Tools
This Langflow feature is currently in public preview. Development is ongoing, and the features and functionality are subject to change. Langflow, and the use of such, is subject to the DataStax Preview Terms. |
Tools are typically connected to agent components at the Tools port. Agents use LLMs as a reasoning engine to decide which of the connected tool components to use to solve a problem.
Tools in agentic functions are, essentially, functions that the agent can call to perform tasks or access external resources.
A function is wrapped as a Tool
object, with a common interface the agent understands.
Agents become aware of tools through tool registration, where the agent is provided a list of available tools, typically at agent initialization. The Tool
object’s description tells the agent what the tool can do.
The agent then uses a connected LLM to reason through the problem to decide which tool is best for the job.
Use a tool in a flow
Tools are typically connected to agent components at the Tools port.
The simple agent starter project uses URL and Calculator tools connected to an agent component to answer a user’s questions. The OpenAI LLM acts as a brain for the agent to decide which tool to use.
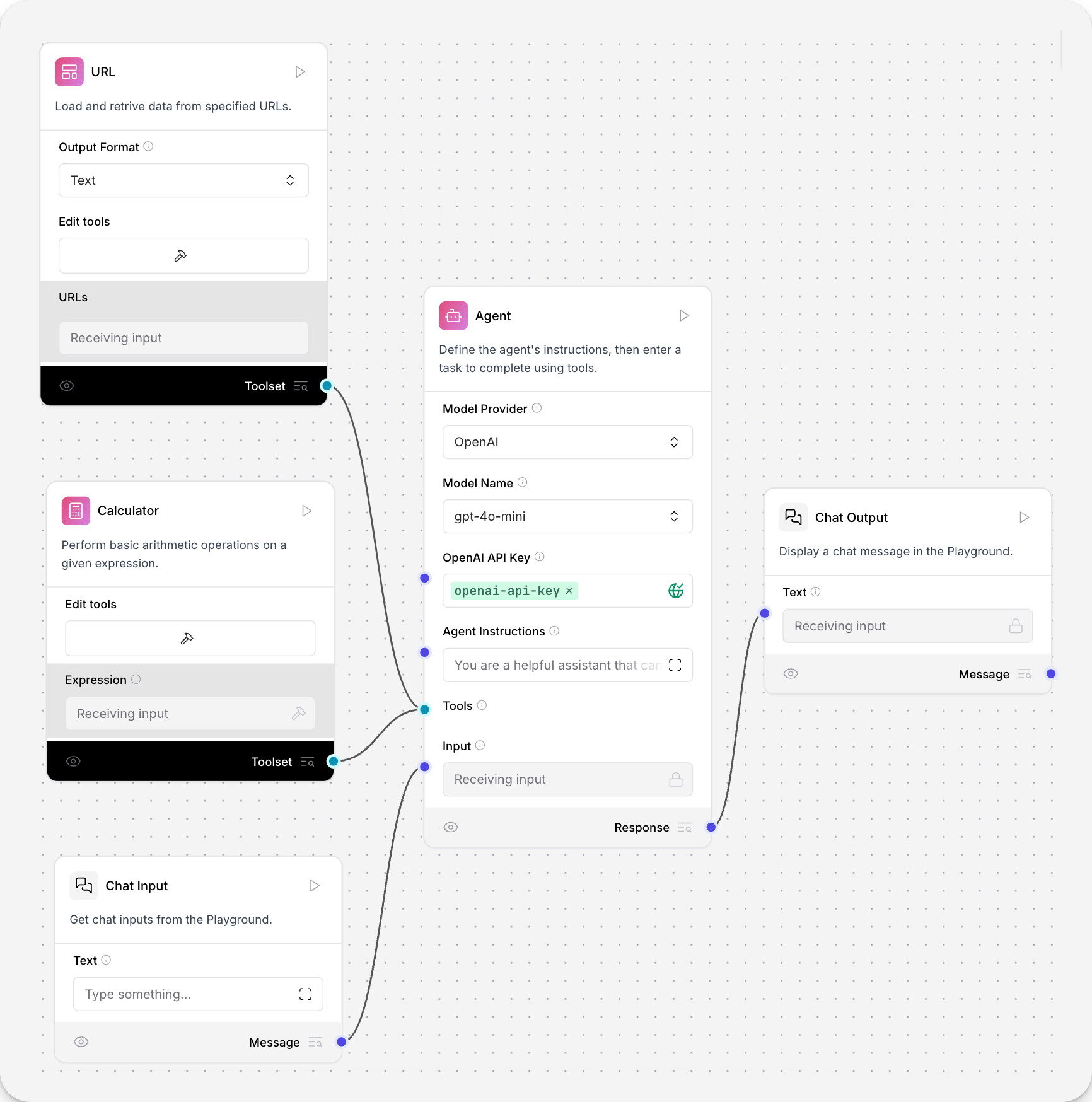
To make a component into a tool that an agent can use, enable Tool mode in the component. Enabling Tool mode modifies a component input to accept calls from an agent. If the component you want to connect to an agent doesn’t have a Tool mode option, you can modify the component’s inputs to become a tool. For an example, see Make any component a tool.
arXiv
This component searches and retrieves papers from arXiv.org.
Parameters
Name | Display Name | Info |
---|---|---|
search_query |
Search Query |
The search query for arXiv papers, for example, 'quantum computing'. |
search_type |
Search Field |
The field to search in. |
max_results |
Max Results |
Maximum number of results to return. |
Name | Display Name | Info |
---|---|---|
papers |
Papers |
List of retrieved arXiv papers. |
Component code
arxiv.py
import urllib.request
from urllib.parse import urlparse
from xml.etree.ElementTree import Element
from defusedxml.ElementTree import fromstring
from langflow.custom import Component
from langflow.io import DropdownInput, IntInput, MessageTextInput, Output
from langflow.schema import Data
class ArXivComponent(Component):
display_name = "arXiv"
description = "Search and retrieve papers from arXiv.org"
icon = "arXiv"
inputs = [
MessageTextInput(
name="search_query",
display_name="Search Query",
info="The search query for arXiv papers (e.g., 'quantum computing')",
tool_mode=True,
),
DropdownInput(
name="search_type",
display_name="Search Field",
info="The field to search in",
options=["all", "title", "abstract", "author", "cat"], # cat is for category
value="all",
),
IntInput(
name="max_results",
display_name="Max Results",
info="Maximum number of results to return",
value=10,
),
]
outputs = [
Output(display_name="Papers", name="papers", method="search_papers"),
]
def build_query_url(self) -> str:
"""Build the arXiv API query URL."""
base_url = "http://export.arxiv.org/api/query?"
# Build the search query
search_query = f"{self.search_type}:{self.search_query}"
# URL parameters
params = {
"search_query": search_query,
"max_results": str(self.max_results),
}
# Convert params to URL query string
query_string = "&".join([f"{k}={urllib.parse.quote(str(v))}" for k, v in params.items()])
return base_url + query_string
def parse_atom_response(self, response_text: str) -> list[dict]:
"""Parse the Atom XML response from arXiv."""
# Parse XML safely using defusedxml
root = fromstring(response_text)
# Define namespace dictionary for XML parsing
ns = {"atom": "http://www.w3.org/2005/Atom", "arxiv": "http://arxiv.org/schemas/atom"}
papers = []
# Process each entry (paper)
for entry in root.findall("atom:entry", ns):
paper = {
"id": self._get_text(entry, "atom:id", ns),
"title": self._get_text(entry, "atom:title", ns),
"summary": self._get_text(entry, "atom:summary", ns),
"published": self._get_text(entry, "atom:published", ns),
"updated": self._get_text(entry, "atom:updated", ns),
"authors": [author.find("atom:name", ns).text for author in entry.findall("atom:author", ns)],
"arxiv_url": self._get_link(entry, "alternate", ns),
"pdf_url": self._get_link(entry, "related", ns),
"comment": self._get_text(entry, "arxiv:comment", ns),
"journal_ref": self._get_text(entry, "arxiv:journal_ref", ns),
"primary_category": self._get_category(entry, ns),
"categories": [cat.get("term") for cat in entry.findall("atom:category", ns)],
}
papers.append(paper)
return papers
def _get_text(self, element: Element, path: str, ns: dict) -> str | None:
"""Safely extract text from an XML element."""
el = element.find(path, ns)
return el.text.strip() if el is not None and el.text else None
def _get_link(self, element: Element, rel: str, ns: dict) -> str | None:
"""Get link URL based on relation type."""
for link in element.findall("atom:link", ns):
if link.get("rel") == rel:
return link.get("href")
return None
def _get_category(self, element: Element, ns: dict) -> str | None:
"""Get primary category."""
cat = element.find("arxiv:primary_category", ns)
return cat.get("term") if cat is not None else None
def search_papers(self) -> list[Data]:
"""Search arXiv and return results."""
try:
# Build the query URL
url = self.build_query_url()
# Validate URL scheme and host
parsed_url = urlparse(url)
if parsed_url.scheme not in ("http", "https"):
error_msg = f"Invalid URL scheme: {parsed_url.scheme}"
raise ValueError(error_msg)
if parsed_url.hostname != "export.arxiv.org":
error_msg = f"Invalid host: {parsed_url.hostname}"
raise ValueError(error_msg)
# Create a custom opener that only allows http/https schemes
class RestrictedHTTPHandler(urllib.request.HTTPHandler):
def http_open(self, req):
return super().http_open(req)
class RestrictedHTTPSHandler(urllib.request.HTTPSHandler):
def https_open(self, req):
return super().https_open(req)
# Build opener with restricted handlers
opener = urllib.request.build_opener(RestrictedHTTPHandler, RestrictedHTTPSHandler)
urllib.request.install_opener(opener)
# Make the request with validated URL using restricted opener
response = opener.open(url)
response_text = response.read().decode("utf-8")
# Parse the response
papers = self.parse_atom_response(response_text)
# Convert to Data objects
results = [Data(data=paper) for paper in papers]
self.status = results
except (urllib.error.URLError, ValueError) as e:
error_data = Data(data={"error": f"Request error: {e!s}"})
self.status = error_data
return [error_data]
else:
return results
Astra DB tool
This component creates a tool an Agent can use to retrieve data from a DataStax Astra DB collection.
Parameters
Name | Type | Description |
---|---|---|
tool_name |
String |
The name of the tool |
tool_description |
String |
The description of the tool |
namespace |
String |
The namespace within Astra where the collection is stored (default: "default_keyspace") |
collection_name |
String |
The name of the collection within Astra DB |
token |
SecretString |
Authentication token for accessing Astra DB |
api_endpoint |
String |
API endpoint URL for the Astra DB service |
projection_attributes |
String |
Attributes to return, separated by commas (default: "*") |
tool_params |
List[Dict] |
Attributes to filter and description for the model |
static_filters |
List[Dict] |
Attributes to filter and corresponding values |
number_of_results |
Integer |
Number of results to return (default: 5) |
Name | Type | Description |
---|---|---|
tool |
StructuredTool |
Astra DB search tool for use in LangChain |
Component code
astradb.py
import os
from typing import Any
from astrapy import Collection, DataAPIClient, Database
from langchain.pydantic_v1 import BaseModel, Field, create_model
from langchain_core.tools import StructuredTool, Tool
from langflow.base.langchain_utilities.model import LCToolComponent
from langflow.io import DictInput, IntInput, SecretStrInput, StrInput
from langflow.schema import Data
class AstraDBToolComponent(LCToolComponent):
display_name: str = "Astra DB Tool"
description: str = "Create a tool to get transactional data from DataStax Astra DB Collection"
documentation: str = "https://docs.langflow.org/Components/components-tools#astra-db-tool"
icon: str = "AstraDB"
inputs = [
StrInput(
name="tool_name",
display_name="Tool Name",
info="The name of the tool.",
required=True,
),
StrInput(
name="tool_description",
display_name="Tool Description",
info="The description of the tool.",
required=True,
),
StrInput(
name="namespace",
display_name="Namespace Name",
info="The name of the namespace within Astra where the collection is be stored.",
value="default_keyspace",
advanced=True,
),
StrInput(
name="collection_name",
display_name="Collection Name",
info="The name of the collection within Astra DB where the vectors will be stored.",
required=True,
),
SecretStrInput(
name="token",
display_name="Astra DB Application Token",
info="Authentication token for accessing Astra DB.",
value="ASTRA_DB_APPLICATION_TOKEN",
required=True,
),
SecretStrInput(
name="api_endpoint",
display_name="Database" if os.getenv("ASTRA_ENHANCED", "false").lower() == "true" else "API Endpoint",
info="API endpoint URL for the Astra DB service.",
value="ASTRA_DB_API_ENDPOINT",
required=True,
),
StrInput(
name="projection_attributes",
display_name="Projection Attributes",
info="Attributes to return separated by comma.",
required=True,
value="*",
advanced=True,
),
DictInput(
name="tool_params",
info="Attributes to filter and description to the model. Add ! for mandatory (e.g: !customerId)",
display_name="Tool params",
is_list=True,
),
DictInput(
name="static_filters",
info="Attributes to filter and correspoding value",
display_name="Static filters",
advanced=True,
is_list=True,
),
IntInput(
name="number_of_results",
display_name="Number of Results",
info="Number of results to return.",
advanced=True,
value=5,
),
]
_cached_client: DataAPIClient | None = None
_cached_db: Database | None = None
_cached_collection: Collection | None = None
def _build_collection(self):
if self._cached_collection:
return self._cached_collection
cached_client = DataAPIClient(self.token)
cached_db = cached_client.get_database(self.api_endpoint, namespace=self.namespace)
self._cached_collection = cached_db.get_collection(self.collection_name)
return self._cached_collection
def create_args_schema(self) -> dict[str, BaseModel]:
args: dict[str, tuple[Any, Field] | list[str]] = {}
for key in self.tool_params:
if key.startswith("!"): # Mandatory
args[key[1:]] = (str, Field(description=self.tool_params[key]))
else: # Optional
args[key] = (str | None, Field(description=self.tool_params[key], default=None))
model = create_model("ToolInput", **args, __base__=BaseModel)
return {"ToolInput": model}
def build_tool(self) -> Tool:
"""Builds an Astra DB Collection tool.
Returns:
Tool: The built Astra DB tool.
"""
schema_dict = self.create_args_schema()
tool = StructuredTool.from_function(
name=self.tool_name,
args_schema=schema_dict["ToolInput"],
description=self.tool_description,
func=self.run_model,
return_direct=False,
)
self.status = "Astra DB Tool created"
return tool
def projection_args(self, input_str: str) -> dict:
elements = input_str.split(",")
result = {}
for element in elements:
if element.startswith("!"):
result[element[1:]] = False
else:
result[element] = True
return result
def run_model(self, **args) -> Data | list[Data]:
collection = self._build_collection()
results = collection.find(
({**args, **self.static_filters}),
projection=self.projection_args(self.projection_attributes),
limit=self.number_of_results,
)
data: list[Data] = [Data(data=doc) for doc in results]
self.status = data
return data
Astra DB CQL tool
This component creates a tool an Agent can use to retrieve data from a DataStax Astra DB CQL table.
Parameters
Name | Type | Description |
---|---|---|
tool_name |
String |
The name of the tool |
tool_description |
String |
The tool description to be passed to the model |
keyspace |
String |
The keyspace name within Astra DB where the data is stored |
table_name |
String |
The name of the table within Astra DB where the data is stored |
token |
SecretString |
Authentication token for accessing Astra DB |
api_endpoint |
String |
API endpoint URL for the Astra DB service |
projection_fields |
String |
Attributes to return, separated by commas (default: "*") |
partition_keys |
List[Dict] |
Field name and description for partition keys |
clustering_keys |
List[Dict] |
Field name and description for clustering keys |
static_filters |
List[Dict] |
Field name and value for static filters |
number_of_results |
Integer |
Number of results to return (default: 5) |
Name | Type | Description |
---|---|---|
tool |
StructuredTool |
Astra DB CQL search tool for use in LangChain |
Component code
astradb_cql.py
import urllib
from http import HTTPStatus
from typing import Any
import requests
from langchain.pydantic_v1 import BaseModel, Field, create_model
from langchain_core.tools import StructuredTool, Tool
from langflow.base.langchain_utilities.model import LCToolComponent
from langflow.io import DictInput, IntInput, SecretStrInput, StrInput
from langflow.schema import Data
class AstraDBCQLToolComponent(LCToolComponent):
display_name: str = "Astra DB CQL"
description: str = "Create a tool to get transactional data from DataStax Astra DB CQL Table"
documentation: str = "https://docs.langflow.org/Components/components-tools#astra-db-cql-tool"
icon: str = "AstraDB"
inputs = [
StrInput(name="tool_name", display_name="Tool Name", info="The name of the tool.", required=True),
StrInput(
name="tool_description",
display_name="Tool Description",
info="The tool description to be passed to the model.",
required=True,
),
StrInput(
name="keyspace",
display_name="Keyspace",
value="default_keyspace",
info="The keyspace name within Astra DB where the data is stored.",
required=True,
advanced=True,
),
StrInput(
name="table_name",
display_name="Table Name",
info="The name of the table within Astra DB where the data is stored.",
required=True,
),
SecretStrInput(
name="token",
display_name="Astra DB Application Token",
info="Authentication token for accessing Astra DB.",
value="ASTRA_DB_APPLICATION_TOKEN",
required=True,
),
StrInput(
name="api_endpoint",
display_name="API Endpoint",
info="API endpoint URL for the Astra DB service.",
value="ASTRA_DB_API_ENDPOINT",
required=True,
),
StrInput(
name="projection_fields",
display_name="Projection fields",
info="Attributes to return separated by comma.",
required=True,
value="*",
advanced=True,
),
DictInput(
name="partition_keys",
display_name="Partition Keys",
is_list=True,
info="Field name and description to the model",
required=True,
),
DictInput(
name="clustering_keys",
display_name="Clustering Keys",
is_list=True,
info="Field name and description to the model",
),
DictInput(
name="static_filters",
display_name="Static Filters",
is_list=True,
advanced=True,
info="Field name and value. When filled, it will not be generated by the LLM.",
),
IntInput(
name="number_of_results",
display_name="Number of Results",
info="Number of results to return.",
advanced=True,
value=5,
),
]
def astra_rest(self, args):
headers = {"Accept": "application/json", "X-Cassandra-Token": f"{self.token}"}
astra_url = f"{self.api_endpoint}/api/rest/v2/keyspaces/{self.keyspace}/{self.table_name}/"
key = []
# Partition keys are mandatory
key = [self.partition_keys[k] for k in self.partition_keys]
# Clustering keys are optional
for k in self.clustering_keys:
if k in args:
key.append(args[k])
elif self.static_filters[k] is not None:
key.append(self.static_filters[k])
url = f"{astra_url}{'/'.join(key)}?page-size={self.number_of_results}"
if self.projection_fields != "*":
url += f"&fields={urllib.parse.quote(self.projection_fields.replace(' ', ''))}"
res = requests.request("GET", url=url, headers=headers, timeout=10)
if int(res.status_code) >= HTTPStatus.BAD_REQUEST:
return res.text
try:
res_data = res.json()
return res_data["data"]
except ValueError:
return res.status_code
def create_args_schema(self) -> dict[str, BaseModel]:
args: dict[str, tuple[Any, Field]] = {}
for key in self.partition_keys:
# Partition keys are mandatory is it doesn't have a static filter
if key not in self.static_filters:
args[key] = (str, Field(description=self.partition_keys[key]))
for key in self.clustering_keys:
# Partition keys are mandatory if has the exclamation mark and doesn't have a static filter
if key not in self.static_filters:
if key.startswith("!"): # Mandatory
args[key[1:]] = (str, Field(description=self.clustering_keys[key]))
else: # Optional
args[key] = (str | None, Field(description=self.clustering_keys[key], default=None))
model = create_model("ToolInput", **args, __base__=BaseModel)
return {"ToolInput": model}
def build_tool(self) -> Tool:
"""Builds a Astra DB CQL Table tool.
Args:
name (str, optional): The name of the tool.
Returns:
Tool: The built AstraDB tool.
"""
schema_dict = self.create_args_schema()
return StructuredTool.from_function(
name=self.tool_name,
args_schema=schema_dict["ToolInput"],
description=self.tool_description,
func=self.run_model,
return_direct=False,
)
def projection_args(self, input_str: str) -> dict:
elements = input_str.split(",")
result = {}
for element in elements:
if element.startswith("!"):
result[element[1:]] = False
else:
result[element] = True
return result
def run_model(self, **args) -> Data | list[Data]:
results = self.astra_rest(args)
data: list[Data] = [Data(data=doc) for doc in results]
self.status = data
return results
Bing Search API
This component allows you to call the Bing Search API.
Parameters
Name | Type | Description |
---|---|---|
bing_subscription_key |
SecretString |
Bing API subscription key |
input_value |
String |
Search query input |
bing_search_url |
String |
Custom Bing Search URL (optional) |
k |
Integer |
Number of search results to return |
Name | Type | Description |
---|---|---|
results |
List[Data] |
List of search results |
tool |
Tool |
Bing Search tool for use in LangChain |
Component code
bing_search_api.py
from typing import cast
from langchain_community.tools.bing_search import BingSearchResults
from langchain_community.utilities import BingSearchAPIWrapper
from langflow.base.langchain_utilities.model import LCToolComponent
from langflow.field_typing import Tool
from langflow.inputs import IntInput, MessageTextInput, MultilineInput, SecretStrInput
from langflow.schema import Data
class BingSearchAPIComponent(LCToolComponent):
display_name = "Bing Search API"
description = "Call the Bing Search API."
name = "BingSearchAPI"
icon = "Bing"
inputs = [
SecretStrInput(name="bing_subscription_key", display_name="Bing Subscription Key"),
MultilineInput(
name="input_value",
display_name="Input",
),
MessageTextInput(name="bing_search_url", display_name="Bing Search URL", advanced=True),
IntInput(name="k", display_name="Number of results", value=4, required=True),
]
def run_model(self) -> list[Data]:
if self.bing_search_url:
wrapper = BingSearchAPIWrapper(
bing_search_url=self.bing_search_url, bing_subscription_key=self.bing_subscription_key
)
else:
wrapper = BingSearchAPIWrapper(bing_subscription_key=self.bing_subscription_key)
results = wrapper.results(query=self.input_value, num_results=self.k)
data = [Data(data=result, text=result["snippet"]) for result in results]
self.status = data
return data
def build_tool(self) -> Tool:
if self.bing_search_url:
wrapper = BingSearchAPIWrapper(
bing_search_url=self.bing_search_url, bing_subscription_key=self.bing_subscription_key
)
else:
wrapper = BingSearchAPIWrapper(bing_subscription_key=self.bing_subscription_key)
return cast("Tool", BingSearchResults(api_wrapper=wrapper, num_results=self.k))
Calculator Tool
This component allows you to evaluate basic arithmetic expressions. It supports addition, subtraction, multiplication, division, and exponentiation. The tool uses a secure evaluation method that prevents the execution of arbitrary Python code.
Parameters
Name | Type | Description |
---|---|---|
expression |
String |
The arithmetic expression to evaluate (for example, 4*4*(33/22)+12-20). |
Name | Type | Description |
---|---|---|
result |
Tool |
Calculator tool for use in LangChain. |
Component code
calculator.py
import ast
import operator
from langchain.tools import StructuredTool
from langchain_core.tools import ToolException
from loguru import logger
from pydantic import BaseModel, Field
from langflow.base.langchain_utilities.model import LCToolComponent
from langflow.field_typing import Tool
from langflow.inputs import MessageTextInput
from langflow.schema import Data
class CalculatorToolComponent(LCToolComponent):
display_name = "Calculator [DEPRECATED]"
description = "Perform basic arithmetic operations on a given expression."
icon = "calculator"
name = "CalculatorTool"
legacy = True
inputs = [
MessageTextInput(
name="expression",
display_name="Expression",
info="The arithmetic expression to evaluate (e.g., '4*4*(33/22)+12-20').",
),
]
class CalculatorToolSchema(BaseModel):
expression: str = Field(..., description="The arithmetic expression to evaluate.")
def run_model(self) -> list[Data]:
return self._evaluate_expression(self.expression)
def build_tool(self) -> Tool:
return StructuredTool.from_function(
name="calculator",
description="Evaluate basic arithmetic expressions. Input should be a string containing the expression.",
func=self._eval_expr_with_error,
args_schema=self.CalculatorToolSchema,
)
def _eval_expr(self, node):
if isinstance(node, ast.Num):
return node.n
if isinstance(node, ast.BinOp):
left_val = self._eval_expr(node.left)
right_val = self._eval_expr(node.right)
return self.operators[type(node.op)](left_val, right_val)
if isinstance(node, ast.UnaryOp):
operand_val = self._eval_expr(node.operand)
return self.operators[type(node.op)](operand_val)
if isinstance(node, ast.Call):
msg = (
"Function calls like sqrt(), sin(), cos() etc. are not supported. "
"Only basic arithmetic operations (+, -, *, /, **) are allowed."
)
raise TypeError(msg)
msg = f"Unsupported operation or expression type: {type(node).__name__}"
raise TypeError(msg)
def _eval_expr_with_error(self, expression: str) -> list[Data]:
try:
return self._evaluate_expression(expression)
except Exception as e:
raise ToolException(str(e)) from e
def _evaluate_expression(self, expression: str) -> list[Data]:
try:
# Parse the expression and evaluate it
tree = ast.parse(expression, mode="eval")
result = self._eval_expr(tree.body)
# Format the result to a reasonable number of decimal places
formatted_result = f"{result:.6f}".rstrip("0").rstrip(".")
self.status = formatted_result
return [Data(data={"result": formatted_result})]
except (SyntaxError, TypeError, KeyError) as e:
error_message = f"Invalid expression: {e}"
self.status = error_message
return [Data(data={"error": error_message, "input": expression})]
except ZeroDivisionError:
error_message = "Error: Division by zero"
self.status = error_message
return [Data(data={"error": error_message, "input": expression})]
except Exception as e: # noqa: BLE001
logger.opt(exception=True).debug("Error evaluating expression")
error_message = f"Error: {e}"
self.status = error_message
return [Data(data={"error": error_message, "input": expression})]
def __init__(self, *args, **kwargs):
super().__init__(*args, **kwargs)
self.operators = {
ast.Add: operator.add,
ast.Sub: operator.sub,
ast.Mult: operator.mul,
ast.Div: operator.truediv,
ast.Pow: operator.pow,
}
DuckDuckGo search
This component performs web searches using the DuckDuckGo search engine with result-limiting capabilities.
Parameters
Name | Display Name | Info |
---|---|---|
input_value |
Search Query |
The search query to be used for the DuckDuckGo search. |
max_results |
Max Results |
Maximum number of results to return. |
max_snippet_length |
Max Snippet Length |
Maximum length of each result snippet. |
Name | Display Name | Info |
---|---|---|
data |
Data |
List of search results as Data objects. |
Component code
duck_duck_go_search_run.py
from langchain_community.tools import DuckDuckGoSearchRun
from langflow.custom import Component
from langflow.inputs import IntInput, MessageTextInput
from langflow.io import Output
from langflow.schema import Data
from langflow.schema.message import Message
class DuckDuckGoSearchComponent(Component):
"""Component for performing web searches using DuckDuckGo."""
display_name = "DuckDuckGo Search"
description = "Search the web using DuckDuckGo with customizable result limits"
documentation = "https://python.langchain.com/docs/integrations/tools/ddg"
icon = "DuckDuckGo"
inputs = [
MessageTextInput(
name="input_value",
display_name="Search Query",
required=True,
info="The search query to execute with DuckDuckGo",
tool_mode=True,
),
IntInput(
name="max_results",
display_name="Max Results",
value=5,
required=False,
advanced=True,
info="Maximum number of search results to return",
),
IntInput(
name="max_snippet_length",
display_name="Max Snippet Length",
value=100,
required=False,
advanced=True,
info="Maximum length of each result snippet",
),
]
outputs = [
Output(display_name="Data", name="data", method="fetch_content"),
Output(display_name="Text", name="text", method="fetch_content_text"),
]
def _build_wrapper(self) -> DuckDuckGoSearchRun:
"""Build the DuckDuckGo search wrapper."""
return DuckDuckGoSearchRun()
def run_model(self) -> list[Data]:
return self.fetch_content()
def fetch_content(self) -> list[Data]:
"""Execute the search and return results as Data objects."""
try:
wrapper = self._build_wrapper()
full_results = wrapper.run(f"{self.input_value} (site:*)")
result_list = full_results.split("\n")[: self.max_results]
data_results = []
for result in result_list:
if result.strip():
snippet = result[: self.max_snippet_length]
data_results.append(
Data(
text=snippet,
data={
"content": result,
"snippet": snippet,
},
)
)
except (ValueError, AttributeError) as e:
error_data = [Data(text=str(e), data={"error": str(e)})]
self.status = error_data
return error_data
else:
self.status = data_results
return data_results
def fetch_content_text(self) -> Message:
"""Return search results as a single text message."""
data = self.fetch_content()
result_string = "\n".join(item.text for item in data)
self.status = result_string
return Message(text=result_string)
Exa Search
This component provides an Exa Search toolkit for search and content retrieval.
Parameters
Name | Display Name | Info |
---|---|---|
metaphor_api_key |
Exa Search API Key |
API key for Exa Search, entered as a password. |
use_autoprompt |
Use Autoprompt |
Whether to use the autoprompt feature. (default: true) |
search_num_results |
Search Number of Results |
Number of results to return for search. (default: 5) |
similar_num_results |
Similar Number of Results |
Number of similar results to return. (default: 5) |
Name | Display Name | Info |
---|---|---|
tools |
Tools |
List of search tools provided by the toolkit. |
Component code
exa_search.py
from langchain_core.tools import tool
from metaphor_python import Metaphor
from langflow.custom import Component
from langflow.field_typing import Tool
from langflow.io import BoolInput, IntInput, Output, SecretStrInput
class ExaSearchToolkit(Component):
display_name = "Exa Search"
description = "Exa Search toolkit for search and content retrieval"
documentation = "https://python.langchain.com/docs/integrations/tools/metaphor_search"
beta = True
name = "ExaSearch"
icon = "ExaSearch"
inputs = [
SecretStrInput(
name="metaphor_api_key",
display_name="Exa Search API Key",
password=True,
),
BoolInput(
name="use_autoprompt",
display_name="Use Autoprompt",
value=True,
),
IntInput(
name="search_num_results",
display_name="Search Number of Results",
value=5,
),
IntInput(
name="similar_num_results",
display_name="Similar Number of Results",
value=5,
),
]
outputs = [
Output(name="tools", display_name="Tools", method="build_toolkit"),
]
def build_toolkit(self) -> Tool:
client = Metaphor(api_key=self.metaphor_api_key)
@tool
def search(query: str):
"""Call search engine with a query."""
return client.search(query, use_autoprompt=self.use_autoprompt, num_results=self.search_num_results)
@tool
def get_contents(ids: list[str]):
"""Get contents of a webpage.
The ids passed in should be a list of ids as fetched from `search`.
"""
return client.get_contents(ids)
@tool
def find_similar(url: str):
"""Get search results similar to a given URL.
The url passed in should be a URL returned from `search`
"""
return client.find_similar(url, num_results=self.similar_num_results)
return [search, get_contents, find_similar]
Glean Search API
This component allows you to call the Glean Search API.
Parameters
Name | Type | Description |
---|---|---|
glean_api_url |
String |
URL of the Glean API |
glean_access_token |
SecretString |
Access token for Glean API authentication |
query |
String |
Search query input |
page_size |
Integer |
Number of results per page (default: 10) |
request_options |
Dict |
Additional options for the API request (optional) |
Name | Type | Description |
---|---|---|
results |
List[Data] |
List of search results |
tool |
Tool |
Glean Search tool for use in LangChain |
Component code
glean_search_api.py
import json
from typing import Any
from urllib.parse import urljoin
import httpx
from langchain_core.tools import StructuredTool, ToolException
from pydantic import BaseModel
from pydantic.v1 import Field
from langflow.base.langchain_utilities.model import LCToolComponent
from langflow.field_typing import Tool
from langflow.inputs import IntInput, MultilineInput, NestedDictInput, SecretStrInput, StrInput
from langflow.schema import Data
class GleanSearchAPISchema(BaseModel):
query: str = Field(..., description="The search query")
page_size: int = Field(10, description="Maximum number of results to return")
request_options: dict[str, Any] | None = Field(default_factory=dict, description="Request Options")
class GleanAPIWrapper(BaseModel):
"""Wrapper around Glean API."""
glean_api_url: str
glean_access_token: str
act_as: str = "langflow-component@datastax.com" # TODO: Detect this
def _prepare_request(
self,
query: str,
page_size: int = 10,
request_options: dict[str, Any] | None = None,
) -> dict:
# Ensure there's a trailing slash
url = self.glean_api_url
if not url.endswith("/"):
url += "/"
return {
"url": urljoin(url, "search"),
"headers": {
"Authorization": f"Bearer {self.glean_access_token}",
"X-Scio-ActAs": self.act_as,
},
"payload": {
"query": query,
"pageSize": page_size,
"requestOptions": request_options,
},
}
def results(self, query: str, **kwargs: Any) -> list[dict[str, Any]]:
results = self._search_api_results(query, **kwargs)
if len(results) == 0:
msg = "No good Glean Search Result was found"
raise AssertionError(msg)
return results
def run(self, query: str, **kwargs: Any) -> list[dict[str, Any]]:
try:
results = self.results(query, **kwargs)
processed_results = []
for result in results:
if "title" in result:
result["snippets"] = result.get("snippets", [{"snippet": {"text": result["title"]}}])
if "text" not in result["snippets"][0]:
result["snippets"][0]["text"] = result["title"]
processed_results.append(result)
except Exception as e:
error_message = f"Error in Glean Search API: {e!s}"
raise ToolException(error_message) from e
return processed_results
def _search_api_results(self, query: str, **kwargs: Any) -> list[dict[str, Any]]:
request_details = self._prepare_request(query, **kwargs)
response = httpx.post(
request_details["url"],
json=request_details["payload"],
headers=request_details["headers"],
)
response.raise_for_status()
response_json = response.json()
return response_json.get("results", [])
@staticmethod
def _result_as_string(result: dict) -> str:
return json.dumps(result, indent=4)
class GleanSearchAPIComponent(LCToolComponent):
display_name = "Glean Search API"
description = "Call Glean Search API"
name = "GleanAPI"
icon = "Glean"
inputs = [
StrInput(
name="glean_api_url",
display_name="Glean API URL",
required=True,
),
SecretStrInput(name="glean_access_token", display_name="Glean Access Token", required=True),
MultilineInput(name="query", display_name="Query", required=True),
IntInput(name="page_size", display_name="Page Size", value=10),
NestedDictInput(name="request_options", display_name="Request Options", required=False),
]
def build_tool(self) -> Tool:
wrapper = self._build_wrapper(
glean_api_url=self.glean_api_url,
glean_access_token=self.glean_access_token,
)
tool = StructuredTool.from_function(
name="glean_search_api",
description="Search Glean for relevant results.",
func=wrapper.run,
args_schema=GleanSearchAPISchema,
)
self.status = "Glean Search API Tool for Langchain"
return tool
def run_model(self) -> list[Data]:
tool = self.build_tool()
results = tool.run(
{
"query": self.query,
"page_size": self.page_size,
"request_options": self.request_options,
}
)
# Build the data
data = [Data(data=result, text=result["snippets"][0]["text"]) for result in results]
self.status = data # type: ignore[assignment]
return data
def _build_wrapper(
self,
glean_api_url: str,
glean_access_token: str,
):
return GleanAPIWrapper(
glean_api_url=glean_api_url,
glean_access_token=glean_access_token,
)
Google search API
This component allows you to call the Google Search API.
Parameters
Name | Type | Description |
---|---|---|
google_api_key |
SecretString |
Google API key for authentication |
google_cse_id |
SecretString |
Google Custom Search Engine ID |
input_value |
String |
Search query input |
k |
Integer |
Number of search results to return |
Name | Type | Description |
---|---|---|
results |
List[Data] |
List of search results |
tool |
Tool |
Google Search tool for use in LangChain |
Component code
google_search_api.py
from langchain_core.tools import Tool
from langflow.base.langchain_utilities.model import LCToolComponent
from langflow.inputs import IntInput, MultilineInput, SecretStrInput
from langflow.schema import Data
class GoogleSearchAPIComponent(LCToolComponent):
display_name = "Google Search API [DEPRECATED]"
description = "Call Google Search API."
name = "GoogleSearchAPI"
icon = "Google"
legacy = True
inputs = [
SecretStrInput(name="google_api_key", display_name="Google API Key", required=True),
SecretStrInput(name="google_cse_id", display_name="Google CSE ID", required=True),
MultilineInput(
name="input_value",
display_name="Input",
),
IntInput(name="k", display_name="Number of results", value=4, required=True),
]
def run_model(self) -> Data | list[Data]:
wrapper = self._build_wrapper()
results = wrapper.results(query=self.input_value, num_results=self.k)
data = [Data(data=result, text=result["snippet"]) for result in results]
self.status = data
return data
def build_tool(self) -> Tool:
wrapper = self._build_wrapper()
return Tool(
name="google_search",
description="Search Google for recent results.",
func=wrapper.run,
)
def _build_wrapper(self):
try:
from langchain_google_community import GoogleSearchAPIWrapper
except ImportError as e:
msg = "Please install langchain-google-community to use GoogleSearchAPIWrapper."
raise ImportError(msg) from e
return GoogleSearchAPIWrapper(google_api_key=self.google_api_key, google_cse_id=self.google_cse_id, k=self.k)
Google serper API
This component allows you to call the Serper.dev Google Search API.
Parameters
Name | Type | Description |
---|---|---|
serper_api_key |
SecretString |
API key for Serper.dev authentication |
input_value |
String |
Search query input |
k |
Integer |
Number of search results to return |
Name | Type | Description |
---|---|---|
results |
List[Data] |
List of search results |
tool |
Tool |
Google Serper search tool for use in LangChain |
Component code
google_serper_api.py
from typing import Any
from langchain.tools import StructuredTool
from langchain_community.utilities.google_serper import GoogleSerperAPIWrapper
from pydantic import BaseModel, Field
from langflow.base.langchain_utilities.model import LCToolComponent
from langflow.field_typing import Tool
from langflow.inputs import (
DictInput,
DropdownInput,
IntInput,
MultilineInput,
SecretStrInput,
)
from langflow.schema import Data
class QuerySchema(BaseModel):
query: str = Field(..., description="The query to search for.")
query_type: str = Field(
"search",
description="The type of search to perform (e.g., 'news' or 'search').",
)
k: int = Field(4, description="The number of results to return.")
query_params: dict[str, Any] = Field({}, description="Additional query parameters to pass to the API.")
class GoogleSerperAPIComponent(LCToolComponent):
display_name = "Google Serper API [DEPRECATED]"
description = "Call the Serper.dev Google Search API."
name = "GoogleSerperAPI"
icon = "Google"
legacy = True
inputs = [
SecretStrInput(name="serper_api_key", display_name="Serper API Key", required=True),
MultilineInput(
name="query",
display_name="Query",
),
IntInput(name="k", display_name="Number of results", value=4, required=True),
DropdownInput(
name="query_type",
display_name="Query Type",
required=False,
options=["news", "search"],
value="search",
),
DictInput(
name="query_params",
display_name="Query Params",
required=False,
value={
"gl": "us",
"hl": "en",
},
list=True,
),
]
def run_model(self) -> Data | list[Data]:
wrapper = self._build_wrapper(self.k, self.query_type, self.query_params)
results = wrapper.results(query=self.query)
# Adjust the extraction based on the `type`
if self.query_type == "search":
list_results = results.get("organic", [])
elif self.query_type == "news":
list_results = results.get("news", [])
else:
list_results = []
data_list = []
for result in list_results:
result["text"] = result.pop("snippet", "")
data_list.append(Data(data=result))
self.status = data_list
return data_list
def build_tool(self) -> Tool:
return StructuredTool.from_function(
name="google_search",
description="Search Google for recent results.",
func=self._search,
args_schema=self.QuerySchema,
)
def _build_wrapper(
self,
k: int = 5,
query_type: str = "search",
query_params: dict | None = None,
) -> GoogleSerperAPIWrapper:
wrapper_args = {
"serper_api_key": self.serper_api_key,
"k": k,
"type": query_type,
}
# Add query_params if provided
if query_params:
wrapper_args.update(query_params) # Merge with additional query params
# Dynamically pass parameters to the wrapper
return GoogleSerperAPIWrapper(**wrapper_args)
def _search(
self,
query: str,
k: int = 5,
query_type: str = "search",
query_params: dict | None = None,
) -> dict:
wrapper = self._build_wrapper(k, query_type, query_params)
return wrapper.results(query=query)
MCP Tools (stdio)
This component connects to a Model Context Protocol (MCP) server over stdio
and exposes its tools as Langflow tools to be used by an Agent component.
To use the MCP stdio component, follow these steps:
-
Add the MCP-stdio component to your workflow, and connect it to an agent. The flow looks like this:
-
In the MCP stdio component, in the mcp command field, enter the command to start your MCP server. For a Fetch server, the command is:
uvx mcp-server-fetch
-
Open the Playground. Ask the agent to summarize recent tech news. The agent calls the MCP server function
fetch
and returns the summary. This confirms the MCP server is connected and working.
Parameters
Name | Type | Description |
---|---|---|
command |
String |
MCP command (default: |
Name | Type | Description |
---|---|---|
tools |
List[Tool] |
List of tools exposed by the MCP server. |
Component code
mcp_stdio.py
# from langflow.field_typing import Data
import os
from contextlib import AsyncExitStack
from typing import Any
from mcp import ClientSession, StdioServerParameters, types
from mcp.client.stdio import stdio_client
from pydantic import BaseModel, Field, create_model
from langflow.base.mcp.util import create_tool_coroutine, create_tool_func
from langflow.custom import Component
from langflow.field_typing import Tool
from langflow.io import MessageTextInput, Output
class MCPStdioClient:
def __init__(self):
# Initialize session and client objects
self.session: ClientSession | None = None
self.exit_stack = AsyncExitStack()
async def connect_to_server(self, command_str: str):
command = command_str.split(" ")
server_params = StdioServerParameters(
command=command[0], args=command[1:], env={"DEBUG": "true", "PATH": os.environ["PATH"]}
)
stdio_transport = await self.exit_stack.enter_async_context(stdio_client(server_params))
self.stdio, self.write = stdio_transport
self.session = await self.exit_stack.enter_async_context(ClientSession(self.stdio, self.write))
await self.session.initialize()
# List available tools
response = await self.session.list_tools()
return response.tools
def create_input_schema_from_json_schema(schema: dict[str, Any]) -> type[BaseModel]:
"""Converts a JSON schema into a Pydantic model dynamically.
:param schema: The JSON schema as a dictionary.
:return: A Pydantic model class.
"""
if schema.get("type") != "object":
msg = "JSON schema must be of type 'object' at the root level."
raise ValueError(msg)
fields = {}
properties = schema.get("properties", {})
required_fields = set(schema.get("required", []))
for field_name, field_def in properties.items():
# Extract type
field_type_str = field_def.get("type", "str") # Default to string type if not specified
field_type = {
"string": str,
"str": str,
"integer": int,
"int": int,
"number": float,
"boolean": bool,
"array": list,
"object": dict,
}.get(field_type_str, Any)
# Extract description and default if present
field_metadata = {"description": field_def.get("description", "")}
if field_name not in required_fields:
field_metadata["default"] = field_def.get("default", None)
# Create Pydantic field
fields[field_name] = (field_type, Field(**field_metadata))
# Dynamically create the model
return create_model("InputSchema", **fields)
class MCPStdio(Component):
client = MCPStdioClient()
tools = types.ListToolsResult
tool_names = [str]
display_name = "MCP Tools (stdio)"
description = (
"Connects to an MCP server over stdio and exposes it's tools as langflow tools to be used by an Agent."
)
documentation: str = "https://docs.langflow.org/components-custom-components"
icon = "code"
name = "MCPStdio"
inputs = [
MessageTextInput(
name="command",
display_name="mcp command",
info="mcp command",
value="uvx mcp-sse-shim@latest",
tool_mode=True,
),
]
outputs = [
Output(display_name="Tools", name="tools", method="build_output"),
]
async def build_output(self) -> list[Tool]:
if self.client.session is None:
self.tools = await self.client.connect_to_server(self.command)
tool_list = []
for tool in self.tools:
args_schema = create_input_schema_from_json_schema(tool.inputSchema)
tool_list.append(
Tool(
name=tool.name,
description=tool.description,
coroutine=create_tool_coroutine(tool.name, args_schema, self.client.session),
func=create_tool_func(tool.name, args_schema),
)
)
self.tool_names = [tool.name for tool in self.tools]
return tool_list
MCP Tools (SSE)
This component connects to a Model Context Protocol (MCP) server over SSE (Server-Sent Events) and exposes its tools as Langflow tools to be used by an Agent component.
Parameters
Name | Type | Description |
---|---|---|
url |
String |
SSE URL (default: |
Name | Type | Description |
---|---|---|
tools |
List[Tool] |
List of tools exposed by the MCP server. |
Component code
mcp_sse.py
# from langflow.field_typing import Data
from contextlib import AsyncExitStack
import httpx
from mcp import ClientSession, types
from mcp.client.sse import sse_client
from langflow.base.mcp.util import create_tool_coroutine, create_tool_func
from langflow.components.tools.mcp_stdio import create_input_schema_from_json_schema
from langflow.custom import Component
from langflow.field_typing import Tool
from langflow.io import MessageTextInput, Output
from langflow.utils.async_helpers import timeout_context
# Define constant for status code
HTTP_TEMPORARY_REDIRECT = 307
class MCPSseClient:
def __init__(self):
# Initialize session and client objects
self.write = None
self.sse = None
self.session: ClientSession | None = None
self.exit_stack = AsyncExitStack()
async def pre_check_redirect(self, url: str):
"""Check if the URL responds with a 307 Redirect."""
async with httpx.AsyncClient(follow_redirects=False) as client:
response = await client.request("HEAD", url)
if response.status_code == HTTP_TEMPORARY_REDIRECT:
return response.headers.get("Location") # Return the redirect URL
return url # Return the original URL if no redirect
async def connect_to_server(
self, url: str, headers: dict[str, str] | None, timeout_seconds: int = 500, sse_read_timeout_seconds: int = 500
):
if headers is None:
headers = {}
url = await self.pre_check_redirect(url)
async with timeout_context(timeout_seconds):
sse_transport = await self.exit_stack.enter_async_context(
sse_client(url, headers, timeout_seconds, sse_read_timeout_seconds)
)
self.sse, self.write = sse_transport
self.session = await self.exit_stack.enter_async_context(ClientSession(self.sse, self.write))
await self.session.initialize()
# List available tools
response = await self.session.list_tools()
return response.tools
class MCPSse(Component):
client = MCPSseClient()
tools = types.ListToolsResult
tool_names = [str]
display_name = "MCP Tools (SSE)"
description = "Connects to an MCP server over SSE and exposes it's tools as langflow tools to be used by an Agent."
documentation: str = "https://docs.langflow.org/components-custom-components"
icon = "code"
name = "MCPSse"
inputs = [
MessageTextInput(
name="url",
display_name="mcp sse url",
info="sse url",
value="http://localhost:7860/api/v1/mcp/sse",
tool_mode=True,
),
]
outputs = [
Output(display_name="Tools", name="tools", method="build_output"),
]
async def build_output(self) -> list[Tool]:
if self.client.session is None:
self.tools = await self.client.connect_to_server(self.url, {})
tool_list = []
for tool in self.tools:
args_schema = create_input_schema_from_json_schema(tool.inputSchema)
tool_list.append(
Tool(
name=tool.name, # maybe format this
description=tool.description,
coroutine=create_tool_coroutine(tool.name, args_schema, self.client.session),
func=create_tool_func(tool.name, self.client.session),
)
)
self.tool_names = [tool.name for tool in self.tools]
return tool_list
Python code structured tool
This component creates a structured tool from Python code using a dataclass.
The component dynamically updates its configuration based on the provided Python code, allowing for custom function arguments and descriptions.
Parameters
Name | Type | Description |
---|---|---|
tool_code |
String |
Python code for the tool’s dataclass |
tool_name |
String |
Name of the tool |
tool_description |
String |
Description of the tool |
return_direct |
Boolean |
Whether to return the function output directly |
tool_function |
String |
Selected function for the tool |
global_variables |
Dict |
Global variables or data for the tool |
Name | Type | Description |
---|---|---|
result_tool |
Tool |
Structured tool created from the Python code |
Component code
python_code_structured_tool.py
import ast
import json
from typing import Any
from langchain.agents import Tool
from langchain_core.tools import StructuredTool
from loguru import logger
from pydantic.v1 import Field, create_model
from pydantic.v1.fields import Undefined
from typing_extensions import override
from langflow.base.langchain_utilities.model import LCToolComponent
from langflow.inputs.inputs import (
BoolInput,
DropdownInput,
FieldTypes,
HandleInput,
MessageTextInput,
MultilineInput,
)
from langflow.io import Output
from langflow.schema import Data
from langflow.schema.dotdict import dotdict
class PythonCodeStructuredTool(LCToolComponent):
DEFAULT_KEYS = [
"code",
"_type",
"text_key",
"tool_code",
"tool_name",
"tool_description",
"return_direct",
"tool_function",
"global_variables",
"_classes",
"_functions",
]
display_name = "Python Code Structured"
description = "structuredtool dataclass code to tool"
documentation = "https://python.langchain.com/docs/modules/tools/custom_tools/#structuredtool-dataclass"
name = "PythonCodeStructuredTool"
icon = "Python"
field_order = ["name", "description", "tool_code", "return_direct", "tool_function"]
legacy: bool = True
inputs = [
MultilineInput(
name="tool_code",
display_name="Tool Code",
info="Enter the dataclass code.",
placeholder="def my_function(args):\n pass",
required=True,
real_time_refresh=True,
refresh_button=True,
),
MessageTextInput(
name="tool_name",
display_name="Tool Name",
info="Enter the name of the tool.",
required=True,
),
MessageTextInput(
name="tool_description",
display_name="Description",
info="Enter the description of the tool.",
required=True,
),
BoolInput(
name="return_direct",
display_name="Return Directly",
info="Should the tool return the function output directly?",
),
DropdownInput(
name="tool_function",
display_name="Tool Function",
info="Select the function for additional expressions.",
options=[],
required=True,
real_time_refresh=True,
refresh_button=True,
),
HandleInput(
name="global_variables",
display_name="Global Variables",
info="Enter the global variables or Create Data Component.",
input_types=["Data"],
field_type=FieldTypes.DICT,
is_list=True,
),
MessageTextInput(name="_classes", display_name="Classes", advanced=True),
MessageTextInput(name="_functions", display_name="Functions", advanced=True),
]
outputs = [
Output(display_name="Tool", name="result_tool", method="build_tool"),
]
@override
async def update_build_config(
self, build_config: dotdict, field_value: Any, field_name: str | None = None
) -> dotdict:
if field_name is None:
return build_config
if field_name not in {"tool_code", "tool_function"}:
return build_config
try:
named_functions = {}
[classes, functions] = self._parse_code(build_config["tool_code"]["value"])
existing_fields = {}
if len(build_config) > len(self.DEFAULT_KEYS):
for key in build_config.copy():
if key not in self.DEFAULT_KEYS:
existing_fields[key] = build_config.pop(key)
names = []
for func in functions:
named_functions[func["name"]] = func
names.append(func["name"])
for arg in func["args"]:
field_name = f"{func['name']}|{arg['name']}"
if field_name in existing_fields:
build_config[field_name] = existing_fields[field_name]
continue
field = MessageTextInput(
display_name=f"{arg['name']}: Description",
name=field_name,
info=f"Enter the description for {arg['name']}",
required=True,
)
build_config[field_name] = field.to_dict()
build_config["_functions"]["value"] = json.dumps(named_functions)
build_config["_classes"]["value"] = json.dumps(classes)
build_config["tool_function"]["options"] = names
except Exception as e: # noqa: BLE001
self.status = f"Failed to extract names: {e}"
logger.opt(exception=True).debug(self.status)
build_config["tool_function"]["options"] = ["Failed to parse", str(e)]
return build_config
async def build_tool(self) -> Tool:
local_namespace = {} # type: ignore[var-annotated]
modules = self._find_imports(self.tool_code)
import_code = ""
for module in modules["imports"]:
import_code += f"global {module}\nimport {module}\n"
for from_module in modules["from_imports"]:
for alias in from_module.names:
import_code += f"global {alias.name}\n"
import_code += (
f"from {from_module.module} import {', '.join([alias.name for alias in from_module.names])}\n"
)
exec(import_code, globals())
exec(self.tool_code, globals(), local_namespace)
class PythonCodeToolFunc:
params: dict = {}
def run(**kwargs):
for key, arg in kwargs.items():
if key not in PythonCodeToolFunc.params:
PythonCodeToolFunc.params[key] = arg
return local_namespace[self.tool_function](**PythonCodeToolFunc.params)
globals_ = globals()
local = {}
local[self.tool_function] = PythonCodeToolFunc
globals_.update(local)
if isinstance(self.global_variables, list):
for data in self.global_variables:
if isinstance(data, Data):
globals_.update(data.data)
elif isinstance(self.global_variables, dict):
globals_.update(self.global_variables)
classes = json.loads(self._attributes["_classes"])
for class_dict in classes:
exec("\n".join(class_dict["code"]), globals_)
named_functions = json.loads(self._attributes["_functions"])
schema_fields = {}
for attr in self._attributes:
if attr in self.DEFAULT_KEYS:
continue
func_name = attr.split("|")[0]
field_name = attr.split("|")[1]
func_arg = self._find_arg(named_functions, func_name, field_name)
if func_arg is None:
msg = f"Failed to find arg: {field_name}"
raise ValueError(msg)
field_annotation = func_arg["annotation"]
field_description = self._get_value(self._attributes[attr], str)
if field_annotation:
exec(f"temp_annotation_type = {field_annotation}", globals_)
schema_annotation = globals_["temp_annotation_type"]
else:
schema_annotation = Any
schema_fields[field_name] = (
schema_annotation,
Field(
default=func_arg.get("default", Undefined),
description=field_description,
),
)
if "temp_annotation_type" in globals_:
globals_.pop("temp_annotation_type")
python_code_tool_schema = None
if schema_fields:
python_code_tool_schema = create_model("PythonCodeToolSchema", **schema_fields)
return StructuredTool.from_function(
func=local[self.tool_function].run,
args_schema=python_code_tool_schema,
name=self.tool_name,
description=self.tool_description,
return_direct=self.return_direct,
)
async def update_frontend_node(self, new_frontend_node: dict, current_frontend_node: dict):
"""This function is called after the code validation is done."""
frontend_node = await super().update_frontend_node(new_frontend_node, current_frontend_node)
frontend_node["template"] = await self.update_build_config(
frontend_node["template"],
frontend_node["template"]["tool_code"]["value"],
"tool_code",
)
frontend_node = await super().update_frontend_node(new_frontend_node, current_frontend_node)
for key in frontend_node["template"]:
if key in self.DEFAULT_KEYS:
continue
frontend_node["template"] = await self.update_build_config(
frontend_node["template"], frontend_node["template"][key]["value"], key
)
frontend_node = await super().update_frontend_node(new_frontend_node, current_frontend_node)
return frontend_node
def _parse_code(self, code: str) -> tuple[list[dict], list[dict]]:
parsed_code = ast.parse(code)
lines = code.split("\n")
classes = []
functions = []
for node in parsed_code.body:
if isinstance(node, ast.ClassDef):
class_lines = lines[node.lineno - 1 : node.end_lineno]
class_lines[-1] = class_lines[-1][: node.end_col_offset]
class_lines[0] = class_lines[0][node.col_offset :]
classes.append(
{
"name": node.name,
"code": class_lines,
}
)
continue
if not isinstance(node, ast.FunctionDef):
continue
func = {"name": node.name, "args": []}
for arg in node.args.args:
if arg.lineno != arg.end_lineno:
msg = "Multiline arguments are not supported"
raise ValueError(msg)
func_arg = {
"name": arg.arg,
"annotation": None,
}
for default in node.args.defaults:
if (
arg.lineno > default.lineno
or arg.col_offset > default.col_offset
or (
arg.end_lineno is not None
and default.end_lineno is not None
and arg.end_lineno < default.end_lineno
)
or (
arg.end_col_offset is not None
and default.end_col_offset is not None
and arg.end_col_offset < default.end_col_offset
)
):
continue
if isinstance(default, ast.Name):
func_arg["default"] = default.id
elif isinstance(default, ast.Constant):
func_arg["default"] = default.value
if arg.annotation:
annotation_line = lines[arg.annotation.lineno - 1]
annotation_line = annotation_line[: arg.annotation.end_col_offset]
annotation_line = annotation_line[arg.annotation.col_offset :]
func_arg["annotation"] = annotation_line
if isinstance(func_arg["annotation"], str) and func_arg["annotation"].count("=") > 0:
func_arg["annotation"] = "=".join(func_arg["annotation"].split("=")[:-1]).strip()
if isinstance(func["args"], list):
func["args"].append(func_arg)
functions.append(func)
return classes, functions
def _find_imports(self, code: str) -> dotdict:
imports: list[str] = []
from_imports = []
parsed_code = ast.parse(code)
for node in parsed_code.body:
if isinstance(node, ast.Import):
imports.extend(alias.name for alias in node.names)
elif isinstance(node, ast.ImportFrom):
from_imports.append(node)
return dotdict({"imports": imports, "from_imports": from_imports})
def _get_value(self, value: Any, annotation: Any) -> Any:
return value if isinstance(value, annotation) else value["value"]
def _find_arg(self, named_functions: dict, func_name: str, arg_name: str) -> dict | None:
for arg in named_functions[func_name]["args"]:
if arg["name"] == arg_name:
return arg
return None
Python REPL Tool
This component creates a Python REPL (Read-Eval-Print Loop) tool for executing Python code.
Parameters
Name | Type | Description |
---|---|---|
name |
String |
The name of the tool (default: "python_repl") |
description |
String |
A description of the tool’s functionality |
global_imports |
List[String] |
List of modules to import globally (default: ["math"]) |
Name | Type | Description |
---|---|---|
tool |
Tool |
Python REPL tool for use in LangChain |
Component code
python_repl.py
import importlib
from langchain.tools import StructuredTool
from langchain_core.tools import ToolException
from langchain_experimental.utilities import PythonREPL
from loguru import logger
from pydantic import BaseModel, Field
from langflow.base.langchain_utilities.model import LCToolComponent
from langflow.field_typing import Tool
from langflow.inputs import StrInput
from langflow.schema import Data
class PythonREPLToolComponent(LCToolComponent):
display_name = "Python REPL [DEPRECATED]"
description = "A tool for running Python code in a REPL environment."
name = "PythonREPLTool"
icon = "Python"
legacy = True
inputs = [
StrInput(
name="name",
display_name="Tool Name",
info="The name of the tool.",
value="python_repl",
),
StrInput(
name="description",
display_name="Tool Description",
info="A description of the tool.",
value="A Python shell. Use this to execute python commands. "
"Input should be a valid python command. "
"If you want to see the output of a value, you should print it out with `print(...)`.",
),
StrInput(
name="global_imports",
display_name="Global Imports",
info="A comma-separated list of modules to import globally, e.g. 'math,numpy'.",
value="math",
),
StrInput(
name="code",
display_name="Python Code",
info="The Python code to execute.",
value="print('Hello, World!')",
),
]
class PythonREPLSchema(BaseModel):
code: str = Field(..., description="The Python code to execute.")
def get_globals(self, global_imports: str | list[str]) -> dict:
global_dict = {}
if isinstance(global_imports, str):
modules = [module.strip() for module in global_imports.split(",")]
elif isinstance(global_imports, list):
modules = global_imports
else:
msg = "global_imports must be either a string or a list"
raise TypeError(msg)
for module in modules:
try:
imported_module = importlib.import_module(module)
global_dict[imported_module.__name__] = imported_module
except ImportError as e:
msg = f"Could not import module {module}"
raise ImportError(msg) from e
return global_dict
def build_tool(self) -> Tool:
globals_ = self.get_globals(self.global_imports)
python_repl = PythonREPL(_globals=globals_)
def run_python_code(code: str) -> str:
try:
return python_repl.run(code)
except Exception as e:
logger.opt(exception=True).debug("Error running Python code")
raise ToolException(str(e)) from e
tool = StructuredTool.from_function(
name=self.name,
description=self.description,
func=run_python_code,
args_schema=self.PythonREPLSchema,
)
self.status = f"Python REPL Tool created with global imports: {self.global_imports}"
return tool
def run_model(self) -> list[Data]:
tool = self.build_tool()
result = tool.run(self.code)
return [Data(data={"result": result})]
Retriever Tool
This component creates a tool for interacting with a retriever in LangChain.
Parameters
Name | Type | Description |
---|---|---|
retriever |
BaseRetriever |
The retriever to interact with |
name |
String |
The name of the tool |
description |
String |
A description of the tool’s functionality |
Name | Type | Description |
---|---|---|
tool |
Tool |
Retriever tool for use in LangChain |
Component code
retriever.py
from langchain_core.tools import create_retriever_tool
from langflow.custom import CustomComponent
from langflow.field_typing import BaseRetriever, Tool
class RetrieverToolComponent(CustomComponent):
display_name = "RetrieverTool"
description = "Tool for interacting with retriever"
name = "RetrieverTool"
legacy = True
icon = "LangChain"
def build_config(self):
return {
"retriever": {
"display_name": "Retriever",
"info": "Retriever to interact with",
"type": BaseRetriever,
"input_types": ["Retriever"],
},
"name": {"display_name": "Name", "info": "Name of the tool"},
"description": {"display_name": "Description", "info": "Description of the tool"},
}
def build(self, retriever: BaseRetriever, name: str, description: str, **kwargs) -> Tool:
_ = kwargs
return create_retriever_tool(
retriever=retriever,
name=name,
description=description,
)
SearXNG Search Tool
This component creates a tool for searching using SearXNG, a metasearch engine.
Parameters
Name | Type | Description |
---|---|---|
url |
String |
The URL of the SearXNG instance |
max_results |
Integer |
Maximum number of results to return |
categories |
List[String] |
Categories to search in |
language |
String |
Language for the search results |
Name | Type | Description |
---|---|---|
result_tool |
Tool |
SearXNG search tool for use in LangChain |
Component code
searxng.py
import json
from collections.abc import Sequence
from typing import Any
import requests
from langchain.agents import Tool
from langchain_core.tools import StructuredTool
from loguru import logger
from pydantic.v1 import Field, create_model
from langflow.base.langchain_utilities.model import LCToolComponent
from langflow.inputs import DropdownInput, IntInput, MessageTextInput, MultiselectInput
from langflow.io import Output
from langflow.schema.dotdict import dotdict
class SearXNGToolComponent(LCToolComponent):
search_headers: dict = {}
display_name = "SearXNG Search"
description = "A component that searches for tools using SearXNG."
name = "SearXNGTool"
legacy: bool = True
inputs = [
MessageTextInput(
name="url",
display_name="URL",
value="http://localhost",
required=True,
refresh_button=True,
),
IntInput(
name="max_results",
display_name="Max Results",
value=10,
required=True,
),
MultiselectInput(
name="categories",
display_name="Categories",
options=[],
value=[],
),
DropdownInput(
name="language",
display_name="Language",
options=[],
),
]
outputs = [
Output(display_name="Tool", name="result_tool", method="build_tool"),
]
def update_build_config(self, build_config: dotdict, field_value: Any, field_name: str | None = None) -> dotdict:
if field_name is None:
return build_config
if field_name != "url":
return build_config
try:
url = f"{field_value}/config"
response = requests.get(url=url, headers=self.search_headers.copy(), timeout=10)
data = None
if response.headers.get("Content-Encoding") == "zstd":
data = json.loads(response.content)
else:
data = response.json()
build_config["categories"]["options"] = data["categories"].copy()
for selected_category in build_config["categories"]["value"]:
if selected_category not in build_config["categories"]["options"]:
build_config["categories"]["value"].remove(selected_category)
languages = list(data["locales"])
build_config["language"]["options"] = languages.copy()
except Exception as e: # noqa: BLE001
self.status = f"Failed to extract names: {e}"
logger.opt(exception=True).debug(self.status)
build_config["categories"]["options"] = ["Failed to parse", str(e)]
return build_config
def build_tool(self) -> Tool:
class SearxSearch:
_url: str = ""
_categories: list[str] = []
_language: str = ""
_headers: dict = {}
_max_results: int = 10
@staticmethod
def search(query: str, categories: Sequence[str] = ()) -> list:
if not SearxSearch._categories and not categories:
msg = "No categories provided."
raise ValueError(msg)
all_categories = SearxSearch._categories + list(set(categories) - set(SearxSearch._categories))
try:
url = f"{SearxSearch._url}/"
headers = SearxSearch._headers.copy()
response = requests.get(
url=url,
headers=headers,
params={
"q": query,
"categories": ",".join(all_categories),
"language": SearxSearch._language,
"format": "json",
},
timeout=10,
).json()
num_results = min(SearxSearch._max_results, len(response["results"]))
return [response["results"][i] for i in range(num_results)]
except Exception as e: # noqa: BLE001
logger.opt(exception=True).debug("Error running SearXNG Search")
return [f"Failed to search: {e}"]
SearxSearch._url = self.url
SearxSearch._categories = self.categories.copy()
SearxSearch._language = self.language
SearxSearch._headers = self.search_headers.copy()
SearxSearch._max_results = self.max_results
globals_ = globals()
local = {}
local["SearxSearch"] = SearxSearch
globals_.update(local)
schema_fields = {
"query": (str, Field(..., description="The query to search for.")),
"categories": (
list[str],
Field(default=[], description="The categories to search in."),
),
}
searx_search_schema = create_model("SearxSearchSchema", **schema_fields)
return StructuredTool.from_function(
func=local["SearxSearch"].search,
args_schema=searx_search_schema,
name="searxng_search_tool",
description="A tool that searches for tools using SearXNG.\nThe available categories are: "
+ ", ".join(self.categories),
)
Search API
This component calls the searchapi.io
API. It can be used to search the web for information.
For more information, see the SearchAPI documentation.
Parameters
Name | Display Name | Info |
---|---|---|
engine |
Engine |
The search engine to use (default: "google") |
api_key |
SearchAPI API Key |
The API key for authenticating with SearchAPI |
input_value |
Input |
The search query or input for the API call |
search_params |
Search parameters |
Additional parameters for customizing the search |
Name | Display Name | Info |
---|---|---|
data |
Search Results |
List of Data objects containing search results |
tool |
Search API Tool |
A Tool object for use in LangChain workflows |
Component code
search_api.py
from typing import Any
from langchain.tools import StructuredTool
from langchain_community.utilities.searchapi import SearchApiAPIWrapper
from pydantic import BaseModel, Field
from langflow.base.langchain_utilities.model import LCToolComponent
from langflow.field_typing import Tool
from langflow.inputs import DictInput, IntInput, MessageTextInput, MultilineInput, SecretStrInput
from langflow.schema import Data
class SearchAPIComponent(LCToolComponent):
display_name: str = "Search API [DEPRECATED]"
description: str = "Call the searchapi.io API with result limiting"
name = "SearchAPI"
documentation: str = "https://www.searchapi.io/docs/google"
icon = "SearchAPI"
legacy = True
inputs = [
MessageTextInput(name="engine", display_name="Engine", value="google"),
SecretStrInput(name="api_key", display_name="SearchAPI API Key", required=True),
MultilineInput(
name="input_value",
display_name="Input",
),
DictInput(name="search_params", display_name="Search parameters", advanced=True, is_list=True),
IntInput(name="max_results", display_name="Max Results", value=5, advanced=True),
IntInput(name="max_snippet_length", display_name="Max Snippet Length", value=100, advanced=True),
]
class SearchAPISchema(BaseModel):
query: str = Field(..., description="The search query")
params: dict[str, Any] = Field(default_factory=dict, description="Additional search parameters")
max_results: int = Field(5, description="Maximum number of results to return")
max_snippet_length: int = Field(100, description="Maximum length of each result snippet")
def _build_wrapper(self):
return SearchApiAPIWrapper(engine=self.engine, searchapi_api_key=self.api_key)
def build_tool(self) -> Tool:
wrapper = self._build_wrapper()
def search_func(
query: str, params: dict[str, Any] | None = None, max_results: int = 5, max_snippet_length: int = 100
) -> list[dict[str, Any]]:
params = params or {}
full_results = wrapper.results(query=query, **params)
organic_results = full_results.get("organic_results", [])[:max_results]
limited_results = []
for result in organic_results:
limited_result = {
"title": result.get("title", "")[:max_snippet_length],
"link": result.get("link", ""),
"snippet": result.get("snippet", "")[:max_snippet_length],
}
limited_results.append(limited_result)
return limited_results
tool = StructuredTool.from_function(
name="search_api",
description="Search for recent results using searchapi.io with result limiting",
func=search_func,
args_schema=self.SearchAPISchema,
)
self.status = f"Search API Tool created with engine: {self.engine}"
return tool
def run_model(self) -> list[Data]:
tool = self.build_tool()
results = tool.run(
{
"query": self.input_value,
"params": self.search_params or {},
"max_results": self.max_results,
"max_snippet_length": self.max_snippet_length,
}
)
data_list = [Data(data=result, text=result.get("snippet", "")) for result in results]
self.status = data_list
return data_list
Serp Search API
This component creates a tool for searching using the Serp API.
Parameters
Name | Type | Description |
---|---|---|
serpapi_api_key |
SecretString |
API key for Serp API authentication |
input_value |
String |
Search query input |
search_params |
Dict |
Additional search parameters (optional) |
Name | Type | Description |
---|---|---|
results |
List[Data] |
List of search results |
tool |
Tool |
Serp API search tool for use in LangChain |
Component code
serp_api.py
from typing import Any
from langchain.tools import StructuredTool
from langchain_community.utilities.serpapi import SerpAPIWrapper
from langchain_core.tools import ToolException
from loguru import logger
from pydantic import BaseModel, Field
from langflow.base.langchain_utilities.model import LCToolComponent
from langflow.field_typing import Tool
from langflow.inputs import DictInput, IntInput, MultilineInput, SecretStrInput
from langflow.schema import Data
class SerpAPISchema(BaseModel):
"""Schema for SerpAPI search parameters."""
query: str = Field(..., description="The search query")
params: dict[str, Any] | None = Field(
default={
"engine": "google",
"google_domain": "google.com",
"gl": "us",
"hl": "en",
},
description="Additional search parameters",
)
max_results: int = Field(5, description="Maximum number of results to return")
max_snippet_length: int = Field(100, description="Maximum length of each result snippet")
class SerpAPIComponent(LCToolComponent):
display_name = "Serp Search API [DEPRECATED]"
description = "Call Serp Search API with result limiting"
name = "SerpAPI"
icon = "SerpSearch"
legacy = True
inputs = [
SecretStrInput(name="serpapi_api_key", display_name="SerpAPI API Key", required=True),
MultilineInput(
name="input_value",
display_name="Input",
),
DictInput(name="search_params", display_name="Parameters", advanced=True, is_list=True),
IntInput(name="max_results", display_name="Max Results", value=5, advanced=True),
IntInput(name="max_snippet_length", display_name="Max Snippet Length", value=100, advanced=True),
]
def _build_wrapper(self, params: dict[str, Any] | None = None) -> SerpAPIWrapper:
"""Build a SerpAPIWrapper with the provided parameters."""
params = params or {}
if params:
return SerpAPIWrapper(
serpapi_api_key=self.serpapi_api_key,
params=params,
)
return SerpAPIWrapper(serpapi_api_key=self.serpapi_api_key)
def build_tool(self) -> Tool:
wrapper = self._build_wrapper(self.search_params)
def search_func(
query: str, params: dict[str, Any] | None = None, max_results: int = 5, max_snippet_length: int = 100
) -> list[dict[str, Any]]:
try:
local_wrapper = wrapper
if params:
local_wrapper = self._build_wrapper(params)
full_results = local_wrapper.results(query)
organic_results = full_results.get("organic_results", [])[:max_results]
limited_results = []
for result in organic_results:
limited_result = {
"title": result.get("title", "")[:max_snippet_length],
"link": result.get("link", ""),
"snippet": result.get("snippet", "")[:max_snippet_length],
}
limited_results.append(limited_result)
except Exception as e:
error_message = f"Error in SerpAPI search: {e!s}"
logger.debug(error_message)
raise ToolException(error_message) from e
return limited_results
tool = StructuredTool.from_function(
name="serp_search_api",
description="Search for recent results using SerpAPI with result limiting",
func=search_func,
args_schema=SerpAPISchema,
)
self.status = "SerpAPI Tool created"
return tool
def run_model(self) -> list[Data]:
tool = self.build_tool()
try:
results = tool.run(
{
"query": self.input_value,
"params": self.search_params or {},
"max_results": self.max_results,
"max_snippet_length": self.max_snippet_length,
}
)
data_list = [Data(data=result, text=result.get("snippet", "")) for result in results]
except Exception as e: # noqa: BLE001
logger.opt(exception=True).debug("Error running SerpAPI")
self.status = f"Error: {e}"
return [Data(data={"error": str(e)}, text=str(e))]
self.status = data_list # type: ignore[assignment]
return data_list
Tavily search API
This component creates a tool for performing web searches using the Tavily API.
Parameters
Name | Type | Description | Required | Default |
---|---|---|---|---|
api_key |
SecretString |
Tavily API Key |
Yes |
- |
query |
String |
Search query |
Yes |
- |
search_depth |
Enum |
Depth of search (BASIC/ADVANCED) |
No |
BASIC |
topic |
Enum |
Search category (GENERAL/NEWS) |
No |
GENERAL |
max_results |
Integer |
Maximum number of results |
No |
5 |
include_images |
Boolean |
Include related images |
No |
False |
include_answer |
Boolean |
Include short answer |
No |
False |
Name | Type | Description |
---|---|---|
results |
List[Data] |
List of search results |
tool |
Tool |
Tavily search tool for use in LangChain |
Component code
tavily.py
import httpx
from loguru import logger
from langflow.custom import Component
from langflow.helpers.data import data_to_text
from langflow.io import BoolInput, DropdownInput, IntInput, MessageTextInput, Output, SecretStrInput
from langflow.schema import Data
from langflow.schema.message import Message
class TavilySearchComponent(Component):
display_name = "Tavily AI Search"
description = """**Tavily AI** is a search engine optimized for LLMs and RAG, \
aimed at efficient, quick, and persistent search results."""
icon = "TavilyIcon"
inputs = [
SecretStrInput(
name="api_key",
display_name="Tavily API Key",
required=True,
info="Your Tavily API Key.",
),
MessageTextInput(
name="query",
display_name="Search Query",
info="The search query you want to execute with Tavily.",
tool_mode=True,
),
DropdownInput(
name="search_depth",
display_name="Search Depth",
info="The depth of the search.",
options=["basic", "advanced"],
value="advanced",
advanced=True,
),
DropdownInput(
name="topic",
display_name="Search Topic",
info="The category of the search.",
options=["general", "news"],
value="general",
advanced=True,
),
DropdownInput(
name="time_range",
display_name="Time Range",
info="The time range back from the current date to include in the search results.",
options=["day", "week", "month", "year"],
value=None,
advanced=True,
combobox=True,
),
IntInput(
name="max_results",
display_name="Max Results",
info="The maximum number of search results to return.",
value=5,
advanced=True,
),
BoolInput(
name="include_images",
display_name="Include Images",
info="Include a list of query-related images in the response.",
value=True,
advanced=True,
),
BoolInput(
name="include_answer",
display_name="Include Answer",
info="Include a short answer to original query.",
value=True,
advanced=True,
),
]
outputs = [
Output(display_name="Data", name="data", method="fetch_content"),
Output(display_name="Text", name="text", method="fetch_content_text"),
]
def fetch_content(self) -> list[Data]:
try:
url = "https://api.tavily.com/search"
headers = {
"content-type": "application/json",
"accept": "application/json",
}
payload = {
"api_key": self.api_key,
"query": self.query,
"search_depth": self.search_depth,
"topic": self.topic,
"max_results": self.max_results,
"include_images": self.include_images,
"include_answer": self.include_answer,
"time_range": self.time_range,
}
with httpx.Client() as client:
response = client.post(url, json=payload, headers=headers)
response.raise_for_status()
search_results = response.json()
data_results = []
if self.include_answer and search_results.get("answer"):
data_results.append(Data(text=search_results["answer"]))
for result in search_results.get("results", []):
content = result.get("content", "")
data_results.append(
Data(
text=content,
data={
"title": result.get("title"),
"url": result.get("url"),
"content": content,
"score": result.get("score"),
},
)
)
if self.include_images and search_results.get("images"):
data_results.append(Data(text="Images found", data={"images": search_results["images"]}))
except httpx.HTTPStatusError as exc:
error_message = f"HTTP error occurred: {exc.response.status_code} - {exc.response.text}"
logger.error(error_message)
return [Data(text=error_message, data={"error": error_message})]
except httpx.RequestError as exc:
error_message = f"Request error occurred: {exc}"
logger.error(error_message)
return [Data(text=error_message, data={"error": error_message})]
except ValueError as exc:
error_message = f"Invalid response format: {exc}"
logger.error(error_message)
return [Data(text=error_message, data={"error": error_message})]
else:
self.status = data_results
return data_results
def fetch_content_text(self) -> Message:
data = self.fetch_content()
result_string = data_to_text("{text}", data)
self.status = result_string
return Message(text=result_string)
Wikidata
This component performs a search using the Wikidata API.
Parameters
Name | Display Name | Info |
---|---|---|
query |
Query |
The text query for similarity search on Wikidata. |
Name | Display Name | Info |
---|---|---|
data |
Data |
The search results from Wikidata API as a list of Data objects. |
text |
Message |
The search results formatted as a text message. |
Component code
wikidata.py
import httpx
from httpx import HTTPError
from langchain_core.tools import ToolException
from langflow.custom import Component
from langflow.helpers.data import data_to_text
from langflow.io import MultilineInput, Output
from langflow.schema import Data
from langflow.schema.message import Message
class WikidataComponent(Component):
display_name = "Wikidata"
description = "Performs a search using the Wikidata API."
icon = "Wikipedia"
inputs = [
MultilineInput(
name="query",
display_name="Query",
info="The text query for similarity search on Wikidata.",
required=True,
tool_mode=True,
),
]
outputs = [
Output(display_name="Data", name="data", method="fetch_content"),
Output(display_name="Message", name="text", method="fetch_content_text"),
]
def fetch_content(self) -> list[Data]:
try:
# Define request parameters for Wikidata API
params = {
"action": "wbsearchentities",
"format": "json",
"search": self.query,
"language": "en",
}
# Send request to Wikidata API
wikidata_api_url = "https://www.wikidata.org/w/api.php"
response = httpx.get(wikidata_api_url, params=params)
response.raise_for_status()
response_json = response.json()
# Extract search results
results = response_json.get("search", [])
if not results:
return [Data(data={"error": "No search results found for the given query."})]
# Transform the API response into Data objects
data = [
Data(
text=f"{result['label']}: {result.get('description', '')}",
data={
"label": result["label"],
"id": result.get("id"),
"url": result.get("url"),
"description": result.get("description", ""),
"concepturi": result.get("concepturi"),
},
)
for result in results
]
self.status = data
except HTTPError as e:
error_message = f"HTTP Error in Wikidata Search API: {e!s}"
raise ToolException(error_message) from None
except KeyError as e:
error_message = f"Data parsing error in Wikidata API response: {e!s}"
raise ToolException(error_message) from None
except ValueError as e:
error_message = f"Value error in Wikidata API: {e!s}"
raise ToolException(error_message) from None
else:
return data
def fetch_content_text(self) -> Message:
data = self.fetch_content()
result_string = data_to_text("{text}", data)
self.status = result_string
return Message(text=result_string)
Wikipedia API
This component creates a tool for searching and retrieving information from Wikipedia.
Parameters
Name | Type | Description |
---|---|---|
input_value |
String |
Search query input |
lang |
String |
Language code for Wikipedia (default: "en") |
k |
Integer |
Number of results to return |
load_all_available_meta |
Boolean |
Whether to load all available metadata (advanced) |
doc_content_chars_max |
Integer |
Maximum number of characters for document content (advanced) |
Name | Type | Description |
---|---|---|
results |
List[Data] |
List of Wikipedia search results |
tool |
Tool |
Wikipedia search tool for use in LangChain |
Component code
wikipedia_api.py
from typing import cast
from langchain_community.tools import WikipediaQueryRun
from langchain_community.utilities.wikipedia import WikipediaAPIWrapper
from langflow.base.langchain_utilities.model import LCToolComponent
from langflow.field_typing import Tool
from langflow.inputs import BoolInput, IntInput, MessageTextInput, MultilineInput
from langflow.schema import Data
class WikipediaAPIComponent(LCToolComponent):
display_name = "Wikipedia API [Deprecated]"
description = "Call Wikipedia API."
name = "WikipediaAPI"
icon = "Wikipedia"
legacy = True
inputs = [
MultilineInput(
name="input_value",
display_name="Input",
),
MessageTextInput(name="lang", display_name="Language", value="en"),
IntInput(name="k", display_name="Number of results", value=4, required=True),
BoolInput(name="load_all_available_meta", display_name="Load all available meta", value=False, advanced=True),
IntInput(
name="doc_content_chars_max", display_name="Document content characters max", value=4000, advanced=True
),
]
def run_model(self) -> list[Data]:
wrapper = self._build_wrapper()
docs = wrapper.load(self.input_value)
data = [Data.from_document(doc) for doc in docs]
self.status = data
return data
def build_tool(self) -> Tool:
wrapper = self._build_wrapper()
return cast("Tool", WikipediaQueryRun(api_wrapper=wrapper))
def _build_wrapper(self) -> WikipediaAPIWrapper:
return WikipediaAPIWrapper(
top_k_results=self.k,
lang=self.lang,
load_all_available_meta=self.load_all_available_meta,
doc_content_chars_max=self.doc_content_chars_max,
)
Wolfram Alpha API
This component creates a tool for querying the Wolfram Alpha API.
Parameters
Name | Type | Description |
---|---|---|
input_value |
String |
Query input for Wolfram Alpha |
app_id |
SecretString |
Wolfram Alpha API App ID |
Name | Type | Description |
---|---|---|
results |
List[Data] |
List containing the Wolfram Alpha API response |
tool |
Tool |
Wolfram Alpha API tool for use in LangChain |
Component code
wolfram_alpha_api.py
from langchain_community.utilities.wolfram_alpha import WolframAlphaAPIWrapper
from langflow.base.langchain_utilities.model import LCToolComponent
from langflow.field_typing import Tool
from langflow.inputs import MultilineInput, SecretStrInput
from langflow.schema import Data
class WolframAlphaAPIComponent(LCToolComponent):
display_name = "WolframAlpha API"
description = """Enables queries to Wolfram Alpha for computational data, facts, and calculations across various \
topics, delivering structured responses."""
name = "WolframAlphaAPI"
inputs = [
MultilineInput(
name="input_value", display_name="Input Query", info="Example query: 'What is the population of France?'"
),
SecretStrInput(name="app_id", display_name="App ID", required=True),
]
icon = "WolframAlphaAPI"
def run_model(self) -> list[Data]:
wrapper = self._build_wrapper()
result_str = wrapper.run(self.input_value)
data = [Data(text=result_str)]
self.status = data
return data
def build_tool(self) -> Tool:
wrapper = self._build_wrapper()
return Tool(name="wolfram_alpha_api", description="Answers mathematical questions.", func=wrapper.run)
def _build_wrapper(self) -> WolframAlphaAPIWrapper:
return WolframAlphaAPIWrapper(wolfram_alpha_appid=self.app_id)
Yahoo Finance News Tool
This component creates a tool for retrieving news from Yahoo Finance.
Parameters
This component does not have any input parameters.
Name | Type | Description |
---|---|---|
tool |
Tool |
Yahoo Finance News tool for use in LangChain |
Component code
yahoo.py
import ast
import pprint
from enum import Enum
import yfinance as yf
from langchain_core.tools import ToolException
from loguru import logger
from pydantic import BaseModel, Field
from langflow.custom import Component
from langflow.inputs import DropdownInput, IntInput, MessageTextInput
from langflow.io import Output
from langflow.schema import Data
from langflow.schema.message import Message
class YahooFinanceMethod(Enum):
GET_INFO = "get_info"
GET_NEWS = "get_news"
GET_ACTIONS = "get_actions"
GET_ANALYSIS = "get_analysis"
GET_BALANCE_SHEET = "get_balance_sheet"
GET_CALENDAR = "get_calendar"
GET_CASHFLOW = "get_cashflow"
GET_INSTITUTIONAL_HOLDERS = "get_institutional_holders"
GET_RECOMMENDATIONS = "get_recommendations"
GET_SUSTAINABILITY = "get_sustainability"
GET_MAJOR_HOLDERS = "get_major_holders"
GET_MUTUALFUND_HOLDERS = "get_mutualfund_holders"
GET_INSIDER_PURCHASES = "get_insider_purchases"
GET_INSIDER_TRANSACTIONS = "get_insider_transactions"
GET_INSIDER_ROSTER_HOLDERS = "get_insider_roster_holders"
GET_DIVIDENDS = "get_dividends"
GET_CAPITAL_GAINS = "get_capital_gains"
GET_SPLITS = "get_splits"
GET_SHARES = "get_shares"
GET_FAST_INFO = "get_fast_info"
GET_SEC_FILINGS = "get_sec_filings"
GET_RECOMMENDATIONS_SUMMARY = "get_recommendations_summary"
GET_UPGRADES_DOWNGRADES = "get_upgrades_downgrades"
GET_EARNINGS = "get_earnings"
GET_INCOME_STMT = "get_income_stmt"
class YahooFinanceSchema(BaseModel):
symbol: str = Field(..., description="The stock symbol to retrieve data for.")
method: YahooFinanceMethod = Field(YahooFinanceMethod.GET_INFO, description="The type of data to retrieve.")
num_news: int | None = Field(5, description="The number of news articles to retrieve.")
class YfinanceComponent(Component):
display_name = "Yahoo Finance"
description = """Uses [yfinance](https://pypi.org/project/yfinance/) (unofficial package) \
to access financial data and market information from Yahoo Finance."""
icon = "trending-up"
inputs = [
MessageTextInput(
name="symbol",
display_name="Stock Symbol",
info="The stock symbol to retrieve data for (e.g., AAPL, GOOG).",
tool_mode=True,
),
DropdownInput(
name="method",
display_name="Data Method",
info="The type of data to retrieve.",
options=list(YahooFinanceMethod),
value="get_news",
),
IntInput(
name="num_news",
display_name="Number of News",
info="The number of news articles to retrieve (only applicable for get_news).",
value=5,
),
]
outputs = [
Output(display_name="Data", name="data", method="fetch_content"),
Output(display_name="Text", name="text", method="fetch_content_text"),
]
def run_model(self) -> list[Data]:
return self.fetch_content()
def fetch_content_text(self) -> Message:
data = self.fetch_content()
result_string = ""
for item in data:
result_string += item.text + "\n"
self.status = result_string
return Message(text=result_string)
def _fetch_yfinance_data(self, ticker: yf.Ticker, method: YahooFinanceMethod, num_news: int | None) -> str:
try:
if method == YahooFinanceMethod.GET_INFO:
result = ticker.info
elif method == YahooFinanceMethod.GET_NEWS:
result = ticker.news[:num_news]
else:
result = getattr(ticker, method.value)()
return pprint.pformat(result)
except Exception as e:
error_message = f"Error retrieving data: {e}"
logger.debug(error_message)
self.status = error_message
raise ToolException(error_message) from e
def fetch_content(self) -> list[Data]:
try:
return self._yahoo_finance_tool(
self.symbol,
YahooFinanceMethod(self.method),
self.num_news,
)
except ToolException:
raise
except Exception as e:
error_message = f"Unexpected error: {e}"
logger.debug(error_message)
self.status = error_message
raise ToolException(error_message) from e
def _yahoo_finance_tool(
self,
symbol: str,
method: YahooFinanceMethod,
num_news: int | None = 5,
) -> list[Data]:
ticker = yf.Ticker(symbol)
result = self._fetch_yfinance_data(ticker, method, num_news)
if method == YahooFinanceMethod.GET_NEWS:
data_list = [
Data(text=f"{article['title']}: {article['link']}", data=article)
for article in ast.literal_eval(result)
]
else:
data_list = [Data(text=result, data={"result": result})]
return data_list