Memory components in Langflow
This Langflow feature is currently in public preview. Development is ongoing, and the features and functionality are subject to change. Langflow, and the use of such, is subject to the DataStax Preview Terms. |
Memory components store and retrieve chat messages by session_id
.
They are distinct from vector store components, because they are built specifically for storing and retrieving chat messages from external databases.
Memory components provide access to their respective external databases as memory. This allows Large Language Models (LLMs) or Agents to access external memory for persistence and context retention.
Use a memory component in a flow
This example flow stores and retrieves chat history from an Astra DB Chat Memory component with Store Message and Chat Memory components.
The Store Message helper component stores chat memories as Data
objects, and the Message History helper component retrieves chat messages as Data
objects or strings.
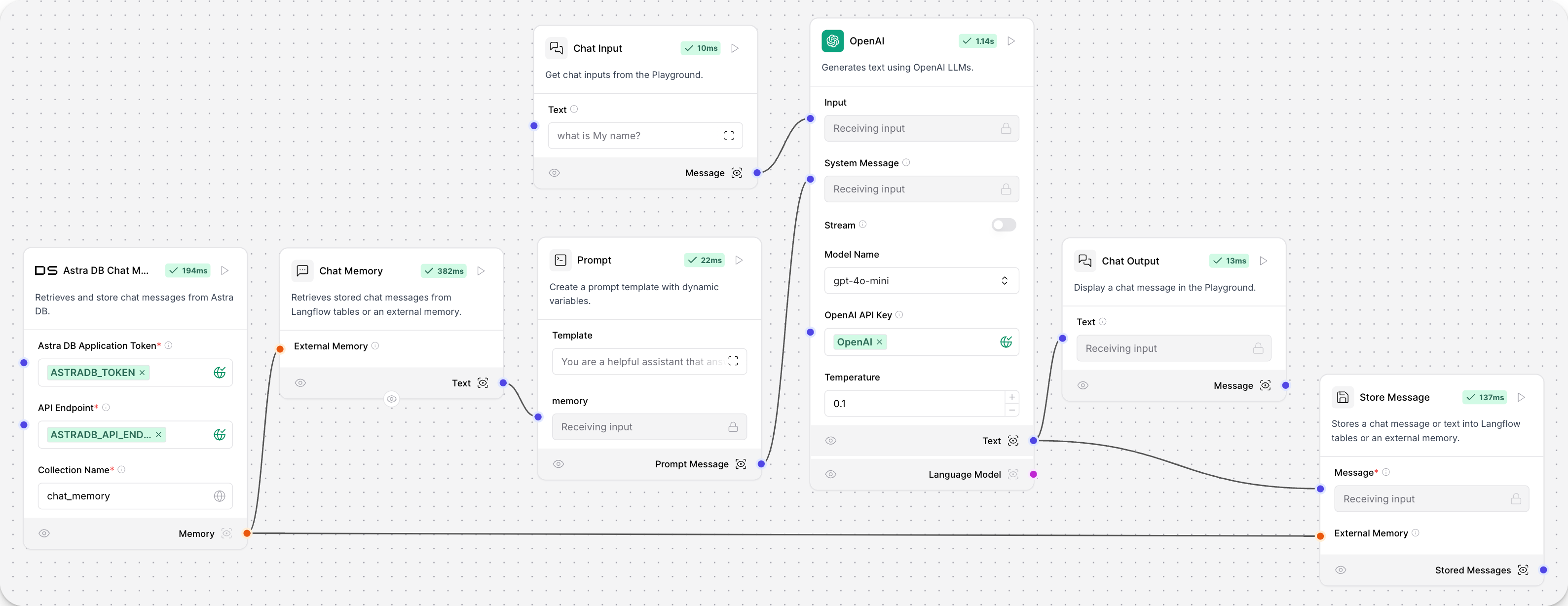
AstraDB Chat Memory Component
This component creates an AstraDBChatMessageHistory
instance, which allows for storing and retrieving chat messages using Astra DB.
Parameters
Name |
Type |
Description |
collection_name |
String |
Name of the Astra DB collection for storing messages. Required. |
token |
SecretString |
Authentication token for Astra DB access. Required. |
api_endpoint |
SecretString |
API endpoint URL for the Astra DB service. Required. |
namespace |
String |
Optional namespace within Astra DB for the collection. |
session_id |
MessageText |
Chat session ID. Uses current session ID if not provided. |
Name |
Type |
Description |
message_history |
BaseChatMessageHistory |
An instance of AstraDBChatMessageHistory for the session. |
Component code
astra_db.py
import os
from astrapy.admin import parse_api_endpoint
from langflow.base.memory.model import LCChatMemoryComponent
from langflow.field_typing.constants import Memory
from langflow.inputs import MessageTextInput, SecretStrInput, StrInput
class AstraDBChatMemory(LCChatMemoryComponent):
display_name = "Astra DB Chat Memory"
description = "Retrieves and store chat messages from Astra DB."
name = "AstraDBChatMemory"
icon: str = "AstraDB"
inputs = [
SecretStrInput(
name="token",
display_name="Astra DB Application Token",
info="Authentication token for accessing Astra DB.",
value="ASTRA_DB_APPLICATION_TOKEN",
required=True,
advanced=os.getenv("ASTRA_ENHANCED", "false").lower() == "true",
),
SecretStrInput(
name="api_endpoint",
display_name="API Endpoint",
info="API endpoint URL for the Astra DB service.",
value="ASTRA_DB_API_ENDPOINT",
required=True,
),
StrInput(
name="collection_name",
display_name="Collection Name",
info="The name of the collection within Astra DB where the vectors will be stored.",
required=True,
),
StrInput(
name="namespace",
display_name="Namespace",
info="Optional namespace within Astra DB to use for the collection.",
advanced=True,
),
MessageTextInput(
name="session_id",
display_name="Session ID",
info="The session ID of the chat. If empty, the current session ID parameter will be used.",
advanced=True,
),
]
def build_message_history(self) -> Memory:
try:
from langchain_astradb.chat_message_histories import AstraDBChatMessageHistory
except ImportError as e:
msg = (
"Could not import langchain Astra DB integration package. "
"Please install it with `pip install langchain-astradb`."
)
raise ImportError(msg) from e
return AstraDBChatMessageHistory(
session_id=self.session_id,
collection_name=self.collection_name,
token=self.token,
api_endpoint=self.api_endpoint,
namespace=self.namespace or None,
environment=parse_api_endpoint(self.api_endpoint).environment,
)
Cassandra Chat Memory Component
This component creates a CassandraChatMessageHistory
instance, enabling storage and retrieval of chat messages using Apache Cassandra or DataStax Astra DB.
Parameters
Name |
Type |
Description |
database_ref |
MessageText |
Contact points for Cassandra or Astra DB database ID. Required. |
username |
MessageText |
Username for Cassandra (leave empty for Astra DB). |
token |
SecretString |
Password for Cassandra or token for Astra DB. Required. |
keyspace |
MessageText |
Keyspace in Cassandra or namespace in Astra DB. Required. |
table_name |
MessageText |
Name of the table or collection for storing messages. Required. |
session_id |
MessageText |
Unique identifier for the chat session. Optional. |
cluster_kwargs |
Dictionary |
Additional keyword arguments for Cassandra cluster configuration. Optional. |
Name |
Type |
Description |
message_history |
BaseChatMessageHistory |
An instance of CassandraChatMessageHistory for the session. |
Component code
cassandra.py
from langflow.base.memory.model import LCChatMemoryComponent
from langflow.field_typing.constants import Memory
from langflow.inputs import DictInput, MessageTextInput, SecretStrInput
class CassandraChatMemory(LCChatMemoryComponent):
display_name = "Cassandra Chat Memory"
description = "Retrieves and store chat messages from Apache Cassandra."
name = "CassandraChatMemory"
icon = "Cassandra"
inputs = [
MessageTextInput(
name="database_ref",
display_name="Contact Points / Astra Database ID",
info="Contact points for the database (or AstraDB database ID)",
required=True,
),
MessageTextInput(
name="username", display_name="Username", info="Username for the database (leave empty for AstraDB)."
),
SecretStrInput(
name="token",
display_name="Password / AstraDB Token",
info="User password for the database (or AstraDB token).",
required=True,
),
MessageTextInput(
name="keyspace",
display_name="Keyspace",
info="Table Keyspace (or AstraDB namespace).",
required=True,
),
MessageTextInput(
name="table_name",
display_name="Table Name",
info="The name of the table (or AstraDB collection) where vectors will be stored.",
required=True,
),
MessageTextInput(
name="session_id", display_name="Session ID", info="Session ID for the message.", advanced=True
),
DictInput(
name="cluster_kwargs",
display_name="Cluster arguments",
info="Optional dictionary of additional keyword arguments for the Cassandra cluster.",
advanced=True,
is_list=True,
),
]
def build_message_history(self) -> Memory:
from langchain_community.chat_message_histories import CassandraChatMessageHistory
try:
import cassio
except ImportError as e:
msg = "Could not import cassio integration package. Please install it with `pip install cassio`."
raise ImportError(msg) from e
from uuid import UUID
database_ref = self.database_ref
try:
UUID(self.database_ref)
is_astra = True
except ValueError:
is_astra = False
if "," in self.database_ref:
# use a copy because we can't change the type of the parameter
database_ref = self.database_ref.split(",")
if is_astra:
cassio.init(
database_id=database_ref,
token=self.token,
cluster_kwargs=self.cluster_kwargs,
)
else:
cassio.init(
contact_points=database_ref,
username=self.username,
password=self.token,
cluster_kwargs=self.cluster_kwargs,
)
return CassandraChatMessageHistory(
session_id=self.session_id,
table_name=self.table_name,
keyspace=self.keyspace,
)
Mem0 Chat Memory
The Mem0 Chat Memory component retrieves and stores chat messages using Mem0 memory storage.
Parameters
Name | Display Name | Info |
---|---|---|
mem0_config |
Mem0 Configuration |
Configuration dictionary for initializing Mem0 memory instance. |
ingest_message |
Message to Ingest |
The message content to be ingested into Mem0 memory. |
existing_memory |
Existing Memory Instance |
Optional existing Mem0 memory instance. |
user_id |
User ID |
Identifier for the user associated with the messages. |
search_query |
Search Query |
Input text for searching related memories in Mem0. |
mem0_api_key |
Mem0 API Key |
API key for Mem0 platform (leave empty to use the local version). |
metadata |
Metadata |
Additional metadata to associate with the ingested message. |
openai_api_key |
OpenAI API Key |
API key for OpenAI (required if using OpenAI embeddings without a provided configuration). |
Name | Display Name | Info |
---|---|---|
memory |
Mem0 Memory |
The resulting Mem0 Memory object after ingesting data. |
search_results |
Search Results |
The search results from querying Mem0 memory. |
Component code
mem0_chat_memory.py
import os
from loguru import logger
from mem0 import Memory, MemoryClient
from langflow.base.memory.model import LCChatMemoryComponent
from langflow.inputs import (
DictInput,
HandleInput,
MessageTextInput,
NestedDictInput,
SecretStrInput,
)
from langflow.io import Output
from langflow.schema import Data
class Mem0MemoryComponent(LCChatMemoryComponent):
display_name = "Mem0 Chat Memory"
description = "Retrieves and stores chat messages using Mem0 memory storage."
name = "mem0_chat_memory"
icon: str = "Mem0"
inputs = [
NestedDictInput(
name="mem0_config",
display_name="Mem0 Configuration",
info="""Configuration dictionary for initializing Mem0 memory instance.
Example:
{
"graph_store": {
"provider": "neo4j",
"config": {
"url": "neo4j+s://your-neo4j-url",
"username": "neo4j",
"password": "your-password"
}
},
"version": "v1.1"
}""",
input_types=["Data"],
),
MessageTextInput(
name="ingest_message",
display_name="Message to Ingest",
info="The message content to be ingested into Mem0 memory.",
),
HandleInput(
name="existing_memory",
display_name="Existing Memory Instance",
input_types=["Memory"],
info="Optional existing Mem0 memory instance. If not provided, a new instance will be created.",
),
MessageTextInput(
name="user_id", display_name="User ID", info="Identifier for the user associated with the messages."
),
MessageTextInput(
name="search_query", display_name="Search Query", info="Input text for searching related memories in Mem0."
),
SecretStrInput(
name="mem0_api_key",
display_name="Mem0 API Key",
info="API key for Mem0 platform. Leave empty to use the local version.",
),
DictInput(
name="metadata",
display_name="Metadata",
info="Additional metadata to associate with the ingested message.",
advanced=True,
),
SecretStrInput(
name="openai_api_key",
display_name="OpenAI API Key",
required=False,
info="API key for OpenAI. Required if using OpenAI Embeddings without a provided configuration.",
),
]
outputs = [
Output(name="memory", display_name="Mem0 Memory", method="ingest_data"),
Output(
name="search_results",
display_name="Search Results",
method="build_search_results",
),
]
def build_mem0(self) -> Memory:
"""Initializes a Mem0 memory instance based on provided configuration and API keys."""
if self.openai_api_key:
os.environ["OPENAI_API_KEY"] = self.openai_api_key
try:
if not self.mem0_api_key:
return Memory.from_config(config_dict=dict(self.mem0_config)) if self.mem0_config else Memory()
if self.mem0_config:
return MemoryClient.from_config(api_key=self.mem0_api_key, config_dict=dict(self.mem0_config))
return MemoryClient(api_key=self.mem0_api_key)
except ImportError as e:
msg = "Mem0 is not properly installed. Please install it with 'pip install -U mem0ai'."
raise ImportError(msg) from e
def ingest_data(self) -> Memory:
"""Ingests a new message into Mem0 memory and returns the updated memory instance."""
mem0_memory = self.existing_memory or self.build_mem0()
if not self.ingest_message or not self.user_id:
logger.warning("Missing 'ingest_message' or 'user_id'; cannot ingest data.")
return mem0_memory
metadata = self.metadata or {}
logger.info("Ingesting message for user_id: %s", self.user_id)
try:
mem0_memory.add(self.ingest_message, user_id=self.user_id, metadata=metadata)
except Exception:
logger.exception("Failed to add message to Mem0 memory.")
raise
return mem0_memory
def build_search_results(self) -> Data:
"""Searches the Mem0 memory for related messages based on the search query and returns the results."""
mem0_memory = self.ingest_data()
search_query = self.search_query
user_id = self.user_id
logger.info("Search query: %s", search_query)
try:
if search_query:
logger.info("Performing search with query.")
related_memories = mem0_memory.search(query=search_query, user_id=user_id)
else:
logger.info("Retrieving all memories for user_id: %s", user_id)
related_memories = mem0_memory.get_all(user_id=user_id)
except Exception:
logger.exception("Failed to retrieve related memories from Mem0.")
raise
logger.info("Related memories retrieved: %s", related_memories)
return related_memories
Redis Chat Memory
The Redis Chat Memory component retrieves and stores chat messages using Redis.
Parameters
Name | Display Name | Info |
---|---|---|
host |
hostname |
The IP address or hostname of the Redis server. This is required to establish a connection to the Redis instance. |
port |
port |
The port number on which the Redis server is listening. The default Redis port is 6379. |
database |
database |
The Redis database number to use. Redis supports multiple databases, identified by integers. The default is usually 0. |
username |
Username |
The username for authenticating with the Redis server. This is used if Redis is configured with access control lists (ACLs). |
password |
Password |
The password associated with the username for Redis authentication. This should be kept secure and not exposed in plain text. |
key_prefix |
Key prefix |
A string prefix added to all keys stored in Redis for this chat memory. This helps in organizing and isolating keys for different applications or purposes within the same Redis instance. |
session_id |
Session ID |
A unique identifier for the chat session. This is used to retrieve and store messages specific to a particular conversation or user session. |
Name | Display Name | Info |
---|---|---|
message_history |
Message History |
A |
Component code
redis.py
from urllib import parse
from langchain_community.chat_message_histories.redis import RedisChatMessageHistory
from langflow.base.memory.model import LCChatMemoryComponent
from langflow.field_typing.constants import Memory
from langflow.inputs import IntInput, MessageTextInput, SecretStrInput, StrInput
class RedisIndexChatMemory(LCChatMemoryComponent):
display_name = "Redis Chat Memory"
description = "Retrieves and store chat messages from Redis."
name = "RedisChatMemory"
icon = "Redis"
inputs = [
StrInput(
name="host", display_name="hostname", required=True, value="localhost", info="IP address or hostname."
),
IntInput(name="port", display_name="port", required=True, value=6379, info="Redis Port Number."),
StrInput(name="database", display_name="database", required=True, value="0", info="Redis database."),
MessageTextInput(
name="username", display_name="Username", value="", info="The Redis user name.", advanced=True
),
SecretStrInput(
name="password", display_name="Password", value="", info="The password for username.", advanced=True
),
StrInput(name="key_prefix", display_name="Key prefix", info="Key prefix.", advanced=True),
MessageTextInput(
name="session_id", display_name="Session ID", info="Session ID for the message.", advanced=True
),
]
def build_message_history(self) -> Memory:
kwargs = {}
password: str | None = self.password
if self.key_prefix:
kwargs["key_prefix"] = self.key_prefix
if password:
password = parse.quote_plus(password)
url = f"redis://{self.username}:{self.password}@{self.host}:{self.port}/{self.database}"
return RedisChatMessageHistory(session_id=self.session_id, url=url, **kwargs)
Zep Chat Memory Component
This component is in Legacy. Legacy components can be used in flows, but may not work due to Langflow core updates. Instead, use the AstraDB Chat Memory component. |
This component creates a ZepChatMessageHistory instance, enabling storage and retrieval of chat messages using Zep, a memory server for Large Language Models (LLMs).
Parameters
Name |
Type |
Description |
url |
MessageText |
URL of the Zep instance. Required. |
api_key |
SecretString |
API Key for authentication with the Zep instance. |
api_base_path |
Dropdown |
API version to use. Use |
session_id |
MessageText |
Unique identifier for the chat session. Optional. |
Name |
Type |
Description |
message_history |
BaseChatMessageHistory |
An instance of ZepChatMessageHistory for the session. |
Component code
zep.py
from langflow.base.memory.model import LCChatMemoryComponent
from langflow.field_typing.constants import Memory
from langflow.inputs import DropdownInput, MessageTextInput, SecretStrInput
class ZepChatMemory(LCChatMemoryComponent):
display_name = "Zep Chat Memory"
description = "Retrieves and store chat messages from Zep."
name = "ZepChatMemory"
icon = "ZepMemory"
legacy = True
inputs = [
MessageTextInput(name="url", display_name="Zep URL", info="URL of the Zep instance."),
SecretStrInput(name="api_key", display_name="API Key", info="API Key for the Zep instance."),
DropdownInput(
name="api_base_path",
display_name="API Base Path",
options=["api/v1", "api/v2"],
value="api/v1",
advanced=True,
),
MessageTextInput(
name="session_id", display_name="Session ID", info="Session ID for the message.", advanced=True
),
]
def build_message_history(self) -> Memory:
try:
# Monkeypatch API_BASE_PATH to
# avoid 404
# This is a workaround for the local Zep instance
# cloud Zep works with v2
import zep_python.zep_client
from zep_python import ZepClient
from zep_python.langchain import ZepChatMessageHistory
zep_python.zep_client.API_BASE_PATH = self.api_base_path
except ImportError as e:
msg = "Could not import zep-python package. Please install it with `pip install zep-python`."
raise ImportError(msg) from e
zep_client = ZepClient(api_url=self.url, api_key=self.api_key)
return ZepChatMessageHistory(session_id=self.session_id, zep_client=zep_client)