Prompts
This Langflow feature is currently in public preview. Development is ongoing, and the features and functionality are subject to change. Langflow, and the use of such, is subject to the DataStax Preview Terms. |
A prompt is a structured input to a language model that instructs the model how to handle user inputs and variables.
Prompt components create prompt templates with custom fields and dynamic variables for providing your model with structured, repeatable prompts.
Prompts are a combination of natural language and variables created with curly braces.
Use a prompt component in a flow
An example of modifying a prompt can be found in the Quickstart, where a basic chatbot flow is extended to include a full vector RAG pipeline.
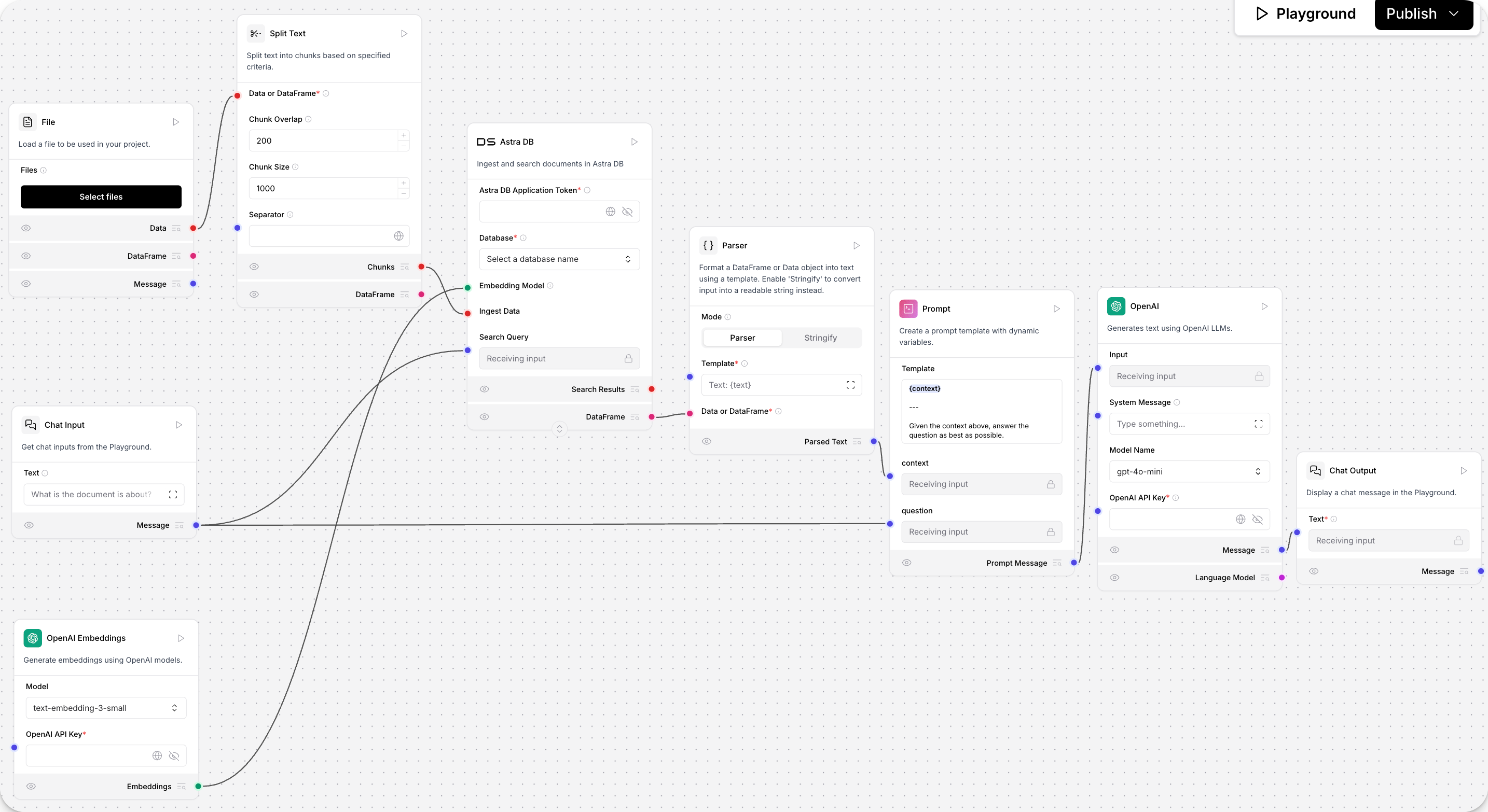
The default prompt in the Prompt component is Answer the user as if you were a GenAI expert, enthusiastic about helping them get started building something fresh.
This prompt creates a "personality" for your LLM’s chat interactions, but it doesn’t include variables that you may find useful when templating prompts.
To modify the prompt template, in the Prompt component, click the Template field. For example, the {context}
variable gives the LLM model access to embedded vector data to return better answers.
Given the context
{context}
Answer the question
{user_question}
When variables are added to a prompt template, new fields are automatically created in the component. These fields can be connected to receive text input from other components to automate prompting, or to output instructions to other components. An example of prompts controlling an agent’s behavior is available in the sequential tasks agent starter flow.
Prompt
This component creates a prompt template with dynamic variables. This is useful for structuring prompts and passing dynamic data to a language model.
Parameters
Name | Display Name | Info |
---|---|---|
template |
Template |
The prompt template with dynamic variables |
Name | Display Name | Info |
---|---|---|
prompt |
Prompt Message |
The built prompt message |
Prompt template field
The template
field allows you to create other fields dynamically by using curly brackets. For example, if you have a template like Hello {name}, how are you?
, a new field called name
is created. Prompt variables can be created with any name inside curly brackets, e.g. {variable_name}
.
Component code
prompt.py
from langflow.base.prompts.api_utils import process_prompt_template
from langflow.custom import Component
from langflow.inputs.inputs import DefaultPromptField
from langflow.io import MessageTextInput, Output, PromptInput
from langflow.schema.message import Message
from langflow.template.utils import update_template_values
class PromptComponent(Component):
display_name: str = "Prompt"
description: str = "Create a prompt template with dynamic variables."
icon = "prompts"
trace_type = "prompt"
name = "Prompt"
inputs = [
PromptInput(name="template", display_name="Template"),
MessageTextInput(
name="tool_placeholder",
display_name="Tool Placeholder",
tool_mode=True,
advanced=True,
info="A placeholder input for tool mode.",
),
]
outputs = [
Output(display_name="Prompt Message", name="prompt", method="build_prompt"),
]
async def build_prompt(self) -> Message:
prompt = Message.from_template(**self._attributes)
self.status = prompt.text
return prompt
def _update_template(self, frontend_node: dict):
prompt_template = frontend_node["template"]["template"]["value"]
custom_fields = frontend_node["custom_fields"]
frontend_node_template = frontend_node["template"]
_ = process_prompt_template(
template=prompt_template,
name="template",
custom_fields=custom_fields,
frontend_node_template=frontend_node_template,
)
return frontend_node
async def update_frontend_node(self, new_frontend_node: dict, current_frontend_node: dict):
"""This function is called after the code validation is done."""
frontend_node = await super().update_frontend_node(new_frontend_node, current_frontend_node)
template = frontend_node["template"]["template"]["value"]
# Kept it duplicated for backwards compatibility
_ = process_prompt_template(
template=template,
name="template",
custom_fields=frontend_node["custom_fields"],
frontend_node_template=frontend_node["template"],
)
# Now that template is updated, we need to grab any values that were set in the current_frontend_node
# and update the frontend_node with those values
update_template_values(new_template=frontend_node, previous_template=current_frontend_node["template"])
return frontend_node
def _get_fallback_input(self, **kwargs):
return DefaultPromptField(**kwargs)
Langchain Hub prompt
This component allows you to use prompts from LangChain Hub in your flow.
Parameters
Name | Type | Description |
---|---|---|
langchain_api_key |
SecretString |
Your LangChain API key for authentication |
langchain_hub_prompt |
String |
The LangChain Hub prompt to use (e.g., "efriis/my-first-prompt") |
Name | Type | Description |
---|---|---|
prompt |
Message |
The built prompt from LangChain Hub |
Component code
langchain_hub.py
import re
from langchain_core.prompts import HumanMessagePromptTemplate
from langflow.custom import Component
from langflow.inputs import DefaultPromptField, SecretStrInput, StrInput
from langflow.io import Output
from langflow.schema.message import Message
class LangChainHubPromptComponent(Component):
display_name: str = "Prompt Hub"
description: str = "Prompt Component that uses LangChain Hub prompts"
beta = True
icon = "LangChain"
trace_type = "prompt"
name = "LangChain Hub Prompt"
inputs = [
SecretStrInput(
name="langchain_api_key",
display_name="Your LangChain API Key",
info="The LangChain API Key to use.",
required=True,
),
StrInput(
name="langchain_hub_prompt",
display_name="LangChain Hub Prompt",
info="The LangChain Hub prompt to use, i.e., 'efriis/my-first-prompt'",
refresh_button=True,
required=True,
),
]
outputs = [
Output(display_name="Build Prompt", name="prompt", method="build_prompt"),
]
def update_build_config(self, build_config: dict, field_value: str, field_name: str | None = None):
# If the field is not langchain_hub_prompt or the value is empty, return the build config as is
if field_name != "langchain_hub_prompt" or not field_value:
return build_config
# Fetch the template
template = self._fetch_langchain_hub_template()
# Get the template's messages
if hasattr(template, "messages"):
template_messages = template.messages
else:
template_messages = [HumanMessagePromptTemplate(prompt=template)]
# Extract the messages from the prompt data
prompt_template = [message_data.prompt for message_data in template_messages]
# Regular expression to find all instances of {<string>}
pattern = r"\{(.*?)\}"
# Get all the custom fields
custom_fields: list[str] = []
full_template = ""
for message in prompt_template:
# Find all matches
matches = re.findall(pattern, message.template)
custom_fields += matches
# Create a string version of the full template
full_template = full_template + "\n" + message.template
# No need to reprocess if we have them already
if all("param_" + custom_field in build_config for custom_field in custom_fields):
return build_config
# Easter egg: Show template in info popup
build_config["langchain_hub_prompt"]["info"] = full_template
# Remove old parameter inputs if any
for key in build_config.copy():
if key.startswith("param_"):
del build_config[key]
# Now create inputs for each
for custom_field in custom_fields:
new_parameter = DefaultPromptField(
name=f"param_{custom_field}",
display_name=custom_field,
info="Fill in the value for {" + custom_field + "}",
).to_dict()
# Add the new parameter to the build config
build_config[f"param_{custom_field}"] = new_parameter
return build_config
async def build_prompt(
self,
) -> Message:
# Fetch the template
template = self._fetch_langchain_hub_template()
# Get the parameters from the attributes
params_dict = {param: getattr(self, "param_" + param, f"{{{param}}}") for param in template.input_variables}
original_params = {k: v.text if hasattr(v, "text") else v for k, v in params_dict.items() if v is not None}
prompt_value = template.invoke(original_params)
# Update the template with the new value
original_params["template"] = prompt_value.to_string()
# Now pass the filtered attributes to the function
prompt = Message.from_template(**original_params)
self.status = prompt.text
return prompt
def _fetch_langchain_hub_template(self):
import langchain.hub
# Check if the api key is provided
if not self.langchain_api_key:
msg = "Please provide a LangChain API Key"
raise ValueError(msg)
# Pull the prompt from LangChain Hub
return langchain.hub.pull(self.langchain_hub_prompt, api_key=self.langchain_api_key)