Models
This Langflow feature is currently in public preview. Development is ongoing, and the features and functionality are subject to change. Langflow, and the use of such, is subject to the DataStax Preview Terms. |
Model components generate text using large language models.
Refer to your specific component’s documentation for more information on parameters.
Use a model component in a flow
Model components receive inputs and prompts for generating text, and the generated text is sent to an output component.
The model output can also be sent to the Language Model port and on to a Parse Data component, where the output can be parsed into structured Data
objects.
This example has the OpenAI model in a chatbot flow. For more information, see the Quickstart.
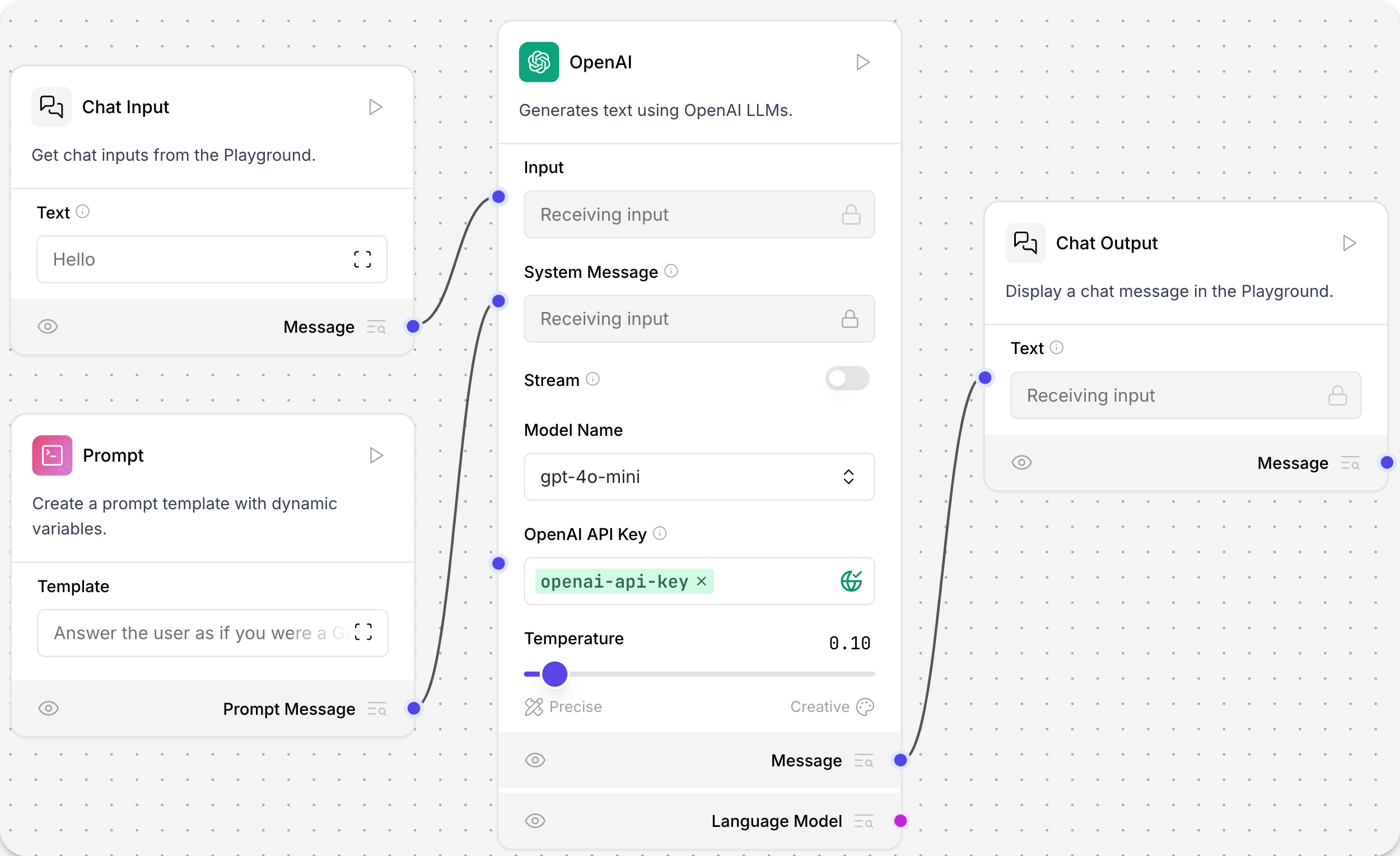
AI/ML API
This component creates a ChatOpenAI model instance using the AIML API.
For more information, see AIML documentation.
Parameters
Name | Type | Description |
---|---|---|
max_tokens |
Integer |
The maximum number of tokens to generate. Set to 0 for unlimited tokens. Range: 0-128000. |
model_kwargs |
Dictionary |
Additional keyword arguments for the model. |
model_name |
String |
The name of the AIML model to use. Options are predefined in AIML_CHAT_MODELS. |
aiml_api_base |
String |
The base URL of the AIML API. Defaults to https://api.aimlapi.com. |
api_key |
SecretString |
The AIML API Key to use for the model. |
temperature |
Float |
Controls randomness in the output. Default: 0.1. |
seed |
Integer |
Controls reproducibility of the job. |
Name | Type | Description |
---|---|---|
model |
LanguageModel |
An instance of ChatOpenAI configured with the specified parameters. |
Component code
aiml.py
404: Not Found
Amazon Bedrock
This component generates text using Amazon Bedrock LLMs.
For more information, see Amazon Bedrock documentation.
Parameters
Name | Type | Description |
---|---|---|
model_id |
String |
The ID of the Amazon Bedrock model to use. Options include various models from Amazon, Anthropic, AI21, Cohere, Meta, Mistral, and Stability AI. |
aws_access_key |
SecretString |
AWS Access Key for authentication. |
aws_secret_key |
SecretString |
AWS Secret Key for authentication. |
credentials_profile_name |
String |
Name of the AWS credentials profile to use (advanced). |
region_name |
String |
AWS region name. Default: "us-east-1". |
model_kwargs |
Dictionary |
Additional keyword arguments for the model (advanced). |
endpoint_url |
String |
Custom endpoint URL for the Bedrock service (advanced). |
Name | Type | Description |
---|---|---|
model |
LanguageModel |
An instance of ChatBedrock configured with the specified parameters. |
Component code
amazon_bedrock.py
404: Not Found
Anthropic
This component allows the generation of text using Anthropic Chat and Language models.
For more information, see the Anthropic documentation.
Parameters
Name | Type | Description |
---|---|---|
max_tokens |
Integer |
The maximum number of tokens to generate. Set to 0 for unlimited tokens. Default: 4096. |
model |
String |
The name of the Anthropic model to use. Options include various Claude 3 models. |
anthropic_api_key |
SecretString |
Your Anthropic API key for authentication. |
temperature |
Float |
Controls randomness in the output. Default: 0.1. |
anthropic_api_url |
String |
Endpoint of the Anthropic API. Defaults to 'https://api.anthropic.com' if not specified (advanced). |
prefill |
String |
Prefill text to guide the model’s response (advanced). |
Name | Type | Description |
---|---|---|
model |
LanguageModel |
An instance of ChatAnthropic configured with the specified parameters. |
Component code
anthropic.py
404: Not Found
Azure OpenAI
This component generates text using Azure OpenAI LLM.
For more information, see the Azure OpenAI documentation.
Parameters
Name | Display Name | Info |
---|---|---|
Model Name |
Model Name |
Specifies the name of the Azure OpenAI model to be used for text generation. |
Azure Endpoint |
Azure Endpoint |
Your Azure endpoint, including the resource. |
Deployment Name |
Deployment Name |
Specifies the name of the deployment. |
API Version |
API Version |
Specifies the version of the Azure OpenAI API to be used. |
API Key |
API Key |
Your Azure OpenAI API key. |
Temperature |
Temperature |
Specifies the sampling temperature. Defaults to |
Max Tokens |
Max Tokens |
Specifies the maximum number of tokens to generate. Defaults to |
Input Value |
Input Value |
Specifies the input text for text generation. |
Stream |
Stream |
Specifies whether to stream the response from the model. Defaults to |
Name | Type | Description |
---|---|---|
model |
LanguageModel |
An instance of AzureOpenAI configured with the specified parameters. |
Component code
azure_openai.py
404: Not Found
Azure OpenAI component error
If you’re encountering the following error when building the Azure OpenAI component:
Error building Component Azure OpenAI: 1 validation error for MessageTextInput value Value error, Invalid value type <class 'NoneType'> [type=value_error, input_value=None, input_type=NoneType]
Ensure that the MessageTextInput
fields (input_value
, sender_name
, session_id
) are correctly defined and used in the message_response
method.
Ensure that the values from your Azure deployment, like resource groups, subscriptions, and regions, are being passed correctly to the component. For more information, see the Azure OpenAI documentation.
Cohere
This component generates text using Cohere’s language models.
For more information, see the Cohere documentation.
Parameters
Name | Display Name | Info |
---|---|---|
Cohere API Key |
Cohere API Key |
Your Cohere API key. |
Max Tokens |
Max Tokens |
Specifies the maximum number of tokens to generate. Defaults to |
Temperature |
Temperature |
Specifies the sampling temperature. Defaults to |
Input Value |
Input Value |
Specifies the input text for text generation. |
Name | Type | Description |
---|---|---|
model |
LanguageModel |
An instance of the Cohere model configured with the specified parameters. |
Component code
cohere.py
404: Not Found
DeepSeek
This component generates text using DeepSeek’s language models. For more information, see the DeepSeek documentation.
Parameters
Name | Type | Description |
---|---|---|
max_tokens |
Integer |
Maximum number of tokens to generate. Set to 0 for unlimited. Range: 0-128000. |
model_kwargs |
Dictionary |
Additional keyword arguments for the model. |
json_mode |
Boolean |
If True, outputs JSON regardless of passing a schema. |
model_name |
String |
The DeepSeek model to use. Default: deepseek-chat. |
api_base |
String |
Base URL for API requests. Default: |
api_key |
SecretString |
Your DeepSeek API key for authentication. |
temperature |
Float |
Controls randomness in responses. Range: [0.0, 2.0]. Default: 1.0. |
seed |
Integer |
Number initialized for random number generation. Use the same seed integer for more reproducible results, and use a different seed number for more random results. |
Name | Type | Description |
---|---|---|
model |
LanguageModel |
An instance of ChatOpenAI configured with the specified parameters. |
Component code
deepseek.py
404: Not Found
Google Generative AI
This component generates text using Google’s Generative AI models.
For more information, see the Google Generative AI documentation.
Parameters
Name | Display Name | Info |
---|---|---|
Google API Key |
Google API Key |
Your Google API key to use for the Google Generative AI. |
Model |
Model |
The name of the model to use, such as |
Max Output Tokens |
Max Output Tokens |
The maximum number of tokens to generate. |
Temperature |
Temperature |
Run inference with this temperature. |
Top K |
Top K |
Consider the set of top K most probable tokens. |
Top P |
Top P |
The maximum cumulative probability of tokens to consider when sampling. |
N |
N |
Number of chat completions to generate for each prompt. |
Component code
google_generative_ai.py
404: Not Found
Groq
This component generates text using Groq’s language models.
For more information, see the Groq documentation.
Parameters
Name | Type | Description |
---|---|---|
groq_api_key |
SecretString |
API key for the Groq API. |
groq_api_base |
String |
Base URL path for API requests. Default: "https://api.groq.com" (advanced). |
max_tokens |
Integer |
The maximum number of tokens to generate (advanced). |
temperature |
Float |
Controls randomness in the output. Range: [0.0, 1.0]. Default: 0.1. |
n |
Integer |
Number of chat completions to generate for each prompt (advanced). |
model_name |
String |
The name of the Groq model to use. Options are dynamically fetched from the Groq API. |
Name | Type | Description |
---|---|---|
model |
LanguageModel |
An instance of ChatGroq configured with the specified parameters. |
Component code
groq.py
404: Not Found
Hugging Face API
This component sends requests to the Hugging Face API to generate text using the model specified in the Model ID field.
The Hugging Face API is a hosted inference API for models hosted on Hugging Face, and requires a Hugging Face API token to authenticate.
In this example based on the Basic prompting flow, the Hugging Face API model component replaces the Open AI model. By selecting different hosted models, you can see how different models return different results.
-
Create a Basic prompting flow.
-
Replace the OpenAI model component with a Hugging Face API model component.
-
In the Hugging Face API component, add your Hugging Face API token to the API Token field.
-
Open the Playground and ask a question to the model, and then see how it responds.
-
Try different models to see how they perform differently.
For more information, see the Hugging Face documentation.
Parameters
Name | Display Name | Info |
---|---|---|
model_id |
String |
The model ID from Hugging Face Hub. For example, "gpt2", "facebook/bart-large". |
huggingfacehub_api_token |
SecretString |
Your Hugging Face API token for authentication. |
temperature |
Float |
Controls randomness in the output. Range: [0.0, 1.0]. Default: 0.7. |
max_new_tokens |
Integer |
Maximum number of tokens to generate. Default: 512. |
top_p |
Float |
Nucleus sampling parameter. Range: [0.0, 1.0]. Default: 0.95. |
top_k |
Integer |
Top-k sampling parameter. Default: 50. |
model_kwargs |
Dictionary |
Additional keyword arguments to pass to the model. |
Name | Type | Description |
---|---|---|
model |
LanguageModel |
An instance of Hugging Face configured with the specified parameters. |
Component code
huggingface.py
404: Not Found
IBM watsonx.ai
This component generates text using IBM watsonx.ai foundation models.
To use IBM watsonx.ai model components, replace a model component with the IBM watsonx.ai component in a flow.
An example flow looks like the following:
The values for API endpoint, Project ID, API key, and Model Name are found in your IBM watsonx.ai deployment. For more information, see the Langchain documentation.
Name | Type | Description |
---|---|---|
url |
String |
The base URL of the watsonx API. |
project_id |
String |
Your watsonx Project ID. |
api_key |
SecretString |
Your IBM watsonx API Key. |
model_name |
String |
The name of the watsonx model to use. Options are dynamically fetched from the API. |
max_tokens |
Integer |
The maximum number of tokens to generate. Default: |
stop_sequence |
String |
The sequence where generation should stop. |
temperature |
Float |
Controls randomness in the output. Default: |
top_p |
Float |
Controls nucleus sampling, which limits the model to tokens whose probability is below the |
frequency_penalty |
Float |
Controls frequency penalty. A positive value decreases the probability of repeating tokens, and a negative value increases the probability. Range: Default: |
presence_penalty |
Float |
Controls presence penalty. A positive value increases the likelihood of new topics being introduced. Default: |
seed |
Integer |
A random seed for the model. Default: |
logprobs |
Boolean |
Whether to return log probabilities of output tokens or not. Default: |
top_logprobs |
Integer |
The number of most likely tokens to return at each position. Default: |
logit_bias |
String |
A JSON string of token IDs to bias or suppress. |
Name | Type | Description |
---|---|---|
model |
LanguageModel |
An instance of ChatWatsonx configured with the specified parameters. |
Component code
watsonx.py
404: Not Found
Language model
This component generates text using either OpenAI or Anthropic language models.
Use this component as a drop-in replacement for LLM models to switch between different model providers and models.
Instead of swapping out model components when you want to try a different provider, like switching between OpenAI and Anthropic components, change the provider dropdown in this single component. This makes it easier to experiment with and compare different models while keeping the rest of your flow intact.
For more information, see the OpenAI documentation and Anthropic documentation.
Parameters
Name | Type | Description |
---|---|---|
provider |
String |
The model provider to use. Options: "OpenAI", "Anthropic". Default: "OpenAI". |
model_name |
String |
The name of the model to use. Options depend on the selected provider. |
api_key |
SecretString |
The API Key for authentication with the selected provider. |
input_value |
String |
The input text to send to the model. |
system_message |
String |
A system message that helps set the behavior of the assistant. |
stream |
Boolean |
Whether to stream the response. Default: |
temperature |
Float |
Controls randomness in responses. Range: |
Name | Type | Description |
---|---|---|
model |
LanguageModel |
An instance of ChatOpenAI or ChatAnthropic configured with the specified parameters. |
Component code
language_model.py
from typing import Any
from langchain_anthropic import ChatAnthropic
from langchain_google_genai import ChatGoogleGenerativeAI
from langchain_openai import ChatOpenAI
from langflow.base.models.anthropic_constants import ANTHROPIC_MODELS
from langflow.base.models.google_generative_ai_constants import GOOGLE_GENERATIVE_AI_MODELS
from langflow.base.models.model import LCModelComponent
from langflow.base.models.openai_constants import OPENAI_MODEL_NAMES
from langflow.field_typing import LanguageModel
from langflow.field_typing.range_spec import RangeSpec
from langflow.inputs.inputs import BoolInput
from langflow.io import DropdownInput, MessageInput, MultilineInput, SecretStrInput, SliderInput
from langflow.schema.dotdict import dotdict
class LanguageModelComponent(LCModelComponent):
display_name = "Language Model"
description = "Runs a language model given a specified provider. "
icon = "brain-circuit"
category = "models"
priority = 0 # Set priority to 0 to make it appear first
inputs = [
DropdownInput(
name="provider",
display_name="Model Provider",
options=["OpenAI", "Anthropic", "Google"],
value="OpenAI",
info="Select the model provider",
real_time_refresh=True,
options_metadata=[{"icon": "OpenAI"}, {"icon": "Anthropic"}, {"icon": "Google"}],
),
DropdownInput(
name="model_name",
display_name="Model Name",
options=OPENAI_MODEL_NAMES,
value=OPENAI_MODEL_NAMES[0],
info="Select the model to use",
),
SecretStrInput(
name="api_key",
display_name="OpenAI API Key",
info="Model Provider API key",
required=False,
show=True,
real_time_refresh=True,
),
MessageInput(
name="input_value",
display_name="Input",
info="The input text to send to the model",
),
MultilineInput(
name="system_message",
display_name="System Message",
info="A system message that helps set the behavior of the assistant",
advanced=True,
),
BoolInput(
name="stream",
display_name="Stream",
info="Whether to stream the response",
value=False,
advanced=True,
),
SliderInput(
name="temperature",
display_name="Temperature",
value=0.1,
info="Controls randomness in responses",
range_spec=RangeSpec(min=0, max=1, step=0.01),
advanced=True,
),
]
def build_model(self) -> LanguageModel:
provider = self.provider
model_name = self.model_name
temperature = self.temperature
stream = self.stream
if provider == "OpenAI":
if not self.api_key:
msg = "OpenAI API key is required when using OpenAI provider"
raise ValueError(msg)
return ChatOpenAI(
model_name=model_name,
temperature=temperature,
streaming=stream,
openai_api_key=self.api_key,
)
if provider == "Anthropic":
if not self.api_key:
msg = "Anthropic API key is required when using Anthropic provider"
raise ValueError(msg)
return ChatAnthropic(
model=model_name,
temperature=temperature,
streaming=stream,
anthropic_api_key=self.api_key,
)
if provider == "Google":
if not self.api_key:
msg = "Google API key is required when using Google provider"
raise ValueError(msg)
return ChatGoogleGenerativeAI(
model=model_name,
temperature=temperature,
streaming=stream,
google_api_key=self.api_key,
)
msg = f"Unknown provider: {provider}"
raise ValueError(msg)
def update_build_config(self, build_config: dotdict, field_value: Any, field_name: str | None = None) -> dotdict:
if field_name == "provider":
if field_value == "OpenAI":
build_config["model_name"]["options"] = OPENAI_MODEL_NAMES
build_config["model_name"]["value"] = OPENAI_MODEL_NAMES[0]
build_config["api_key"]["display_name"] = "OpenAI API Key"
elif field_value == "Anthropic":
build_config["model_name"]["options"] = ANTHROPIC_MODELS
build_config["model_name"]["value"] = ANTHROPIC_MODELS[0]
build_config["api_key"]["display_name"] = "Anthropic API Key"
elif field_value == "Google":
build_config["model_name"]["options"] = GOOGLE_GENERATIVE_AI_MODELS
build_config["model_name"]["value"] = GOOGLE_GENERATIVE_AI_MODELS[0]
build_config["api_key"]["display_name"] = "Google API Key"
return build_config
LM Studio
This component generates text using LM Studiolocal language models.
Parameters
Name | Type | Description |
---|---|---|
base_url |
String |
The URL where LM Studio is running. Default: "http://localhost:1234". |
max_tokens |
Integer |
Maximum number of tokens to generate in the response. Default: 512. |
temperature |
Float |
Controls randomness in the output. Range: [0.0, 2.0]. Default: 0.7. |
top_p |
Float |
Controls diversity via nucleus sampling. Range: [0.0, 1.0]. Default: 1.0. |
stop |
List[String] |
List of strings that stop generation when encountered (advanced). |
stream |
Boolean |
Whether to stream the response. Default: False. |
presence_penalty |
Float |
Penalizes repeated tokens. Range: [-2.0, 2.0]. Default: 0.0. |
frequency_penalty |
Float |
Penalizes frequent tokens. Range: [-2.0, 2.0]. Default: 0.0. |
Name | Type | Description |
---|---|---|
model |
LanguageModel |
An instance of LM Studio configured with the specified parameters. |
Component code
lmstudiomodel.py
404: Not Found
Maritalk
This component generates text using Maritalk LLMs. For more information, see Maritalk documentation.
Parameters
Name | Type | Description |
---|---|---|
max_tokens |
Integer |
The maximum number of tokens to generate. Set to 0 for unlimited tokens. Default: 512. |
model_name |
String |
The name of the Maritalk model to use. Options: "sabia-2-small", "sabia-2-medium". Default: "sabia-2-small". |
api_key |
SecretString |
The Maritalk API Key to use for authentication. |
temperature |
Float |
Controls randomness in the output. Range: [0, 1]. Default: 0.1. |
Name | Type | Description |
---|---|---|
model |
LanguageModel |
An instance of ChatMaritalk configured with the specified parameters. |
Component code
maritalk.py
404: Not Found
Mistral
This component generates text using MistralAI LLMs.
For more information, see Mistral AI documentation.
Parameters
Name | Type | Description |
---|---|---|
max_tokens |
Integer |
The maximum number of tokens to generate. Set to 0 for unlimited tokens (advanced). |
model_name |
String |
The name of the Mistral AI model to use. Options include "open-mixtral-8x7b", "open-mixtral-8x22b", "mistral-small-latest", "mistral-medium-latest", "mistral-large-latest", and "codestral-latest". Default: "codestral-latest". |
mistral_api_base |
String |
The base URL of the Mistral API. Defaults to https://api.mistral.ai/v1 (advanced). |
api_key |
SecretString |
The Mistral API Key to use for authentication. |
temperature |
Float |
Controls randomness in the output. Default: 0.5. |
max_retries |
Integer |
Maximum number of retries for API calls. Default: 5 (advanced). |
timeout |
Integer |
Timeout for API calls in seconds. Default: 60 (advanced). |
max_concurrent_requests |
Integer |
Maximum number of concurrent API requests. Default: 3 (advanced). |
top_p |
Float |
Nucleus sampling parameter. Default: 1 (advanced). |
random_seed |
Integer |
Seed for random number generation. Default: 1 (advanced). |
safe_mode |
Boolean |
Enables safe mode for content generation (advanced). |
Name | Type | Description |
---|---|---|
model |
LanguageModel |
An instance of ChatMistralAI configured with the specified parameters. |
Component code
mistral.py
404: Not Found
Novita AI
This component generates text using Novita AI’s language models. For more information, see the Novita AI documentation.
Parameters
Name | Type | Description |
---|---|---|
api_key |
SecretString |
Your Novita AI API Key. |
model |
String |
The id of the Novita AI model to use. |
max_tokens |
Integer |
The maximum number of tokens to generate. Set to 0 for unlimited tokens. |
temperature |
Float |
Controls randomness in the output. Range: [0.0, 1.0]. Default: 0.7. |
top_p |
Float |
Controls the nucleus sampling. Range: [0.0, 1.0]. Default: 1.0. |
frequency_penalty |
Float |
Controls the frequency penalty. Range: [0.0, 2.0]. Default: 0.0. |
presence_penalty |
Float |
Controls the presence penalty. Range: [0.0, 2.0]. Default: 0.0. |
Name | Type | Description |
---|---|---|
model |
LanguageModel |
An instance of Novita AI model configured with the specified parameters. |
Component code
novita.py
404: Not Found
NVIDIA
This component generates text using NVIDIA LLMs.
For more information, see NVIDIA AI Foundation Models documentation.
Parameters
Name | Type | Description |
---|---|---|
max_tokens |
Integer |
The maximum number of tokens to generate. Set to 0 for unlimited tokens (advanced). |
model_name |
String |
The name of the NVIDIA model to use. Default: "mistralai/mixtral-8x7b-instruct-v0.1". |
base_url |
String |
The base URL of the NVIDIA API. Default: "https://integrate.api.nvidia.com/v1". |
nvidia_api_key |
SecretString |
The NVIDIA API Key for authentication. |
temperature |
Float |
Controls randomness in the output. Default: 0.1. |
seed |
Integer |
The seed controls the reproducibility of the job (advanced). Default: 1. |
Name | Type | Description |
---|---|---|
model |
LanguageModel |
An instance of ChatNVIDIA configured with the specified parameters. |
Component code
nvidia.py
404: Not Found
Ollama
This component generates text using Ollama’s language models.
For more information, see the Ollama documentation.
Parameters
Name | Display Name | Info |
---|---|---|
Base URL |
Base URL |
Endpoint of the Ollama API. |
Model Name |
Model Name |
The model name to use. |
Temperature |
Temperature |
Controls the creativity of model responses. |
Name | Type | Description |
---|---|---|
model |
LanguageModel |
An instance of an Ollama model configured with the specified parameters. |
Component code
ollama.py
404: Not Found
OpenAI
The OpenAIModelComponent generates text using OpenAI’s language models. It builds and returns a ChatOpenAI model instance with the specified configurations.
Parameters
Name | Display Name | Info |
---|---|---|
max_tokens |
Max Tokens |
Maximum number of tokens to generate |
model_kwargs |
Model Kwargs |
Additional keyword arguments for the model |
json_mode |
JSON Mode |
Enable JSON output mode |
output_schema |
Schema |
Schema for the model’s output |
model_name |
Model Name |
Name of the OpenAI model to use |
openai_api_base |
OpenAI API Base |
Base URL for the OpenAI API |
api_key |
OpenAI API Key |
API key for authentication |
temperature |
Temperature |
Controls randomness in output |
seed |
Seed |
Seed for reproducibility |
Name | Display Name | Info |
---|---|---|
output |
Language Model |
Configured ChatOpenAI model instance. |
Component code
openai.py
404: Not Found
OpenRouter
This component generates text using OpenRouter’s unified API for multiple AI models from different providers. For more information, see OpenRouter documentation.
Parameters
Name | Type | Description |
---|---|---|
api_key |
SecretString |
Your OpenRouter API key for authentication. |
site_url |
String |
Your site URL for OpenRouter rankings (advanced). |
app_name |
String |
Your app name for OpenRouter rankings (advanced). |
provider |
String |
The AI model provider to use. |
model_name |
String |
The specific model to use for chat completion. |
temperature |
Float |
Controls randomness in the output. Range: [0.0, 2.0]. Default: 0.7. |
max_tokens |
Integer |
The maximum number of tokens to generate (advanced). |
Name | Type | Description |
---|---|---|
model |
LanguageModel |
An instance of ChatOpenAI configured with the specified parameters. |
Component code
openrouter.py
404: Not Found
Perplexity
This component generates text using Perplexity’s language models.
For more information, see the Perplexity documentation.
Parameters
Name | Type | Description |
---|---|---|
model_name |
String |
The name of the Perplexity model to use. Options include various Llama 3.1 models. |
max_output_tokens |
Integer |
The maximum number of tokens to generate. |
api_key |
SecretString |
The Perplexity API Key for authentication. |
temperature |
Float |
Controls randomness in the output. Default: 0.75. |
top_p |
Float |
The maximum cumulative probability of tokens to consider when sampling (advanced). |
n |
Integer |
Number of chat completions to generate for each prompt (advanced). |
top_k |
Integer |
Number of top tokens to consider for top-k sampling. Must be positive (advanced). |
Name | Type | Description |
---|---|---|
model |
LanguageModel |
An instance of ChatPerplexity configured with the specified parameters. |
Component code
perplexity.py
404: Not Found
Qianfan
This component generates text using Qianfan’s language models.
For more information, see the Qianfan documentation.
Component code
baidu_qianfan_chat.py
404: Not Found
SambaNova
This component generates text using SambaNova LLMs.
For more information, see the Sambanova Cloud documentation.
Parameters
Name | Display Name | Info |
---|---|---|
sambanova_url |
SambaNova URL |
Base URL path for API requests. The default is |
sambanova_api_key |
SambaNova API Key |
Your SambaNova API Key. |
model_name |
Model Name |
The name of the Sambanova model to use. |
max_tokens |
Max Tokens |
The maximum number of tokens to generate. Set to |
temperature |
Temperature |
Controls randomness in the output. The default value is |
Name | Display Name | Info |
---|---|---|
model |
Model |
An instance of a SambaNova model configured with the specified parameters. |
Component code
sambanova.py
404: Not Found
VertexAI
This component generates text using Vertex AI LLMs.
For more information, see Google Vertex AI documentation.
Parameters
Name | Type | Description |
---|---|---|
credentials |
File |
JSON credentials file. Leave empty to fallback to environment variables. File type: JSON. |
model_name |
String |
The name of the Vertex AI model to use. Default: "gemini-1.5-pro". |
project |
String |
The project ID (advanced). |
location |
String |
The location for the Vertex AI API. Default: "us-central1" (advanced). |
max_output_tokens |
Integer |
The maximum number of tokens to generate (advanced). |
max_retries |
Integer |
Maximum number of retries for API calls. Default: 1 (advanced). |
temperature |
Float |
Controls randomness in the output. Default: 0.0. |
top_k |
Integer |
The number of highest probability vocabulary tokens to keep for top-k-filtering (advanced). |
top_p |
Float |
The cumulative probability of parameter highest probability vocabulary tokens to keep for nucleus sampling. Default: 0.95 (advanced). |
verbose |
Boolean |
Whether to print verbose output. Default: False (advanced). |
Name | Type | Description |
---|---|---|
model |
LanguageModel |
An instance of ChatVertexAI configured with the specified parameters. |
Component code
vertexai.py
404: Not Found
xAI
This component generates text using xAI models like Grok.
For more information, see the xAI documentation.
Parameters
Name | Type | Description |
---|---|---|
max_tokens |
Integer |
Maximum number of tokens to generate. Set to |
model_kwargs |
Dictionary |
Additional keyword arguments for the model. |
json_mode |
Boolean |
If |
model_name |
String |
The xAI model to use. Default: |
base_url |
String |
Base URL for API requests. Default: |
api_key |
SecretString |
Your xAI API key for authentication. |
temperature |
Float |
Controls randomness in the output. Range: |
seed |
Integer |
Controls reproducibility of the job. |
Name | Type | Description |
---|---|---|
model |
LanguageModel |
An instance of ChatOpenAI configured with the specified parameters. |
Component code
xai.py
404: Not Found