(e.g., string, integer, float, date)."
"If a value is missing or cannot be determined, return a default "
"(e.g., null, 0, or 'N/A')."
"If multiple instances of the expected structure exist within the input_text, "
"stream each as a separate JSON object."
),
required=True,
advanced=True,
),
MessageTextInput(
name="schema_name",
display_name="Schema Name",
info="Provide a name for the output data schema.",
advanced=True,
),
TableInput(
name="output_schema",
display_name="Output Schema",
info="Define the structure and data types for the model's output.",
required=True,
# TODO: remove deault value
table_schema=[
{
"name": "name",
"display_name": "Name",
"type": "str",
"description": "Specify the name of the output field.",
"default": "field",
"edit_mode": EditMode.INLINE,
},
{
"name": "description",
"display_name": "Description",
"type": "str",
"description": "Describe the purpose of the output field.",
"default": "description of field",
"edit_mode": EditMode.POPOVER,
},
{
"name": "type",
"display_name": "Type",
"type": "str",
"edit_mode": EditMode.INLINE,
"description": (
"Indicate the data type of the output field (e.g., str, int, float, bool, list, dict)."
),
"options": ["str", "int", "float", "bool", "list", "dict"],
"default": "str",
},
{
"name": "multiple",
"display_name": "Multiple",
"type": "boolean",
"description": "Set to True if this output field should be a list of the specified type.",
"default": "False",
"edit_mode": EditMode.INLINE,
},
],
value=[
{
"name": "field",
"description": "description of field",
"type": "str",
"multiple": "False",
}
],
),
BoolInput(
name="multiple",
advanced=True,
display_name="Generate Multiple",
info="[Deplrecated] Always set to True",
value=True,
),
]
outputs = [
Output(
name="structured_output",
display_name="Structured Output",
method="build_structured_output",
),
Output(
name="structured_output_dataframe",
display_name="DataFrame",
method="as_dataframe",
),
]
def build_structured_output_base(self) -> Data:
schema_name = self.schema_name or "OutputModel"
if not hasattr(self.llm, "with_structured_output"):
msg = "Language model does not support structured output."
raise TypeError(msg)
if not self.output_schema:
msg = "Output schema cannot be empty"
raise ValueError(msg)
output_model_ = build_model_from_schema(self.output_schema)
output_model = create_model(
schema_name,
objects=(list[output_model_], Field(description=f"A list of {schema_name}.")), # type: ignore[valid-type]
)
try:
llm_with_structured_output = cast("LanguageModel", self.llm).with_structured_output(schema=output_model) # type: ignore[valid-type, attr-defined]
except NotImplementedError as exc:
msg = f"{self.llm.__class__.__name__} does not support structured output."
raise TypeError(msg) from exc
config_dict = {
"run_name": self.display_name,
"project_name": self.get_project_name(),
"callbacks": self.get_langchain_callbacks(),
}
result = get_chat_result(
runnable=llm_with_structured_output,
system_message=self.system_prompt,
input_value=self.input_value,
config=config_dict,
)
if isinstance(result, BaseModel):
result = result.model_dump()
if "objects" in result:
return result["objects"]
return result
def build_structured_output(self) -> Data:
output = self.build_structured_output_base()
return Data(results=output)
def as_dataframe(self) -> DataFrame:
output = self.build_structured_output_base()
if isinstance(output, list):
return DataFrame(data=output)
return DataFrame(data=[output])